Multi - Criteria group decision making with picture linguistic numbers
In 2013, Cuong and Kreinovich defined picture fuzzy set (PFS) which is a direct extension of fuzzy set (FS) and
intuitionistic fuzzy set (IFS). Wang et al. (2014) proposed intuitionistic linguistic number (ILN) as a combination of
IFS and linguistic approach. Motivated by PFS and linguistic approach, this paper introduces the concept of picture
linguistic number (PLN), which constitutes a generalization of ILN for picture circumstances. For multi-criteria
group decision making (MCGDM) problems with picture linguistic information, we define a score index and two
accuracy indexes of PLNs, and propose an approach to the comparison between two PLNs. Simultaneously, some
operation laws for PLNs are defined and the related properties are studied. Further, some aggregation operations
are developed: picture linguistic arithmetic averaging (PLAA), picture linguistic weighted arithmetic averaging
(PLWAA), picture linguistic ordered weighted averaging (PLOWA) and picture linguistic hybrid averaging (PLHA)
operators. Finally, based on the PLWAA and PLHA operators, we propose an approach to handle MCGDM under
PLN environment.
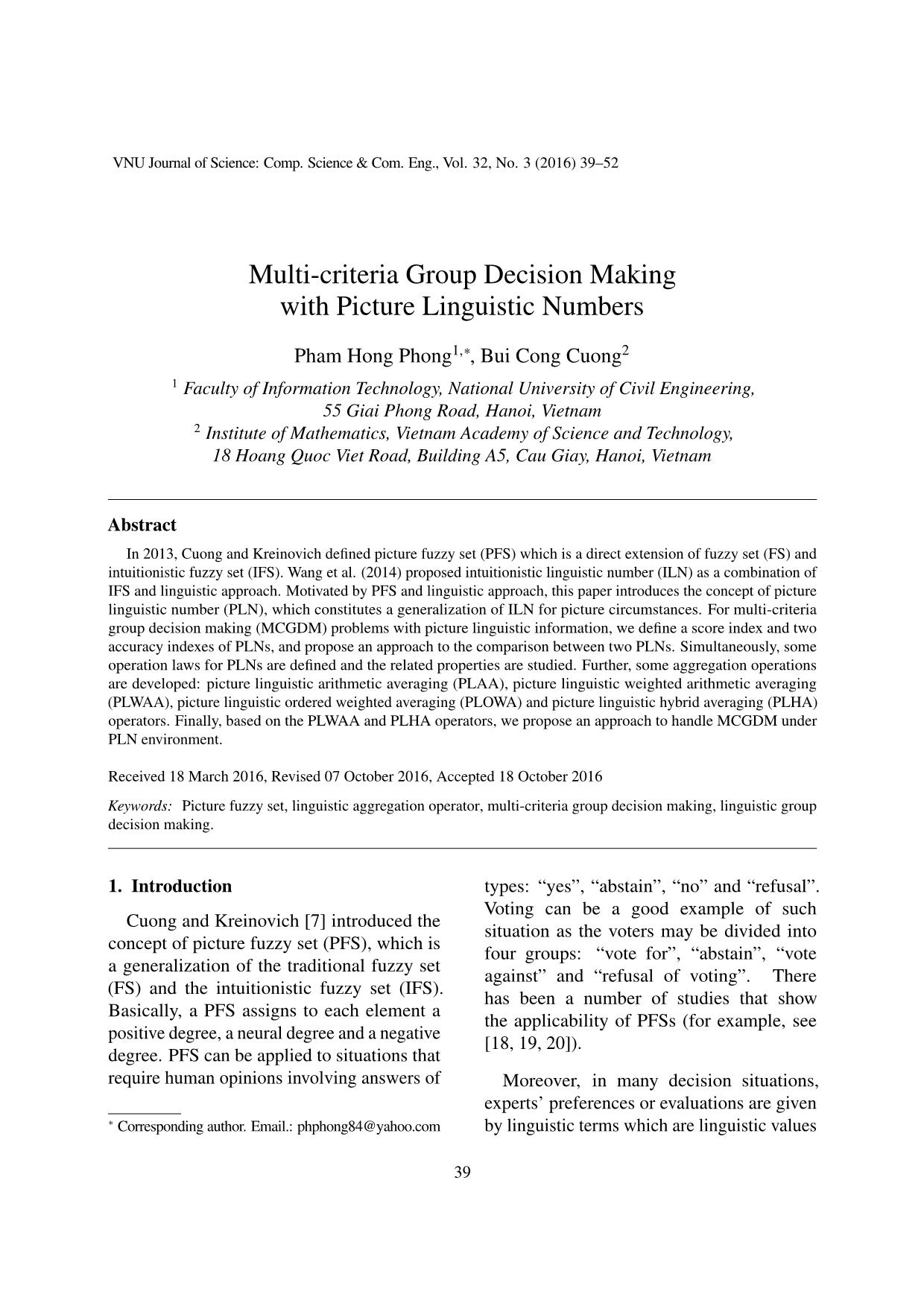
Trang 1
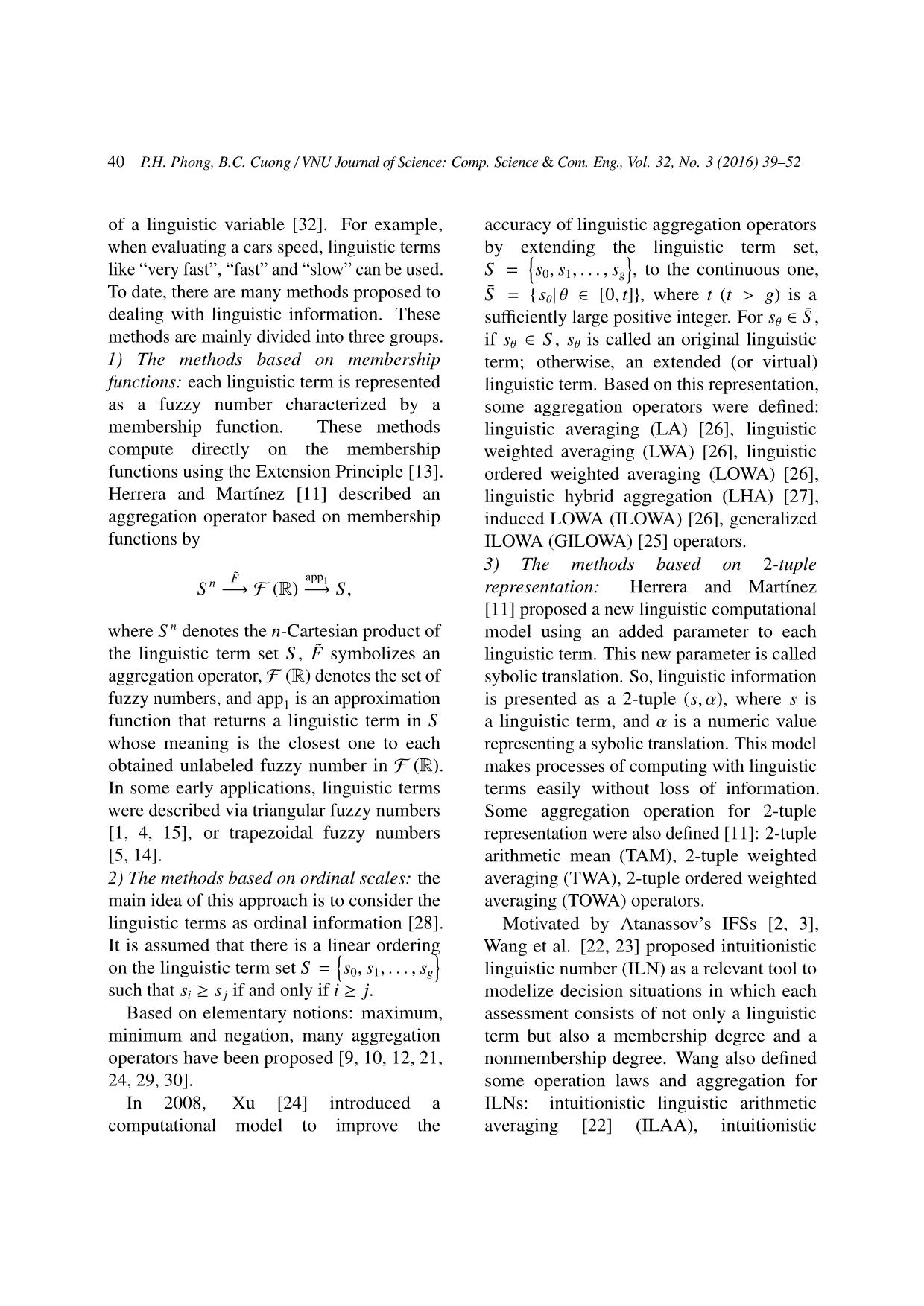
Trang 2
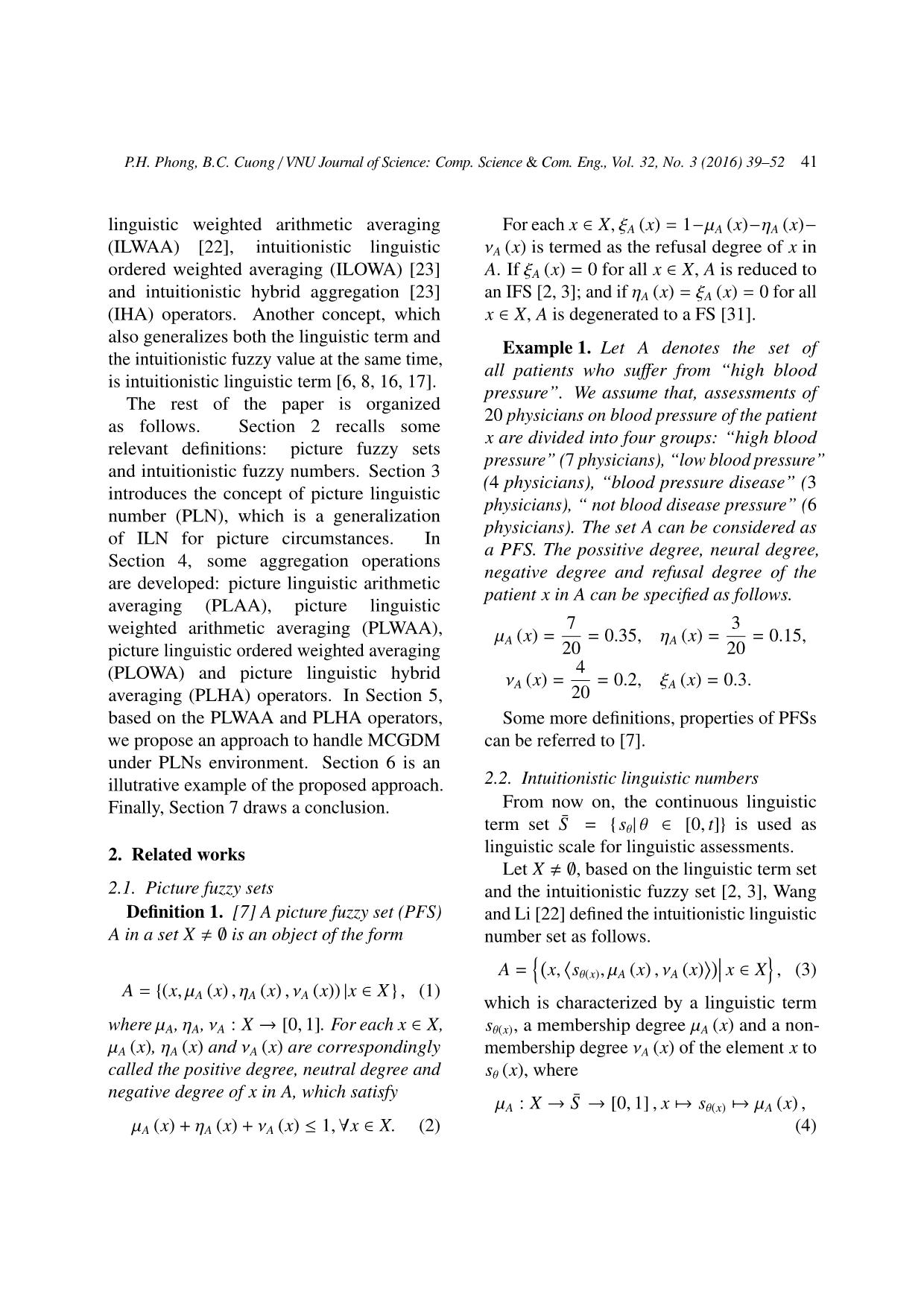
Trang 3
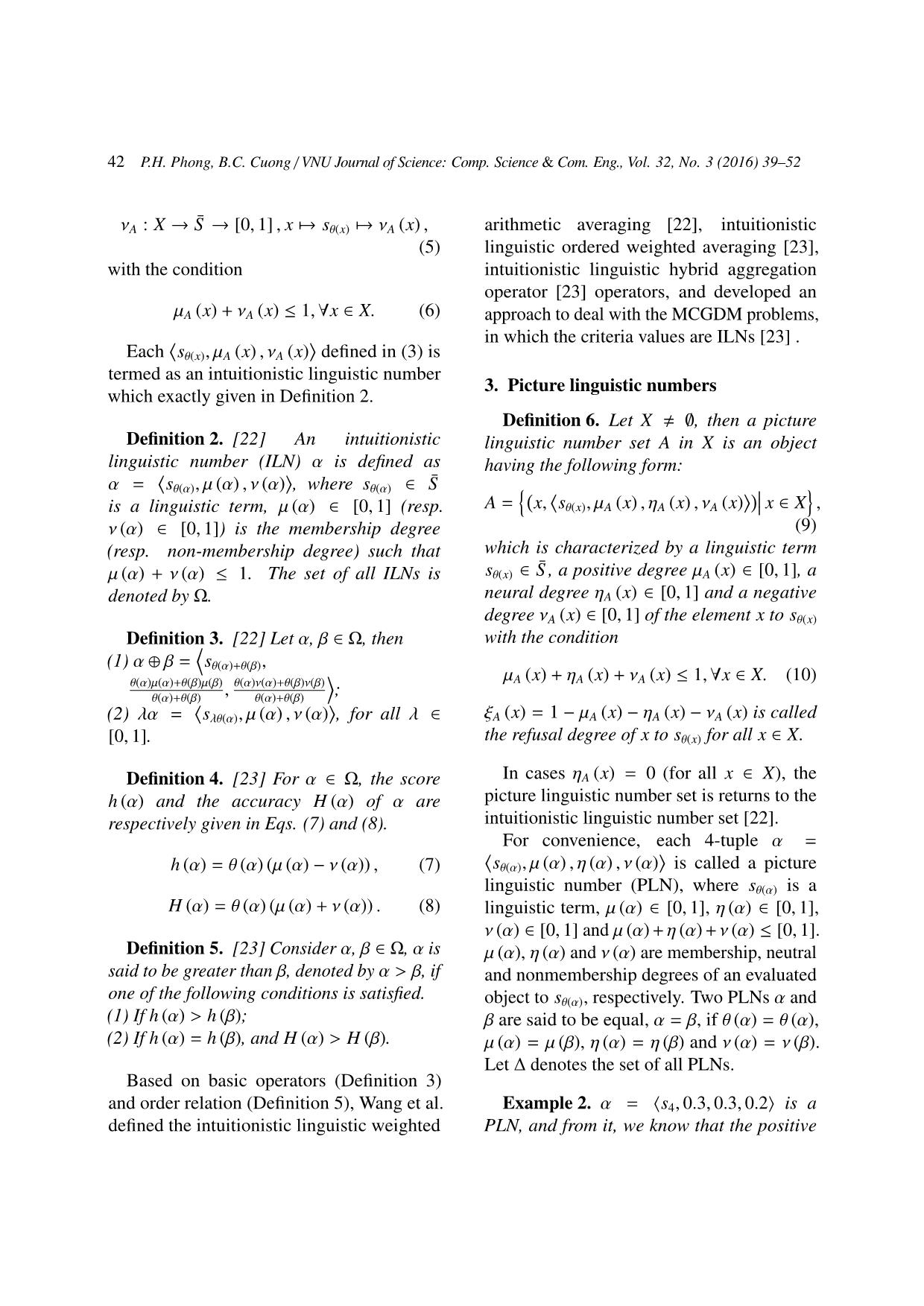
Trang 4
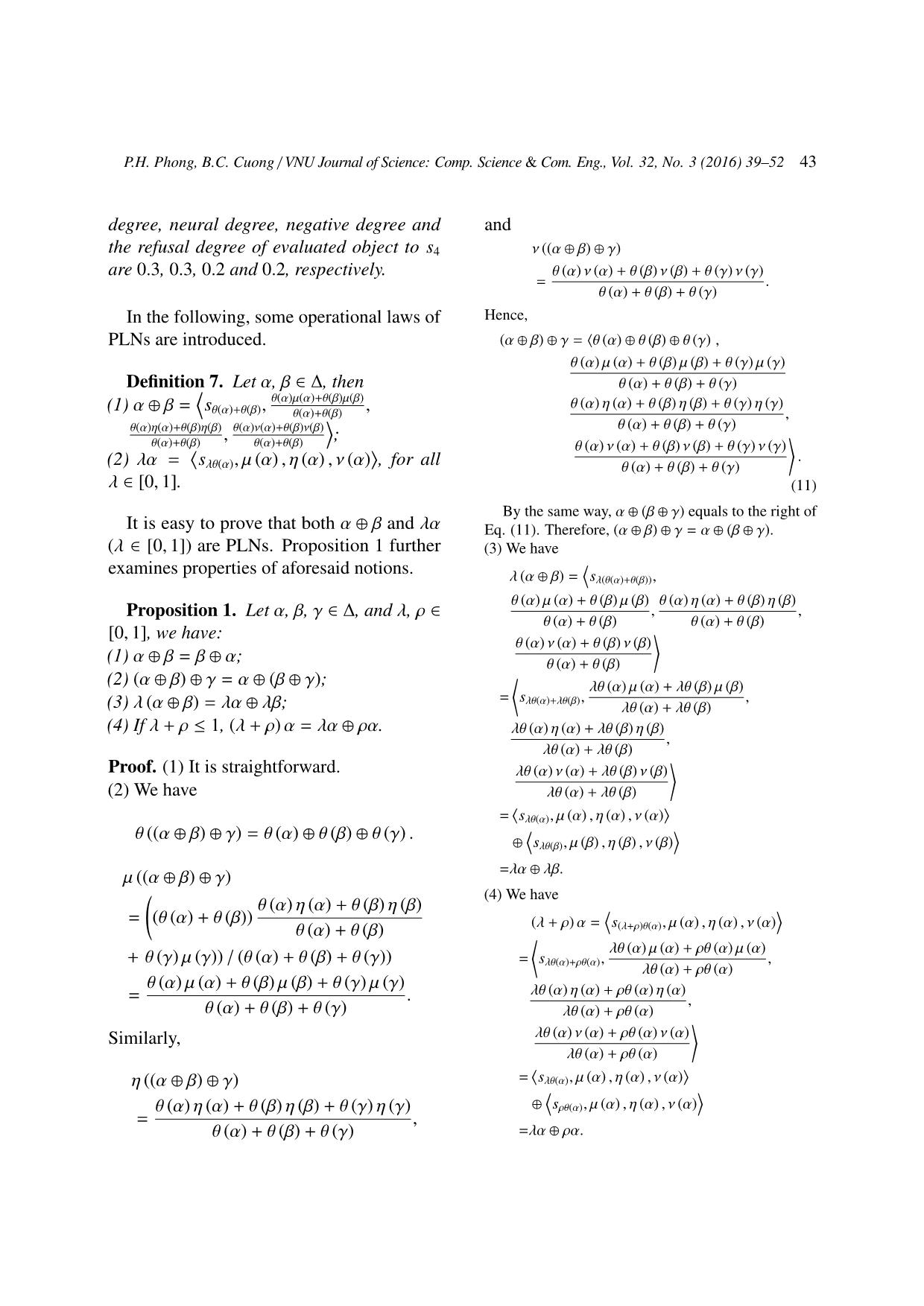
Trang 5
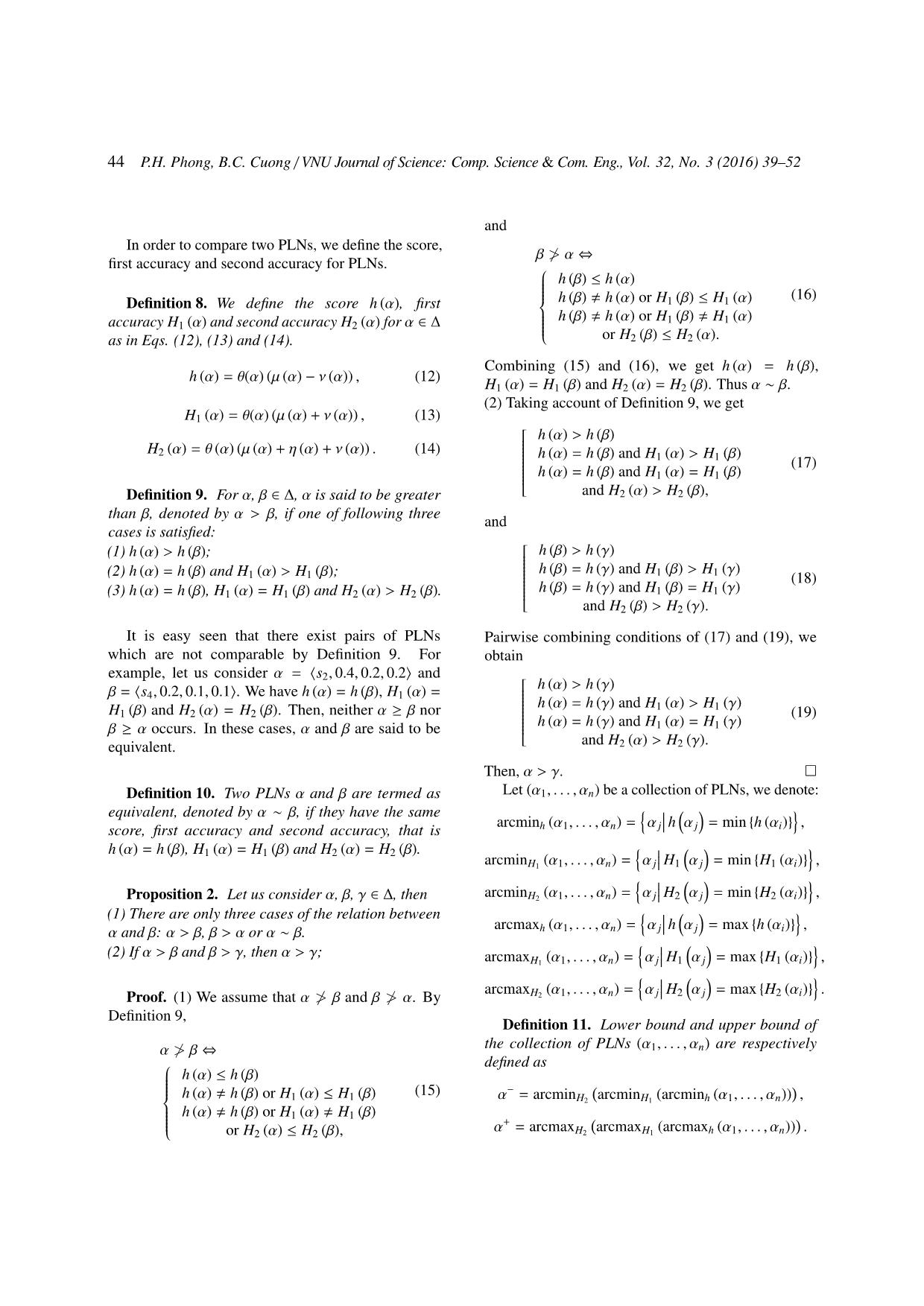
Trang 6
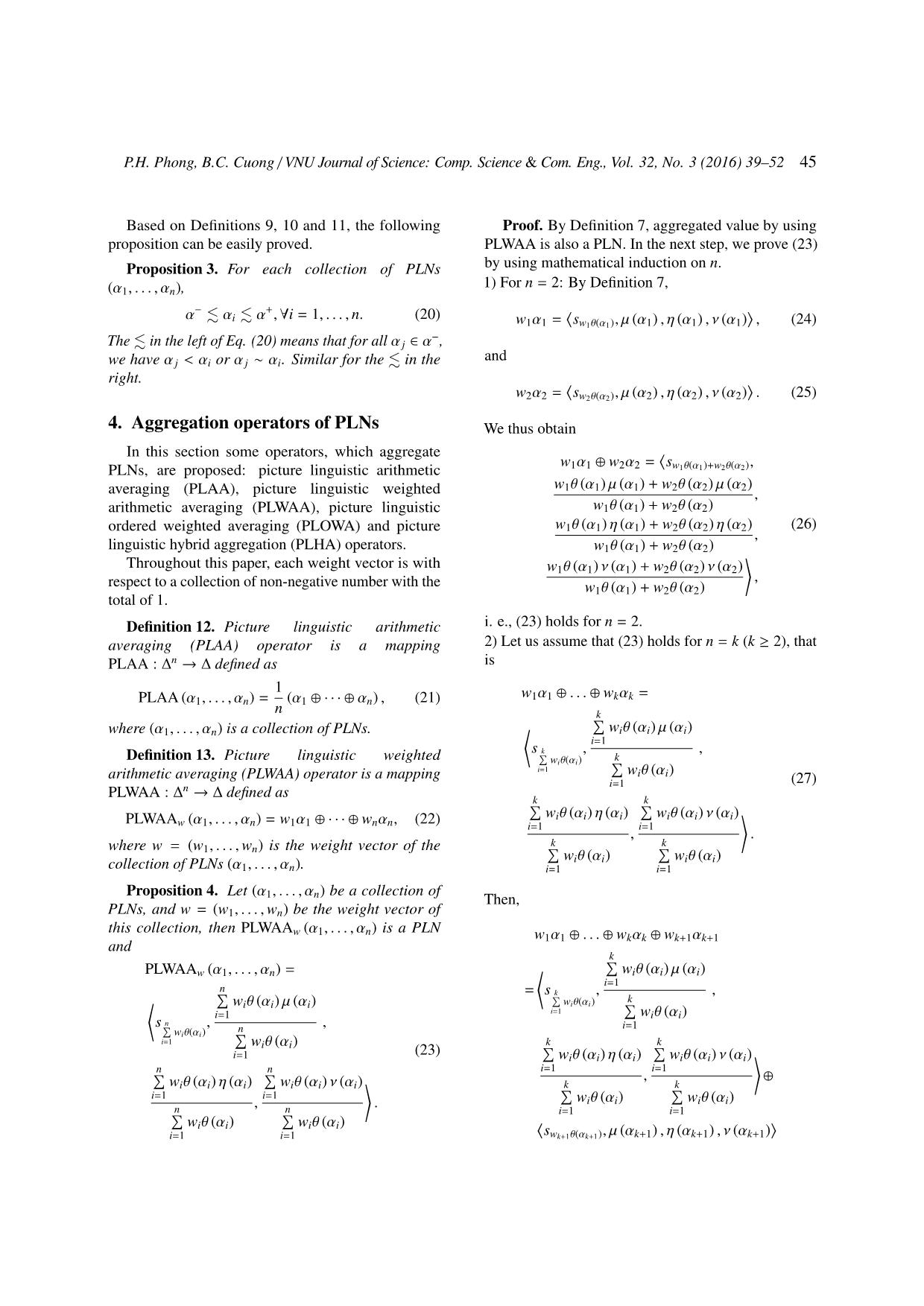
Trang 7
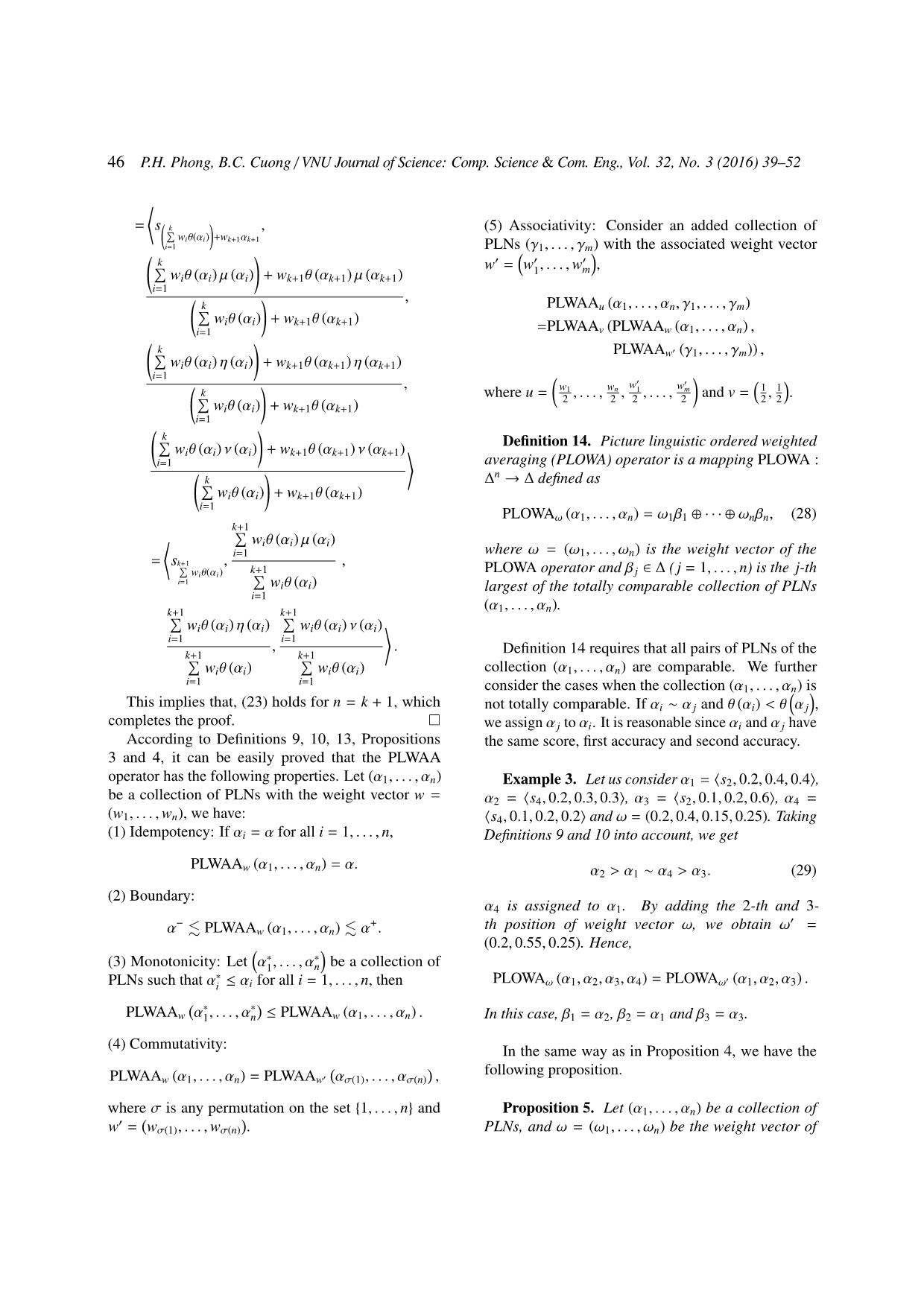
Trang 8
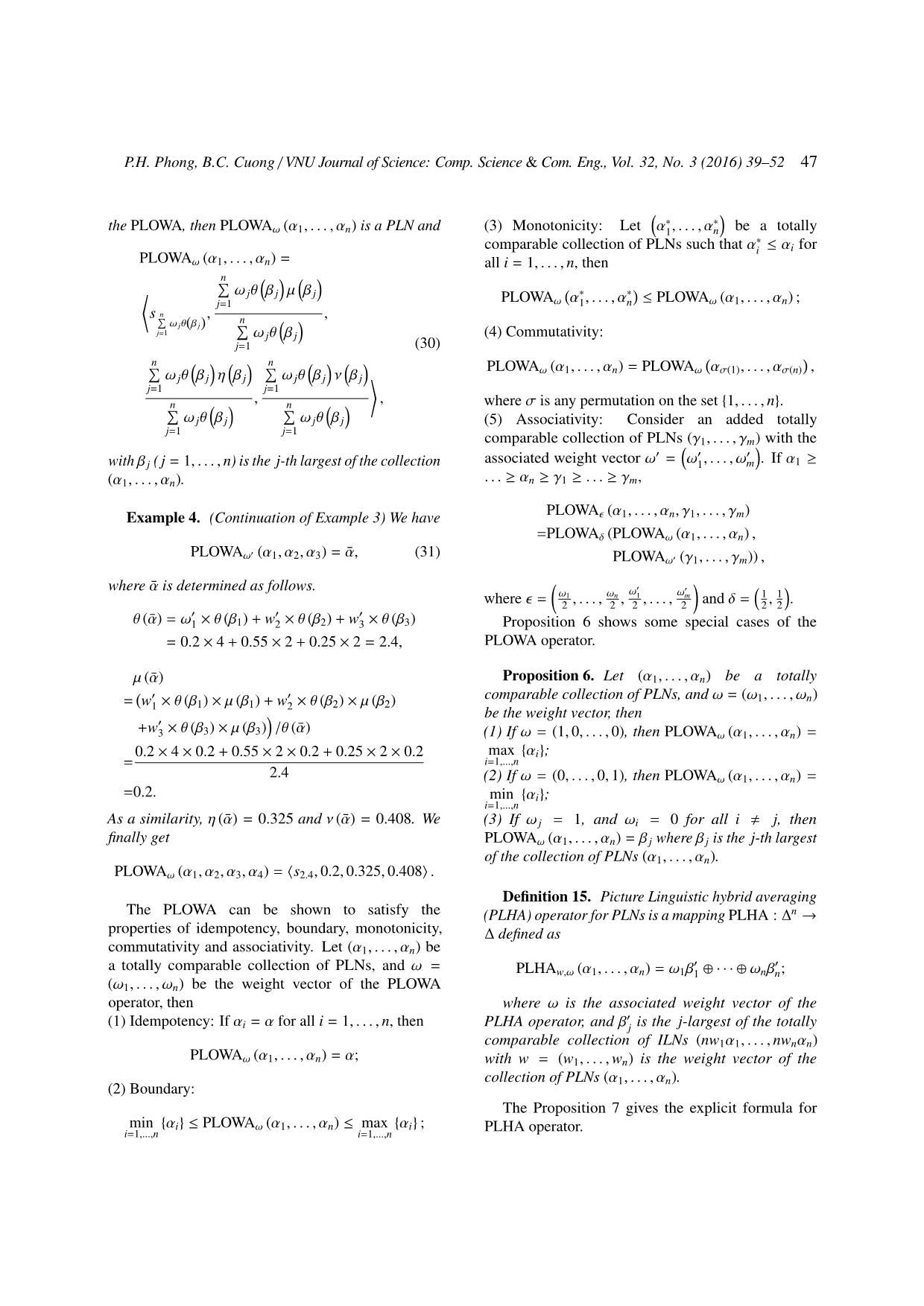
Trang 9
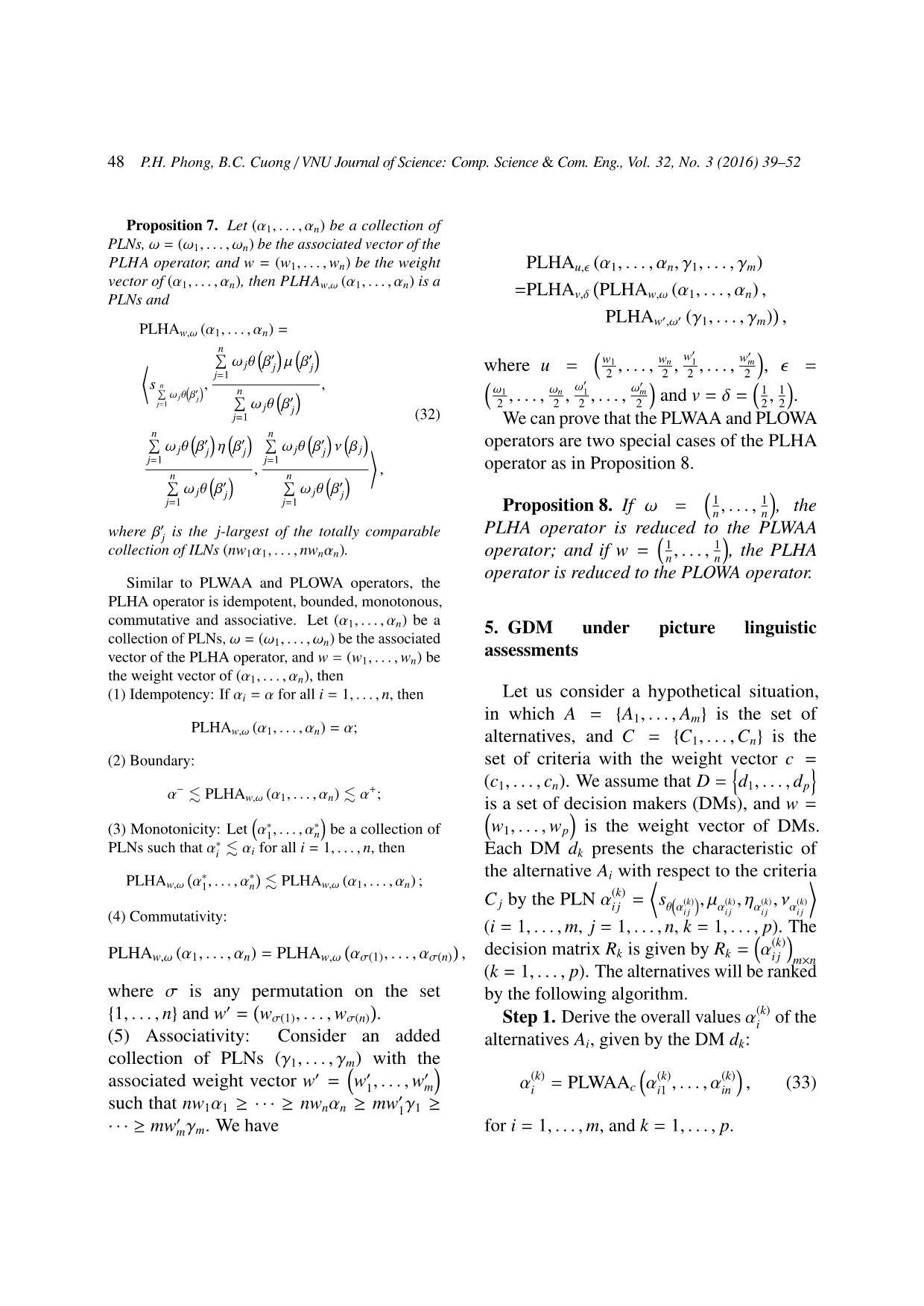
Trang 10
Tải về để xem bản đầy đủ
Tóm tắt nội dung tài liệu: Multi - Criteria group decision making with picture linguistic numbers
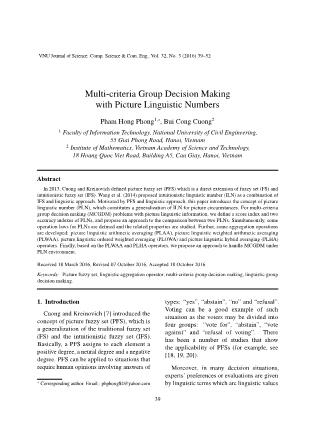
PLOWA (α1, . . . , αn, γ1, . . . , γm) =PLOWAδ (PLOWAω (α1, . . . , αn) , PLOWA 0 (α , α , α ) = α,¯ (31) ω 1 2 3 PLOWAω0 (γ1, . . . , γm)) , where α¯ is determined as follows. 0 0 ω1 ωn ω1 ωm 1 1 where = 2 ,..., 2 , 2 ,..., 2 and δ = 2 , 2 . 0 0 0 θ (α¯) = ω1 × θ (β1) + w2 × θ (β2) + w3 × θ (β3) Proposition 6 shows some special cases of the = 0.2 × 4 + 0.55 × 2 + 0.25 × 2 = 2.4, PLOWA operator. µ (α¯) Proposition 6. Let (α1, . . . , αn) be a totally 0 0 comparable collection of PLNs, and ω = (ω1, . . . , ωn) = w1 × θ (β1) × µ (β1) + w2 × θ (β2) × µ (β2) be the weight vector, then w0 × θ (β ) × µ (β ) /θ (α) + 3 3 3 ¯ (1) If ω = (1, 0,..., 0), then PLOWAω (α1, . . . , αn) = 0.2 × 4 × 0.2 + 0.55 × 2 × 0.2 + 0.25 × 2 × 0.2 max {αi}; = i=1,...,n 2.4 (2) If ω = (0,..., 0, 1), then PLOWAω (α1, . . . , αn) = =0.2. min {αi}; i=1,...,n As a similarity, η (α¯) = 0.325 and ν (α¯) = 0.408. We (3) If ω j = 1, and ωi = 0 for all i , j, then finally get PLOWAω (α1, . . . , αn) = β j where β j is the j-th largest of the collection of PLNs (α1, . . . , αn). PLOWAω (α1, α2, α3, α4) = hs2.4, 0.2, 0.325, 0.408i . Definition 15. Picture Linguistic hybrid averaging The PLOWA can be shown to satisfy the (PLHA) operator for PLNs is a mapping PLHA : ∆n → properties of idempotency, boundary, monotonicity, ∆ defined as commutativity and associativity. Let (α1, . . . , αn) be a totally comparable collection of PLNs, and ω = 0 0 PLHAw,ω (α1, . . . , αn) = ω1β1 ⊕ · · · ⊕ ωnβn; (ω1, . . . , ωn) be the weight vector of the PLOWA operator, then where ω is the associated weight vector of the 0 (1) Idempotency: If αi = α for all i = 1,..., n, then PLHA operator, and β j is the j-largest of the totally comparable collection of ILNs (nw1α1,..., nwnαn) PLOWAω (α1, . . . , αn) = α; with w = (w1,..., wn) is the weight vector of the collection of PLNs (α1, . . . , αn). (2) Boundary: The Proposition 7 gives the explicit formula for min {αi} ≤ PLOWAω (α1, . . . , αn) ≤ max {αi} ; i=1,...,n i=1,...,n PLHA operator. 48 P.H. Phong, B.C. Cuong / VNU Journal of Science: Comp. Science & Com. Eng., Vol. 32, No. 3 (2016) 39–52 Proposition 7. Let (α1, . . . , αn) be a collection of PLNs, ω = (ω1, . . . , ωn) be the associated vector of the PLHA operator, and w = (w1,..., wn) be the weight PLHAu, (α1, . . . , αn, γ1, . . . , γm) vector of (α1, . . . , αn), then PLHAw,ω (α1, . . . , αn) is a =PLHAv,δ PLHAw,ω (α1, . . . , αn) , PLNs and PLHAw0,ω0 (γ1, . . . , γm) , PLHAw,ω (α1, . . . , αn) = n P 0 0 0 0 w1 wn w1 wm * ω jθ β j µ β j where u = ,..., , ,..., , = j=1 2 2 2 2 s n , , ω0 ω0 P ω θ β0 n ω1 ωn 1 m 1 1 j j P 0 ,..., , ,..., and v = δ = , . j=1 2 2 2 2 2 2 ω jθ β j j=1 (32) We can prove that the PLWAA and PLOWA n n P 0 0 P 0 operators are two special cases of the PLHA ω jθ β j η β j ω jθ β j ν β j + j=1 j=1 operator as in Proposition 8. n , n , P 0 P 0 ω jθ β j ω jθ β j j=1 j=1 1 1 Proposition 8. If ω = n ,..., n , the where β0 is the j-largest of the totally comparable PLHA operator is reduced to the PLWAA j collection of ILNs (nw α ,..., nw α ). 1 1 1 1 n n operator; and if w = n ,..., n , the PLHA operator is reduced to the PLOWA operator. Similar to PLWAA and PLOWA operators, the PLHA operator is idempotent, bounded, monotonous, commutative and associative. Let (α1, . . . , αn) be a 5. GDM under picture linguistic collection of PLNs, ω = (ω , . . . , ω ) be the associated 1 n assessments vector of the PLHA operator, and w = (w1,..., wn) be the weight vector of (α1, . . . , αn), then (1) Idempotency: If αi = α for all i = 1,..., n, then Let us consider a hypothetical situation, in which A = {A1,..., Am} is the set of PLHAw,ω (α1, . . . , αn) = α; alternatives, and C = {C1,..., Cn} is the c (2) Boundary: set of criteria with the weight vectorn =o (c1,..., cn). We assume that D = d1,..., dp α− PLHA (α , . . . , α ) α+; . w,ω 1 n . is a set of decision makers (DMs), and w = ∗ ∗ w ,..., w is the weight vector of DMs. (3) Monotonicity: Let α1, . . . , αn be a collection of 1 p ∗ PLNs such that αi . αi for all i = 1,..., n, then Each DM dk presents the characteristic of ∗ ∗ the alternative Ai with respect to the criteria PLHAw,ω α1, . . . , αn . PLHAw,ω (α1, . . . , αn) ; (k) C j by the PLN αi j = sθ α(k) , µα(k) , ηα(k) , να(k) (4) Commutativity: i j i j i j i j (i = 1,..., m, j = 1,..., n, k = 1,..., p). The (k) decision matrix Rk is given by Rk = α PLHAw,ω (α1, . . . , αn) = PLHAw,ω ασ(1), . . . , ασ(n) , i j m×n (k = 1,..., p). The alternatives will be ranked where σ is any permutation on the set by the following algorithm. 0 (k) {1,..., n} and w = wσ(1),..., wσ(n) . Step 1. Derive the overall values αi of the (5) Associativity: Consider an added alternatives Ai, given by the DM dk: collection of PLNs (γ , . . . , γ ) with the 1 m associated weight vector w0 = w0 ,..., w0 (k) (k) (k) 1 m αi = PLWAAc αi1 , . . . , αin , (33) 0 such that nw1α1 ≥ · · · ≥ nwnαn ≥ mw1γ1 ≥ 0 · · · ≥ mwmγm. We have for i = 1,..., m, and k = 1,..., p. P.H. Phong, B.C. Cuong / VNU Journal of Science: Comp. Science & Com. Eng., Vol. 32, No. 3 (2016) 39–52 49 Table 1. Decision matrix R1 C1 C2 C3 A1 hs4, 0.6, 0.1, 0.2i hs4, 0.4, 0.2, 0.2i hs5, 0.2, 0.3, 0.5i A2 hs5, 0.7, 0.2, 0.1i hs4, 0.4, 0.1, 0.4i hs4, 0.5, 0.2, 0.3i A3 hs5, 0.3, 0.1, 0.4i hs5, 0.4, 0.3, 0.3i hs6, 0.7, 0.1, 0.2i A4 hs4, 0.6, 0.1, 0.2i hs4, 0.6, 0.1, 0.2i hs5, 0.3, 0.1, 0.5i Step 2. Derive the collective overall values Ai (i = 1, 2, 3, 4) by the PLHA operator with αi by aggregating the individual overall values associated weight vector ω = (0.2, 0.5, 0.3). (1) (p) αi , . . . , αi : α1 = hs4.40, 0.3965, 0.2045, 0.3438i , (1) (p) h i αi = PLHAw,ω αi , . . . , αi , (34) α2 = s4.57, 0.3481, 0.1428, 0.4040 , α = hs , 0.3628, 0.1666, 0.4050i , 3 5.32 where ω = ω1, . . . , ωp is the weight vector α4 = hs5.16, 0.4098, 0.1510, 0.3948i . of the PLHA operator (i = 1,..., m). Step 3. Calculate the scores h (αi), first Step 3. By eq. (12), accuracies H1 (αi) and second accuracies h (α1) = 0.2318, h (α2) = −0.2556 H2 (αi) (i = 1,..., m), rank the alternatives − by using Definition 9 (the alternative Ai1 is h (α3) = 0.2246, h (α4) = 0.078. called to be better than the alternative Ai , 2 By Definition 9, denoted by Ai1 > Ai2 , iff αi1 > αi2 , for all i1, i2 = 1,..., m). h (α1) > h (α4) > h (α3) > h (α2) 6. An illutrative example then A1 > A4 > A3 > A2. This situation concerns four alternative 7. Conclusion enterprises, which will be chosen by three DMs whose weight vector is w = In this paper, motivated by picture fuzzy (0.3, 0.4, 0.3). The enterprises will be sets and linguistic approaches, the notion considered under three criteria C1, C2 and C3. of picture linguistic numbers are first Assume that the weight vector of the criteria defined. We propose the score, first accuracy is c = (0.37, 0.35, 0.28). Three decision and second accuracy of picture linguistic matrices are listed in Tabs. 1, 2 and 3. numbers, and propose a simple approach Step 1. Using explicit form of the PLWAA for the comparison between two picture operation given in Eq. 23, we obtain overall linguistic numbers. Simultaneously, the (k) values αi of the alternatives Ai given by the operation laws for picture linguistic numbers DMs dk (i = 1, 2, 3, 4 and k = 1, 2, 3) as are given and the accompanied properties are in Tab. 4. studied. Further, some aggregation operators Step 2. Aggregate all the individual overall are developed: picture linguistic arithmetic (1) (2) (3) values αi , αi and αi of the alternatives averaging, picture linguistic weighted 50 P.H. Phong, B.C. Cuong / VNU Journal of Science: Comp. Science & Com. Eng., Vol. 32, No. 3 (2016) 39–52 Table 2. Decision matrix R2 C1 C2 C3 A1 hs4, 0.7, 0.1, 0.2i hs6, 0.2, 0.2, 0.5i hs4, 0.7, 0.2, 0.1i A2 hs3, 0.2, 0.2, 0.6i hs5, 0.5, 0.1, 0.2i hs5, 0.3, 0.1, 0.4i A3 hs4, 0.2, 0.1, 0.5i hs7, 0.2, 0.2, 0.6i hs5, 0.1, 0.2, 0.6i A4 hs5, 0.7, 0.2, 0.1i hs5, 0.2, 0.1, 0.7i hs4, 0.6, 0.1, 0.2i Table 3. Decision matrix R3 C1 C2 C3 A1 hs4, 0.6, 0.3, 0.1i hs6, 0.2, 0.3, 0.5i hs5, 0.2, 0.1, 0.7i A2 hs3, 0.2, 0.2, 0.5i hs5, 0.2, 0.1, 0.6i hs6, 0.2, 0.2, 0.6i A3 hs5, 0.3, 0.2, 0.5i hs7, 0.8, 0.1, 0.1i hs5, 0.2, 0.2, 0.5i A4 hs3, 0.7, 0.1, 0.2i hs5, 0.2, 0.2, 0.5i hs6, 0.3, 0.1, 0.6i (k) Table 4. Overall values αi of the alternatives Ai given by the DMs dk (i = 1, 2, 3, 4; k = 1, 2, 3) d1 d2 d3 A1 hs4.28, 0.4037, 0.1981, 0.2981i hs4.70, 0.4766, 0.1685, 0.3102i hs4.98, 0.3189, 0.2438, 0.4373i A2 hs4.37, 0.5526, 0.1680, 0.2474i hs4.26, 0.3561, 0.1261, 0.3700i hs4.54, 0.2000, 0.1615, 0.5756i A3 hs5.28, 0.4604, 0.1663, 0.3032i hs5.33, 0.1737, 0.1722, 0.5722i hs5.70, 0.4904, 0.1570, 0.3281i A4 hs4.28, 0.5019, 0.1000, 0.2981i hs5.28, 0.4070, 0.1682, 0.3917i hs4.54, 0.3593, 0.1385, 0.4637i arithmetic averaging, picture linguistic References ordered weighted averaging and picture [1] G. I. Adamopoulos and G. P. Pappis, A linguistic hybrid aggregation operators. fuzzy linguistic approach to a multicriteria Finally, based on the picture linguistic sequencing problem, European Journal of weighted arithmetic averaging and the picture Operational Research 92 (1996) 628–636. linguistic hybrid aggregation operators, we [2] K. T. Atanassov, Intuitionistic fuzzy sets, propose an approach to handle multi-criteria Fuzzy Sets and Systems 20 (1986) 87–96. [3] K. T. Atanassov and S. Stoeva, Intuitionistic group decision making problems under L-fuzzy sets, in Cybernetics and Systems picture linguistic environment. Research, eds. R. Trappl (Elsevier Science Pub., Amsterdam, 1986), pp. 539–540. [4] P. Chang and Y. Chen, A fuzzy multicriteria Acknowledgments decision making method for technology transfer strategy selection in biotechnology, This research is funded by the Vietnam Fuzzy Sets and Systems 63 (1994) 131-139. National Foundation for Science and [5] S. M. Chen, A new method for tool steel Technology Development (NAFOSTED) materials selection under fuzzy environment, under grant number 102.01- 2017.02. Fuzzy Sets and Systems 92 (1997) 265–274. [6] Z. C. Chen, P. H. Liu and Z. Pei, An approach to multiple attribute group decision making based on linguistic intuitionistic fuzzy numbers, International Journal of P.H. Phong, B.C. Cuong / VNU Journal of Science: Comp. Science & Com. Eng., Vol. 32, No. 3 (2016) 39–52 51 Computational Intelligence Systems 8 (4) fuzzy sets, Journal of Intelligent & Fuzzy (2015) 747–760. Systems28 (2) (2015) 591-604. [7] B. C. Cuong and V. Kreinovich, Picture [19] L. H. Son, DPFCM: A novel distributed fuzzy sets - a new concept for computational picture fuzzy clustering method on picture intelligence problems, in Proc. 3rd world fuzzy sets, Expert Systems with Applications congress on information and communication 42 (2015) 51–66. technologies (WICT 2013) pp. 1–6. [20] P. H. Thong and L. H. Son, Picture [8] B. C. Cuong and P. H. Phong, Max - fuzzy clustering: a new computational Min Composition of Linguistic Intuitionistic intelligence method, Soft Computing DOI Fuzzy Relations and Application in Medical 10.1007/s00500-015-1712-7 Diagnosis, VNU Journal of Science: Comp. [21] V. Torra, The weighted OWA operator, Science & Com. Eng. 30 (4) (2014) 601–968. International Journal of Intelligent Systems [9] F. Herrera and J. L. Verdegay, Linguistic 12 (1997) 153–166. assessments in group decision”, in Proc. [22] J. Q. Wang and H. B. Li, Multi-criteria First European Congress on Fuzzy and decision-making method based on Intelligent Technologies (Aachen, 1993) pp. aggregation operators for intuitionistic 941–948. linguistic fuzzy numbers, Control and [10] F. Herrera and E. Herrera-Viedma, Decision 25 (10) (2010) 1571–1574, 1584. Aggregation operators for linguistic [23] X. F. Wang, J. Q. Wang and W. E. Yang, weighted information, IEEE Transactions Multi-criteria group decision making method on Systems, Man, and Cybernetics-Part A 27 based on intuitionistic linguistic aggregation (1997), 646–656. operators, Journal of Intelligent & Fuzzy [11] F. Herrera and L. Mart´ınez, A 2-tuple Systems 26 (2014), 115–125. fuzzy linguistic representation model for [24] Z. S. Xu, Uncertain Multiple Attribute computing with words, IEEE Transactions Decision Making: Methods and Applications on Fuzzy Systems 8 (2000) 746–752. (Tsinghua University Press, Beijing, 2004). [12] F. Herrera and E. Herrera-Viedma, Choice [25] Z. S. Xu , Method based on fuzzy linguistic functions and mechanisms for linguistic assessments and GIOWA operator in multi- preference relations, European Journal of attribute group decision making, Journal of Operational Research 120 (2000) 144–161. Systems Science and Mathematical Sciences [13] G. J. Klir and B. Yuan, Fuzzy sets an fuzzy 24 (2004) 218–224. logic: Theory and Applications (Prentice- [26] Z. S. Xu, On generalized induced linguistic Hall PTR, 1995). aggregation operators, International Journal [14] C. K. Law, Using fuzzy numbers in of General Systems 35 (2006) 17–28. educational grading systems, Fuzzy Sets and [27] Z. S. Xu, A note on linguistic hybrid Systems 83 (1996) 311–323. arithmetic averaging operator in group [15] H. M. Lee, Applying fuzzy set theory decision making with linguistic information, to evaluate the rate of aggregative risk Group Decision and Negotiation 15 (2006) in software development, Fuzzy Sets and 581–591. Systems 80 (1996) 323–336. [28] R. R. Yager, A new methodology for ordinal [16] P. H. Phong and B. C. Cuong, Some multiobjective decisions based on fuzzy sets, intuitionistic linguistic aggregation Decision Sciences 12 (1981) 589–600. operators, Journal of Computer Science and [29] R. R. Yager, Applications and extensions of Cybernetics 30 (3) (2014) 216–226. OWA aggregations, International Journal of [17] P. H. Phong and B. C. Cuong, Symbolic Man-Machine Studied 37 (1992) 103–132. computational models for intuitionistic [30] R. R. Yager and A. Rybalov, Understanding linguistic information, Journal of Computer the median as a fusion operator, Science and Cybernetics 32 (1) (2016) 30– International Journal of General Systems 26 44. (1997) 239-263. [18] P. Singh, Correlation coefficients for picture 52 P.H. Phong, B.C. Cuong / VNU Journal of Science: Comp. Science & Com. Eng., Vol. 32, No. 3 (2016) 39–52 [31] L. A. Zadeh, Fuzzy sets, Information and variable and its application to approximate Control 8, 338–353. reasoning-I, Information Sciences 8 (3) [32] L. A. Zadeh, The concept of a linguistic (1975)199–249.
File đính kèm:
multi_criteria_group_decision_making_with_picture_linguistic.pdf