Sir-Dl: An architecture of semantic-based image retrieval using deep learning technique and rdf triple language
The problem of finding and identifying semantics of images is applied in multimedia applications of many different fields such as hospital information system, geographic information system,
digital library system, etc. In this paper, we propose the Semantic-Based Image Retrieval (SBIR)
system based on the deep learning technique; this system is called as SIR-DL that generates visual
semantics based on classifying image contents. Firstly, the color and spatial features of segmented
images are extracted and these visual feature vectors are trained on the deep neural network to obtain
visual words vectors. Then, we retrieve it on ontology to provide the identities and the semantics
of similar images corresponds to a similarity measure. In order to carry out SIR-DL, the algorithms
and diagram of this image retrieval system are proposed and after that we implement them on ImageCLEF@IAPR, which has 20,000 images. Based on experimental results, the effectiveness of our
method is evaluated; these results are compared with some of the works recently published on the
same image dataset. It shows that SIR-DL effectively solves the problem of SBIR and can be used
to build multimedia systems in many different fields.
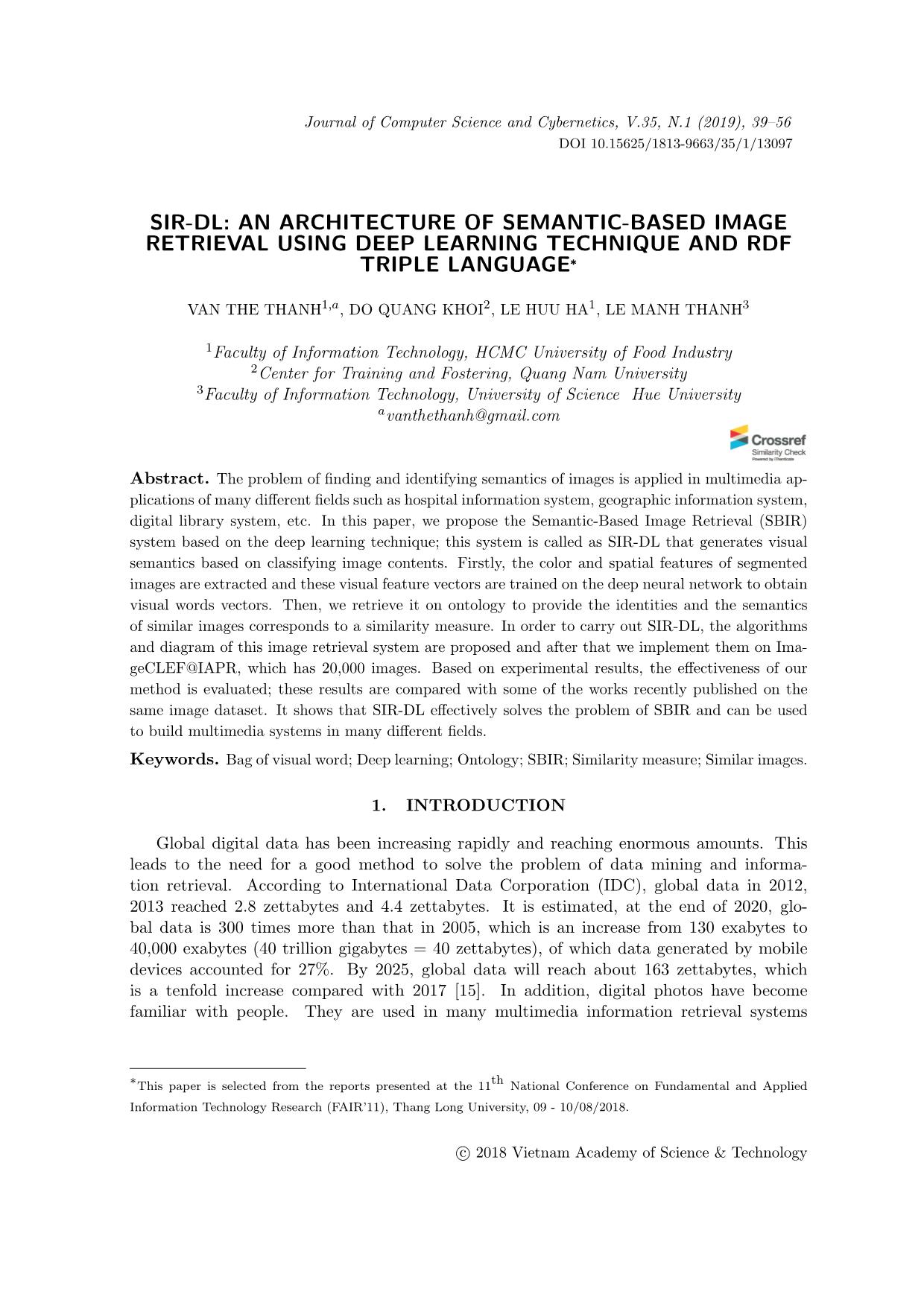
Trang 1
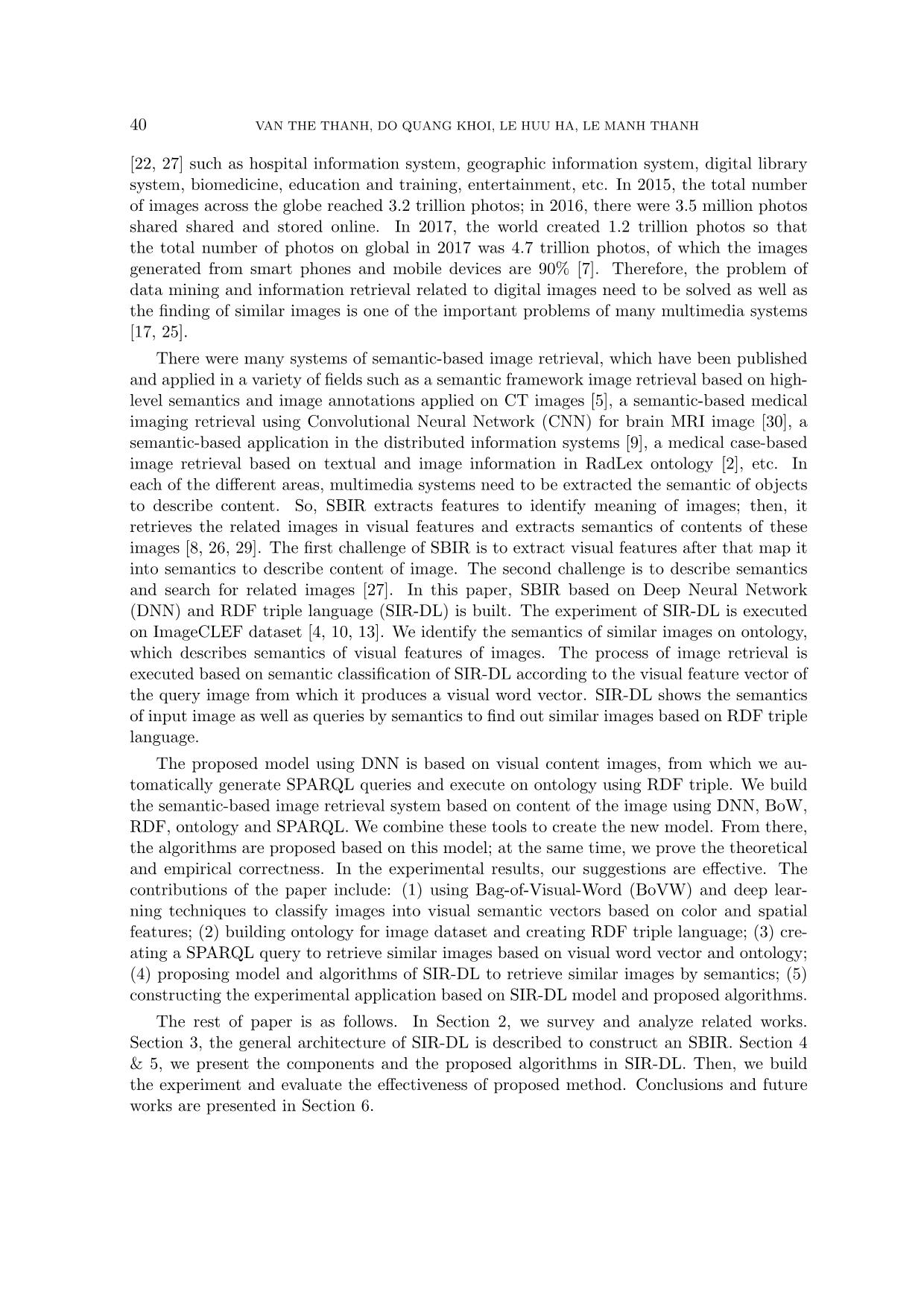
Trang 2
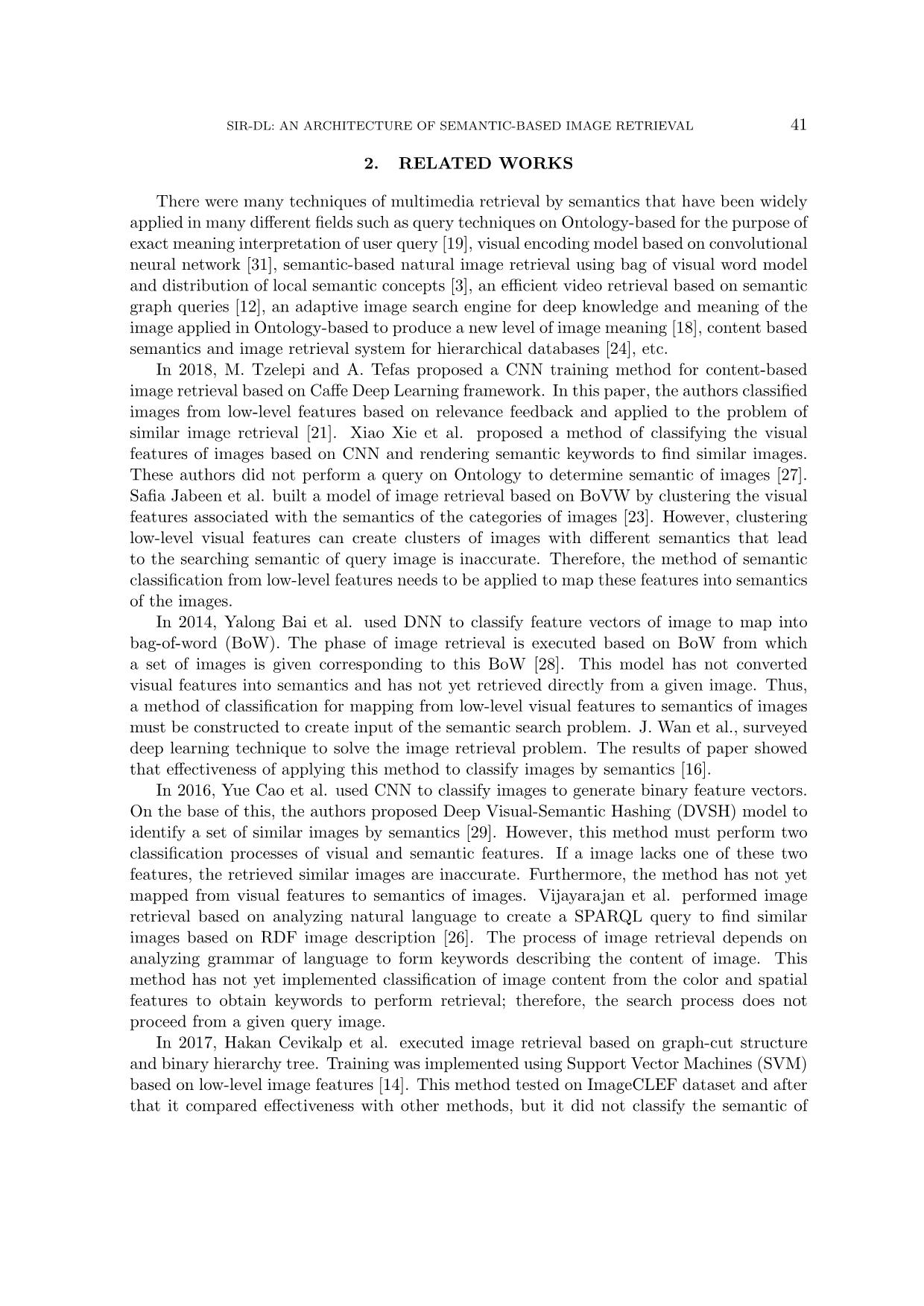
Trang 3
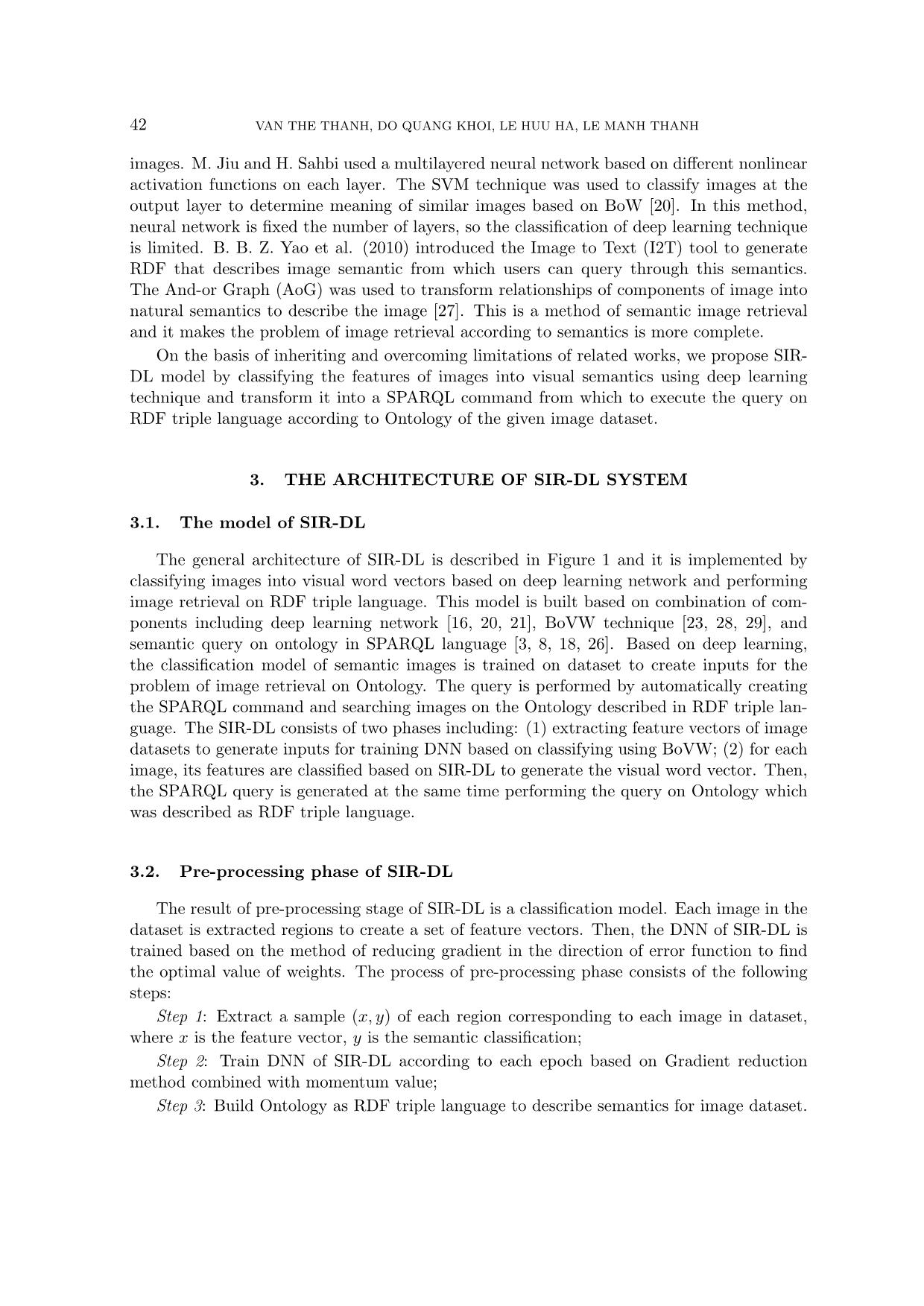
Trang 4
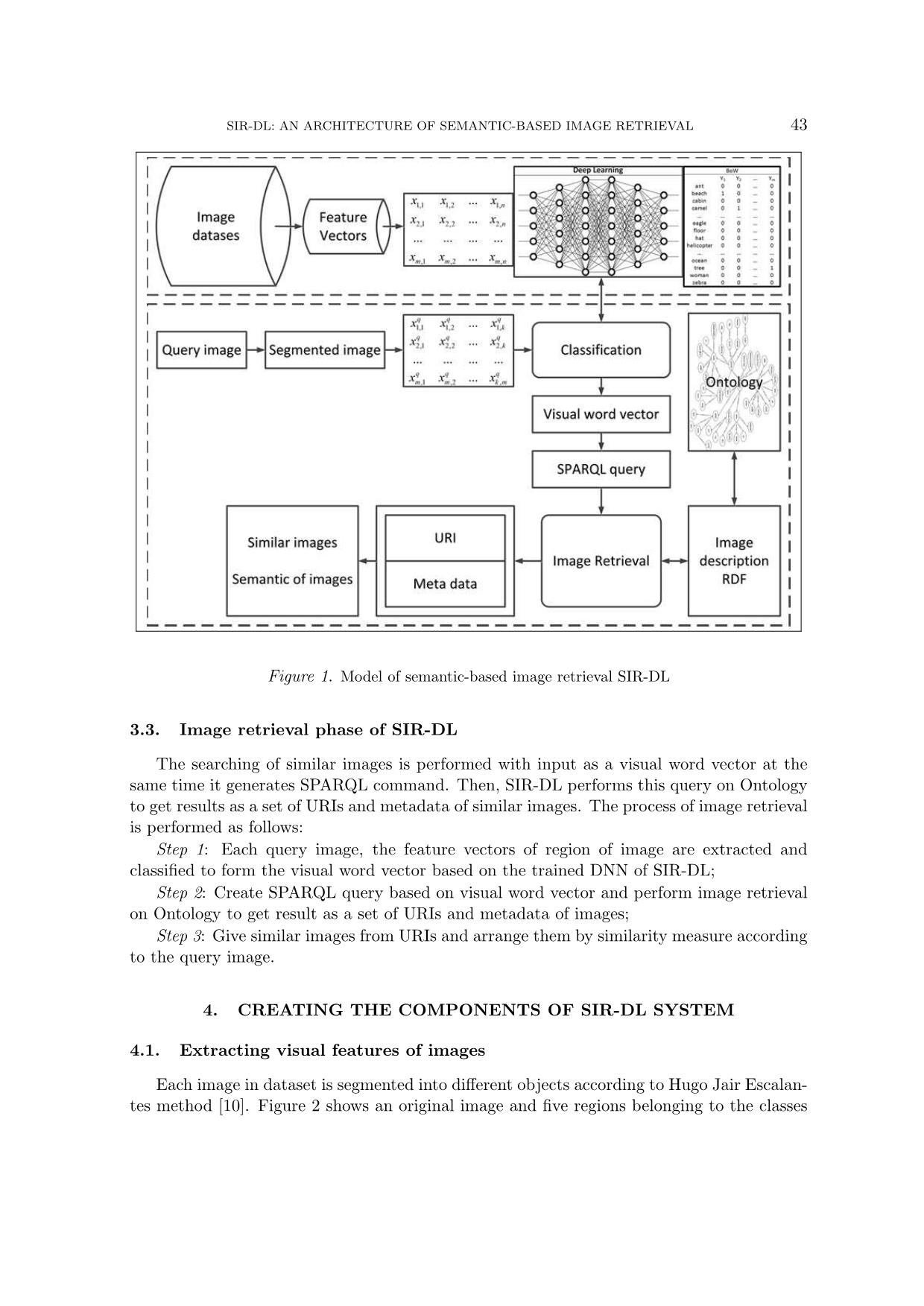
Trang 5
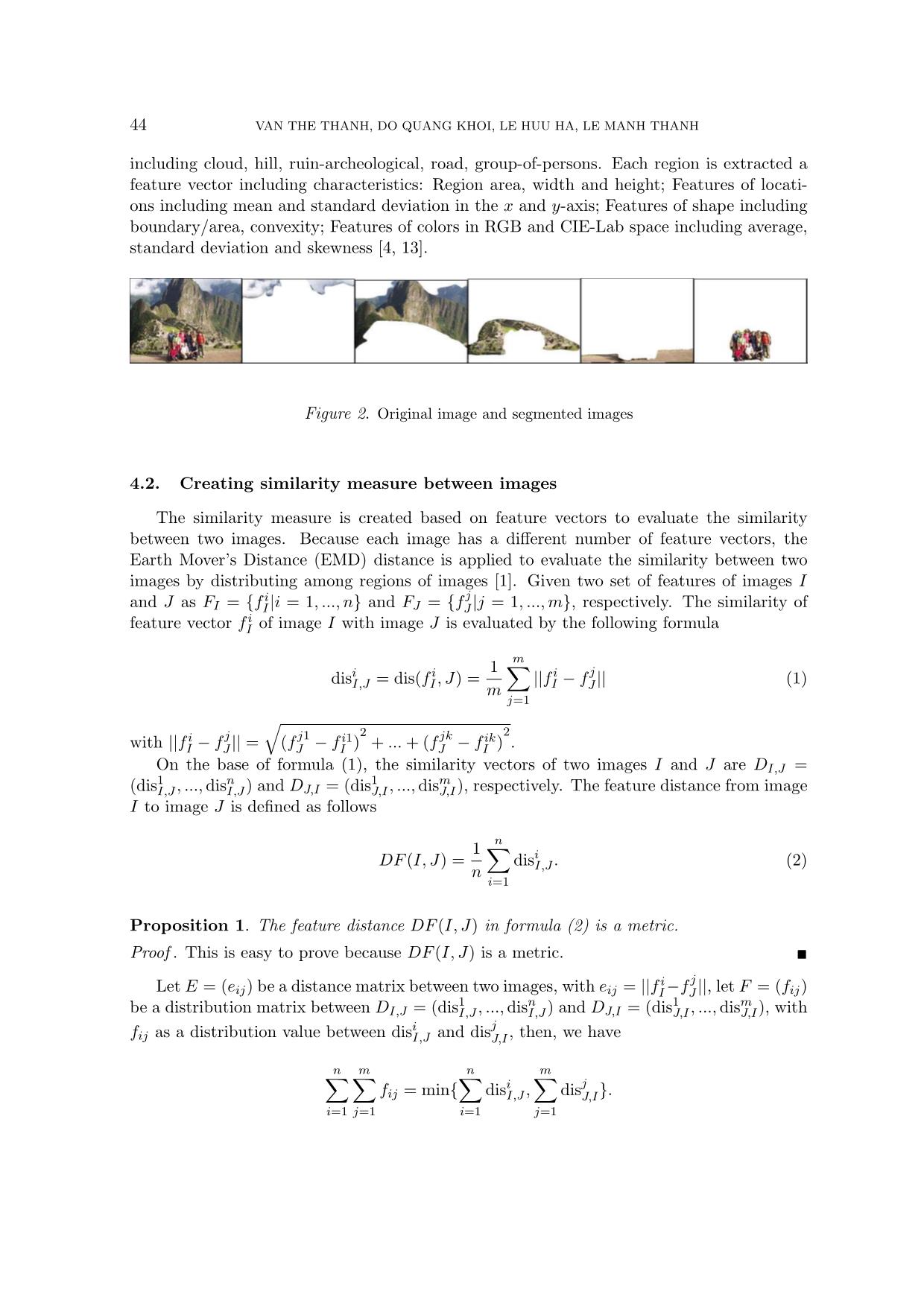
Trang 6
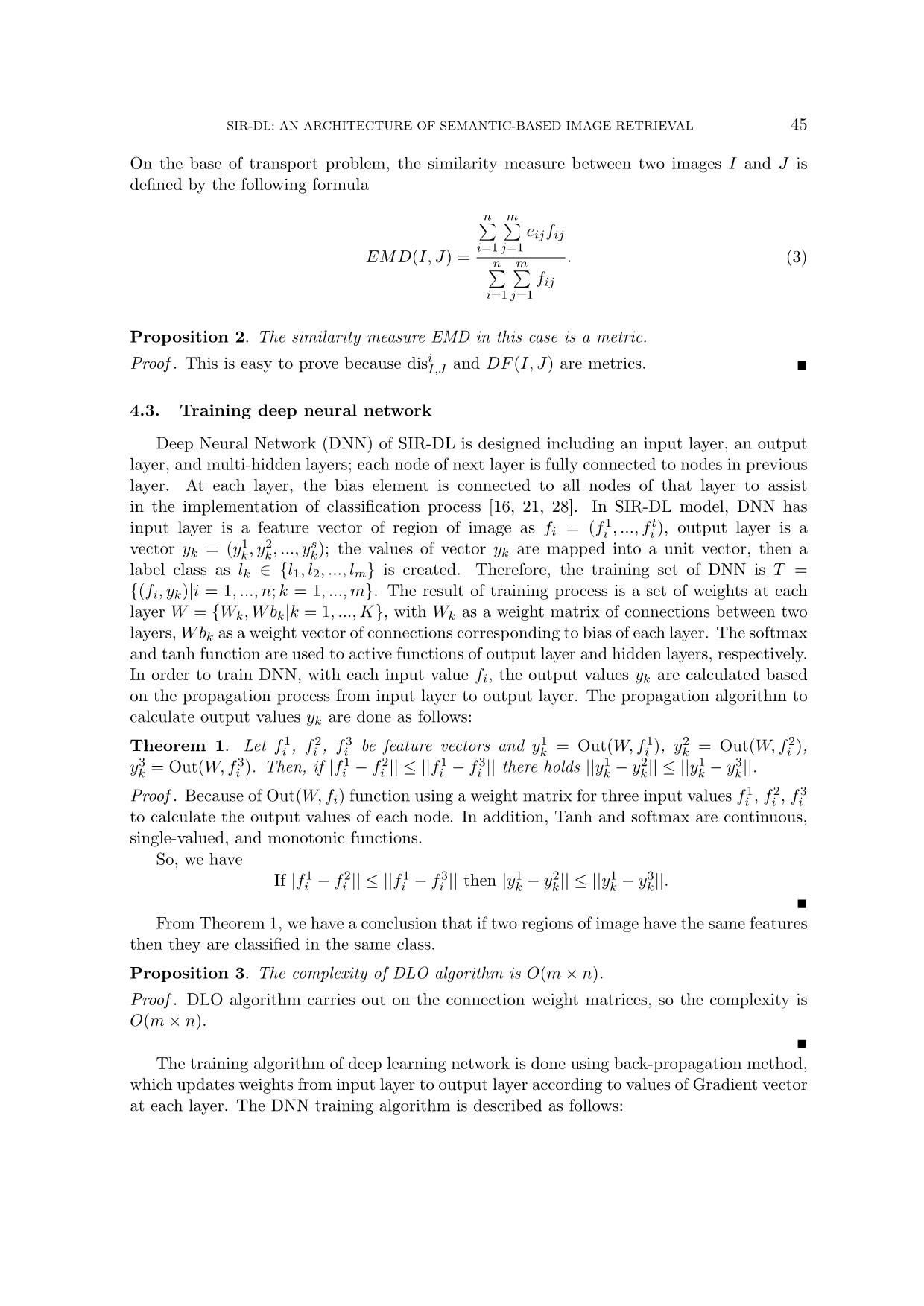
Trang 7
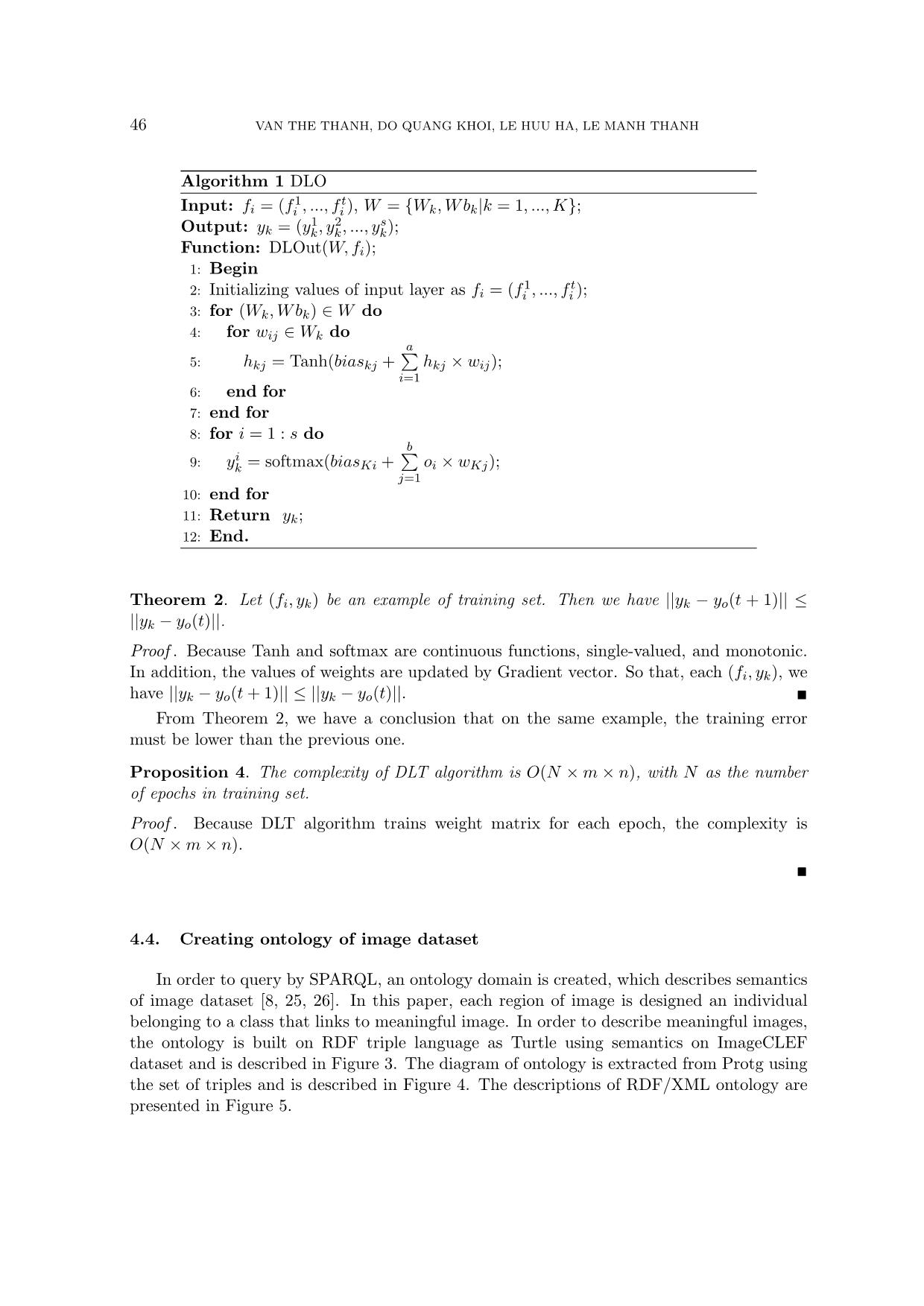
Trang 8
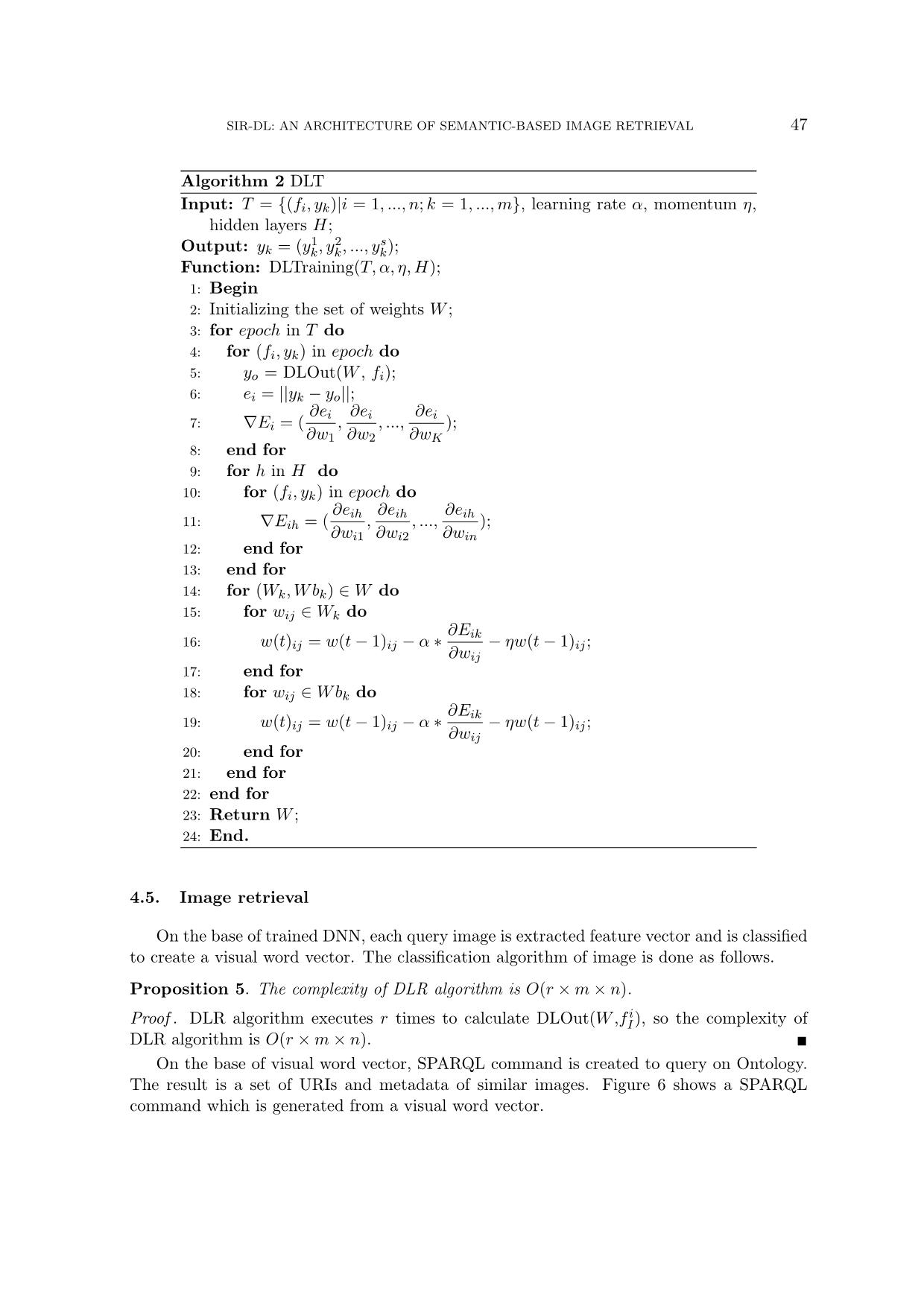
Trang 9
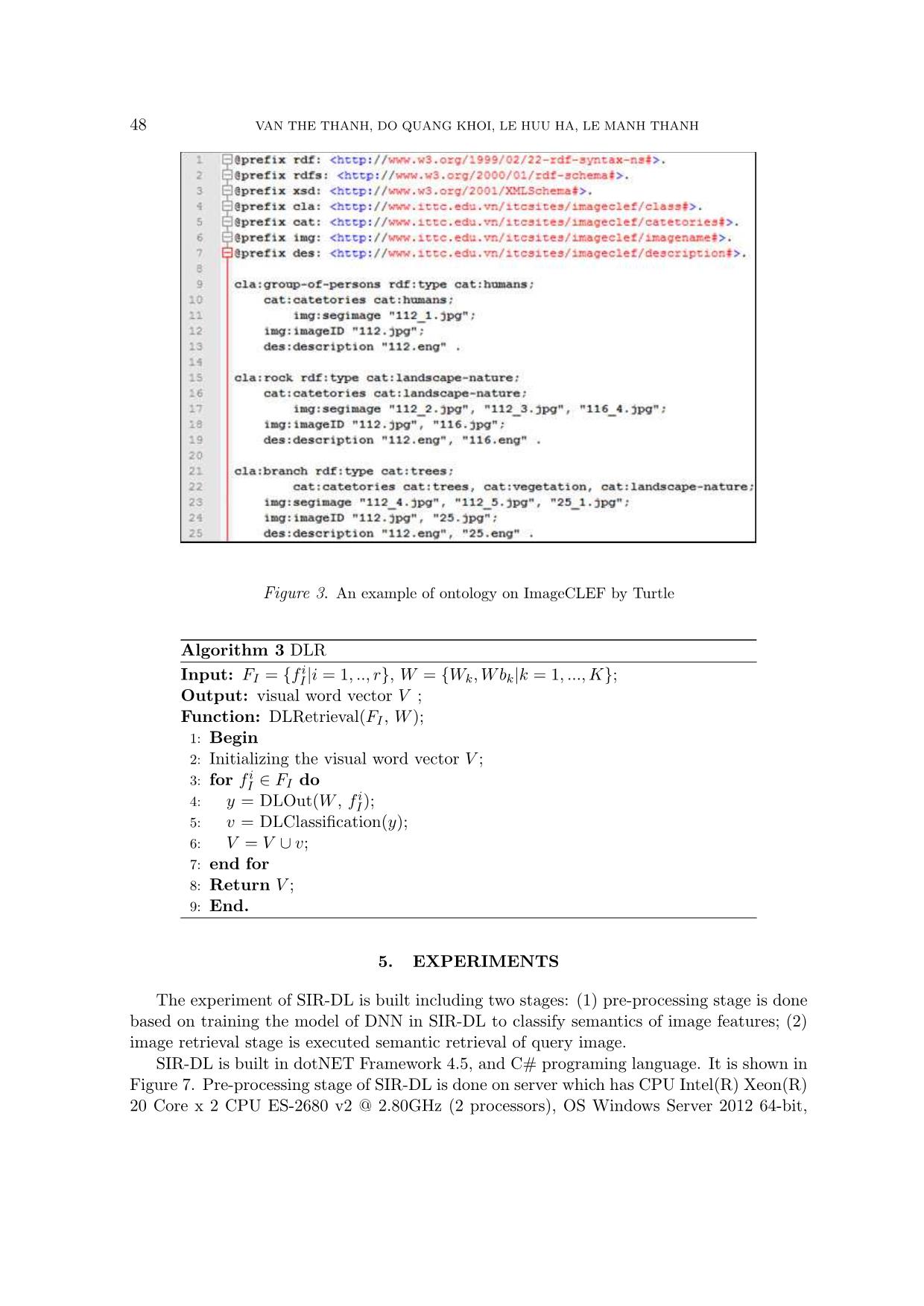
Trang 10
Tải về để xem bản đầy đủ
Tóm tắt nội dung tài liệu: Sir-Dl: An architecture of semantic-based image retrieval using deep learning technique and rdf triple language
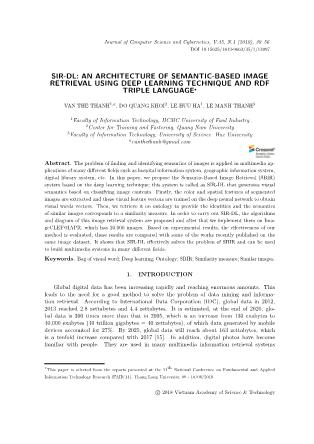
feature vector and is classified to create a visual word vector. The classification algorithm of image is done as follows. Proposition 5. The complexity of DLR algorithm is O(r ×m× n). Proof . DLR algorithm executes r times to calculate DLOut(W ,f iI), so the complexity of DLR algorithm is O(r ×m× n). On the base of visual word vector, SPARQL command is created to query on Ontology. The result is a set of URIs and metadata of similar images. Figure 6 shows a SPARQL command which is generated from a visual word vector. 48 VAN THE THANH, DO QUANG KHOI, LE HUU HA, LE MANH THANH Figure 3. An example of ontology on ImageCLEF by Turtle Algorithm 3 DLR Input: FI = {f iI |i = 1, .., r}, W = {Wk,Wbk|k = 1, ...,K}; Output: visual word vector V ; Function: DLRetrieval(FI , W ); 1: Begin 2: Initializing the visual word vector V ; 3: for f iI ∈ FI do 4: y = DLOut(W , f iI); 5: v = DLClassification(y); 6: V = V ∪ v; 7: end for 8: Return V ; 9: End. 5. EXPERIMENTS The experiment of SIR-DL is built including two stages: (1) pre-processing stage is done based on training the model of DNN in SIR-DL to classify semantics of image features; (2) image retrieval stage is executed semantic retrieval of query image. SIR-DL is built in dotNET Framework 4.5, and C# programing language. It is shown in Figure 7. Pre-processing stage of SIR-DL is done on server which has CPU Intel(R) Xeon(R) 20 Core x 2 CPU ES-2680 v2 @ 2.80GHz (2 processors), OS Windows Server 2012 64-bit, SIR-DL: AN ARCHITECTURE OF SEMANTIC-BASED IMAGE RETRIEVAL 49 Figure 4. Ontology of ImageCLEF dataset on Protege Algorithm 4 DLC Input: vector y; Output: an unit vetor v; Function: DLClassification(y); 1: Begin 2: v = (v1, v2, ..., vn), so that ci = 0; 3: k = argMax(yi); 4: vk = 1; 5: Return v; 6: End. RAM 128 GB. Image retrieval stage is carried out on computer, which has CPU Intel(R) CoreTM i7-2620M, CPU 2,70GHz, RAM 4GB, and OS Windows 7 Professional. The results of experiment are evaluated on ImageCLEF dataset, which has 20,000 images including 276 classes and stores in 41 folders (from 0-th folder to 40-th folder); the volume size of this dataset is 1.64 GB. In order to assess effectiveness of proposed method, the experiment is shown values including precision, recall, and F-measure. These values are described by the recall-precision and ROC curves. The formulas of these values are as follows [1] precision = |relevant images ∩ retrieved images| |retrieved images| , (4) recall = |relevantimages ∩ retrievedimages| |relevantimages| , (5) F-measure = 2× (precision× recall) (precision + recall) . (6) Our empirical data set is divided into two sections, one for training data and one for test data. Number of photos is taken randomly. The results of experiment of SIR-DL are shown in Figure 8, Figure 9, Figure 10, and Figure 11. Performance of SIR-DL is given 50 VAN THE THANH, DO QUANG KHOI, LE HUU HA, LE MANH THANH Figure 5. An example of ontology on ImageCLEF dataset by RDF/XML Figure 6. A SPARQL command SIR-DL: AN ARCHITECTURE OF SEMANTIC-BASED IMAGE RETRIEVAL 51 Figure 7. The application of SIR-DL for semantic-based image retrieval Table 1. Performance of image retrieval of proposed method on ImageCLEF dataset ID No. images Ave. recall Ave. precision Ave. F-measure Ave. query time (ms) 00-10 2460 0.401259 0.609260 0.431001 875.1342 11-20 1797 0.410326 0.589953 0.430598 829.8472 21-30 1239 0.418620 0.607360 0.440907 828.1287 31-40 1431 0.437902 0.640513 0.470151 674.1342 in Table 1, which has 6927 query images; the averages of performance are 0.4123; 0.6054; 0.4381; 834.1439. Accuracies and errors in the process training of deep neural network are shown in Figure 9. The values of accuracy increase and errors decrease show that DLT training algorithm is exact in experiment. Figure 10 shows the curves of Precision-Recall and ROC, each curve describes a set of query images, which are retrieved. The areas under these curves show that the accuracy of image retrieval is not high; however, it has many curves above the average line. Figure 11 shows the average of precision, recall, and F-measure of 39 subjects on Ima- geCLEF dataset. The values of Mean Average Precision (MAP) of proposed method are compared with other methods on the same dataset. They are described in Table 2, which shows that the accuracy of SIR-DL is higher than that of other methods. In Y.Cao’s work [29], the author performs image retrieval rely on CNN using AlexNet. In this method, two vectors are created including the image vector and the sentence vector. Then the authors search similar images but it does not create semantic of image content as well as does not query on Ontology. In this way, the authors only find similar images and can not find the semantic of each image, so this method only performs the first stage of the semantic image retrieval. So that, the accurate of this method more than the one of proposed 52 VAN THE THANH, DO QUANG KHOI, LE HUU HA, LE MANH THANH Figure 8. The result of semantic image retrieval using SIR-DL architecture Figure 9. The accuracies and errors training of DNN in SIR-DL method of this paper. We compared this work to show the difference between two problems, including the image retrieval based on semantic and the semantic-based image retrieval. In the proposed method, we extracted semantics of image from content based on DNN and query on Ontology. Therefore, each query image, we generate semantics from image content and then automatic create a query based on SPARQL language. This shows that we can interpret the content of each image and easily apply in multimedia systems such as Hospital Information System, Geographic Information System, Digital Library System, etc. In addition, if our proposed method compared with the last four years, our results are more effective than the results of the other works. This shows that the effectiveness of our work. SIR-DL: AN ARCHITECTURE OF SEMANTIC-BASED IMAGE RETRIEVAL 53 Figure 10. The Precision-Recall and ROC curves of SIR-DL on ImageCLEF dataset Figure 11. The average of Precision, Recall, F-measure of SIR-DL on ImageCLEF dataset 6. CONCLUSIONS AND FUTURE WORKS In this paper, the model of SIR-DL was built to retrieve similar images based on seman- tics. The process of image retrieval is done by semantic classification using image content from which create a visual word vector to generate a SPARQL query. The results of image retrieval were accessed from the Ontology, which describes image meaning of ImageCLEF dataset. On the base of SIR-DL model, the algorithms were proposed and after that they were assessed performance based on the values of recall, precision, F-measure, and query 54 VAN THE THANH, DO QUANG KHOI, LE HUU HA, LE MANH THANH Table 2. Comparison mean average precision (MAP) of methods on ImageCLEF dataset Methods MAP M. Jiu, 2017 [20] 0.5970 H. Cevikalp 2017 [14] 0.4678 Y. Cao, 2016 [29] 0.7236 V. Vijayarajan, 2016 [26] 0.4618 S. Fakhfakh, 2015 [11] 0.5400 C.A. Hernndez-Gracidas, 2013 [6] 0.5826 our proposed method (SIR-DL) 0.6054 time (milli-seconds). The experimental results of SIR-DL were compared with the result of the other methods on the same dataset from which show that the proposed method is relatively effective. The experiments have shown the correctness of the proposed model and algorithm, so SIR-DL can be improved for semantic image retrieval systems. The future works of SIR-DL are creating the process of online extraction. Then, the training and fin- ding of images can be extracted for online data from WWW based on URIs from which creates image retrieval systems such as HIS, GIS, etc. ACKNOWLEDGMENT The authors wish to thank the Faculty of Information Technology, HCMC University of Food Industry, the Faculty of Information Technology, University of Sciences/Hue University, Vietnam, and the Center for Training and Fostering, Quang Nam University, Vietnam. We would also like to thank the anonymous reviewers for their helpful comments and valuable suggestions. REFERENCES [1] N. R. A. Alzubi, A. Amira, “Semantic content-based image retrieval: A comprehensive study,” Journal of Visual Communication and Image Representation, vol. 32, pp. 20–54, 2017. [2] L. J. A.B. Spanier, D. Cohen, “A new method for the automatic retrieval of medical cases based on the radlex ontology,” International Journal of Computer Assisted Radiology and Surgery, vol. 12, no. 3, pp. 471–484, 2017. [3] Y. Alqasrawi, “Bridging the gap between local semantic concepts and bag of visual words for natural scene image retrieval,” International Journal of Sensors Wireless Communications and Control, vol. 6, no. 3, pp. 174–191, 2016. [4] L. S. C. Hernndez-Gracidas, “Markov random fields and spatial information to improve automatic image annotation,” in Advances in Image and Video Technology. PSIVT 2007. Lecture Notes in Computer Science. Springer, Berlin, Heidelberg, 2007, pp. 879–892. [Online]. Available: https://link.springer.com/chapter/10.1007/978-3-540-77129-6 74 [5] C. B. C. Kurtz, A. Depeursinge, “A semantic framework for the retrieval of similar radiological images based on medical annotations,” in IEEE International Conference SIR-DL: AN ARCHITECTURE OF SEMANTIC-BASED IMAGE RETRIEVAL 55 on Image Processing, TCIP. Paris, France: IEEE, 2004. [Online]. Available: https: //ieeexplore.ieee.org/document/7025454/ [6] M. M.-y.-G. C.A. Hernndez-Gracidas, L.E. Sucar, “Improving image retrieval by using spatial relations,” Multimedia Tools and Applications, vol. 62, no. 2, pp. 479–505, 2013. [7] Deloitte, “Photo sharing: trillions and rising,” Deloitte Touche Tohmatsu Limited, Deloitte Global, Tech. Rep., 2016. [8] B. Z. Y. et al., “I2t: Image parsing to text description,” in Proceedings of the IEEE, vol. 98, no. 8. IEEE, 2010, pp. 1485–1508. [Online]. Available: https://ieeexplore.ieee.org/document/5487377/ [9] C. B. et al., Emerging Semantic-Based Applications. Springer, 2016. [10] H. E. et al., “The segmented and annotated iapr tc-12 benchmark,” Computer Vision and Image Understanding, vol. 114, no. 4, pp. 419–428, 2010. [11] S. F. et al., “Image retrieval based on using hamming distance,” Procedia Comp. Sci., vol. 73, pp. 320–327, 2015. [12] Z. Z. V. S. G. Castanon, Y. Chen, “Efficient activity retrieval through semantic graph queries,” in International conference on Multimedia. Brisbane, Australia: ACM, 2015, pp. 391–400. [Online]. Available: https://dl.acm.org/citation.cfm?id=2806229 [13] M. Grubinger, “Analysis and evaluation of visual information systems performance,” School of Computer Science and Mathematics, Faculty of Health, Engineering and Science, Victoria University, Melbourne, Australia, Tech. Rep., 2007. [14] S. O. H. Cevikalp, M. Elmas, “Large-scale image retrieval using transductive support vector machines,” Computer Vision and Image Understanding, vol. 173, pp. 2–12, 2018. [15] D. R. J. Gantz, “The digital universe in 2020: Big data, bigger digi tal shadows, and biggest growth in the far east.” IDC iView, EMC Corporation, Tech. Rep., 2012 and 2014. [16] S. H. P. W.-J. Z. Y. Z. J. L. J. Wan, D. Wang, “Deep learning for content-based image retrieval: A comprehensive study,” in Proceedings of the 22nd ACM International conference on Multimedia. Orlando, Florida, USA: ACM, 2014, pp. 157–166. [Online]. Available: https://dl.acm.org/citation.cfm?id=2654948 [17] H. A. L. Deligiannidis, Emerging Trends in Image Processing: Computer Vision, and Pattern Recognition, ser. Graduate Texts in Mathematics. Elsevier, USA: Morgan Kaufmann, Waltham, MA 02451, 2015. [18] Y. Li, “Semantic image similarity based on deep knowledge for effective image retrieval,” De- partment of Computer Science., Hong Kong Baptist University, Tech. Rep., 2014. [19] E.-I. C. M.-H. Lee, S. Rho, “Ontology based user query interpretation for semantic multimedia contents retrieval,” Multimedia Tools and Applications, vol. 73, no. 2, pp. 901–915, 2014. [20] H. S. M. Jiu, “Nonlinear deep kernel learning for image annotation,” IEEE Trans. on Image Processing, vol. 26, no. 4, pp. 1820–1832, 2017. [21] A. T. M. Tzelepi, “Deep convolutional learning for content based image retrieval,” Neurocom- puting, vol. 275, pp. 2467–2478, 2018. [22] L. G. P. Muneesawang, N. Zhang, Multimedia Database Retrieval: Technology and Applications, ser. Graduate Texts in Mathematics. Springer, New York Dordrecht London, 2014. 56 VAN THE THANH, DO QUANG KHOI, LE HUU HA, LE MANH THANH [23] T. M.-T. S.-A. R. M. M. S. Jabeen, Z. Mehmood, “An effective content-based image retrieval technique for image visuals representation based on the bag-of-visual-wordsmodel,” PLoS ONE, vol. 13, no. 4, pp. 1–24, 2018. [24] H. S. Pandey, PriteeKhanna, “A semantics and image retrieval system for hierarchical image databases,” Information Processing & Management, vol. 52, no. 4, pp. 571–591, 2016. [25] S. M. Sohail Sarwar, Zia Ul Qayyum, “Ontology based image retrieval framework using quali- tative semantic image descriptions,” Procedia Computer Science, vol. 22, no. open access, pp. 285–294, 2013. [26] P. T. M. L. V. Vijayarajan, M. Dinakaran, “A generic framework for ontology based information retrieval and image retrieval in web data,” Human-centric Computing and Information Sciences, vol. 6, no. 18, pp. 1–30, 2016. [27] J. Z. N. C.-Y. W. X. Xie, X. Cai, “A semantic-based method for visualizing large image collections,” IEEE Transactions on Visualization and Computer Graphics, IEEE Computer Society, pp. 1–15, 2018. [Online]. Available: https://doi.org/10.1109/TVCG.2018.2835485 [28] T. X. C. X.-K. Y. W.-Y. M. T. Z. Y. Bai, W. Yu, “Bag-of-words based deep neural network for image retrieval,” in International Conference on Multimedia. Orlando, Florida, USA: ACM, 2014, pp. 229–232. [Online]. Available: https://dl.acm.org/citation.cfm?id=2656402 [29] J. W. Q. Y.-P. Y. Y. Cao, M. Long, “Deep visual-semantic hashing for cross-modal retrieval,” in Inter. Conf. on Knowl. Discovery and Data Mining, SIGKDD. California, USA: ACM, 2016, pp. 1445–1454. [Online]. Available: https://dl.acm.org/citation.cfm?id=2939812 [30] D. Z. Y. Chou, D.J. Lee, “Semantic-based brain mri image segmentation using convolutional neural network,” in Advances in Visual Computing. ISVC 2016. Lecture Notes in Computer Science, vol. 10072. Springer, Cham, 2016, pp. 628–638. [Online]. Available: https://link.springer.com/chapter/10.1007/978-3-319-50835-1 56 [31] W. H. Z. Zheng, H. Bu, “An approach to classify visual semantic based on visual encoding with the convolutional neural network,” in Proceedings of International Conference on Fuzzy Systems and Knowledge Discovery. Zhangjiajie, China: IEEE, 2015, pp. 854–858. [Online]. Available: https://ieeexplore.ieee.org/document/7382054/ Received on September 13, 2018 Revised on December 13, 2018
File đính kèm:
sir_dl_an_architecture_of_semantic_based_image_retrieval_usi.pdf