Một cách tiếp cận mới cho khoảng cách đối ngẫu bằng không của bài toán tối ưu véctơ sử dụng tập đặc trưng
Trong bài viết này, chúng tôi đề xuất các kết quả về khoảng cách đối ngẫu bằng không trong
bài toán tối ưu véctơ trên một không gian vectơ tôpô Hausdorff lồi địa phương với một hàm mục
tiêu có giá trị vectơ được cực tiểu hóa dưới một tập và một ràng buộc nón lồi. Các kết quả này
sau đó được áp dụng cho bài toán quy hoạch tuyến tính.
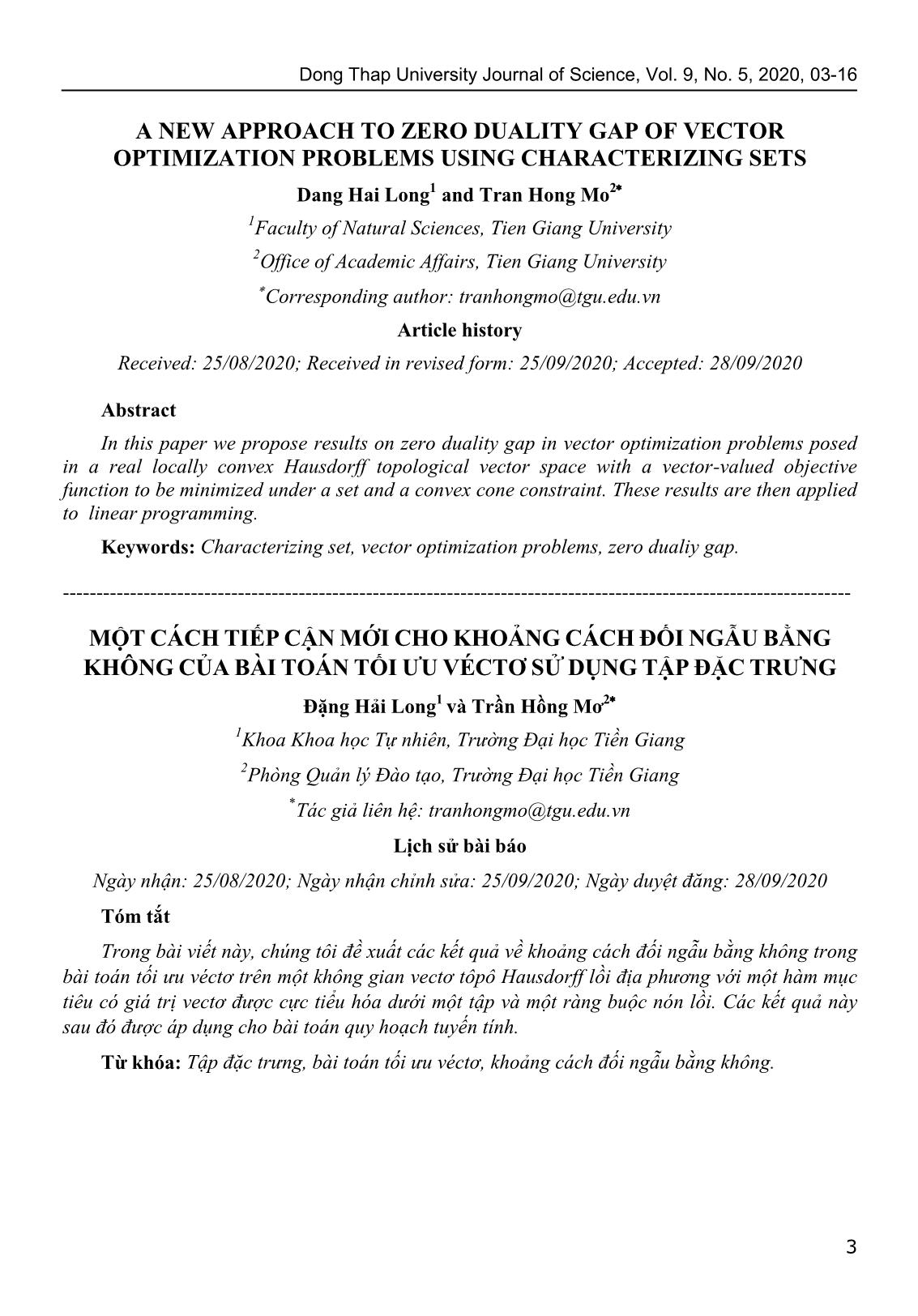
Trang 1
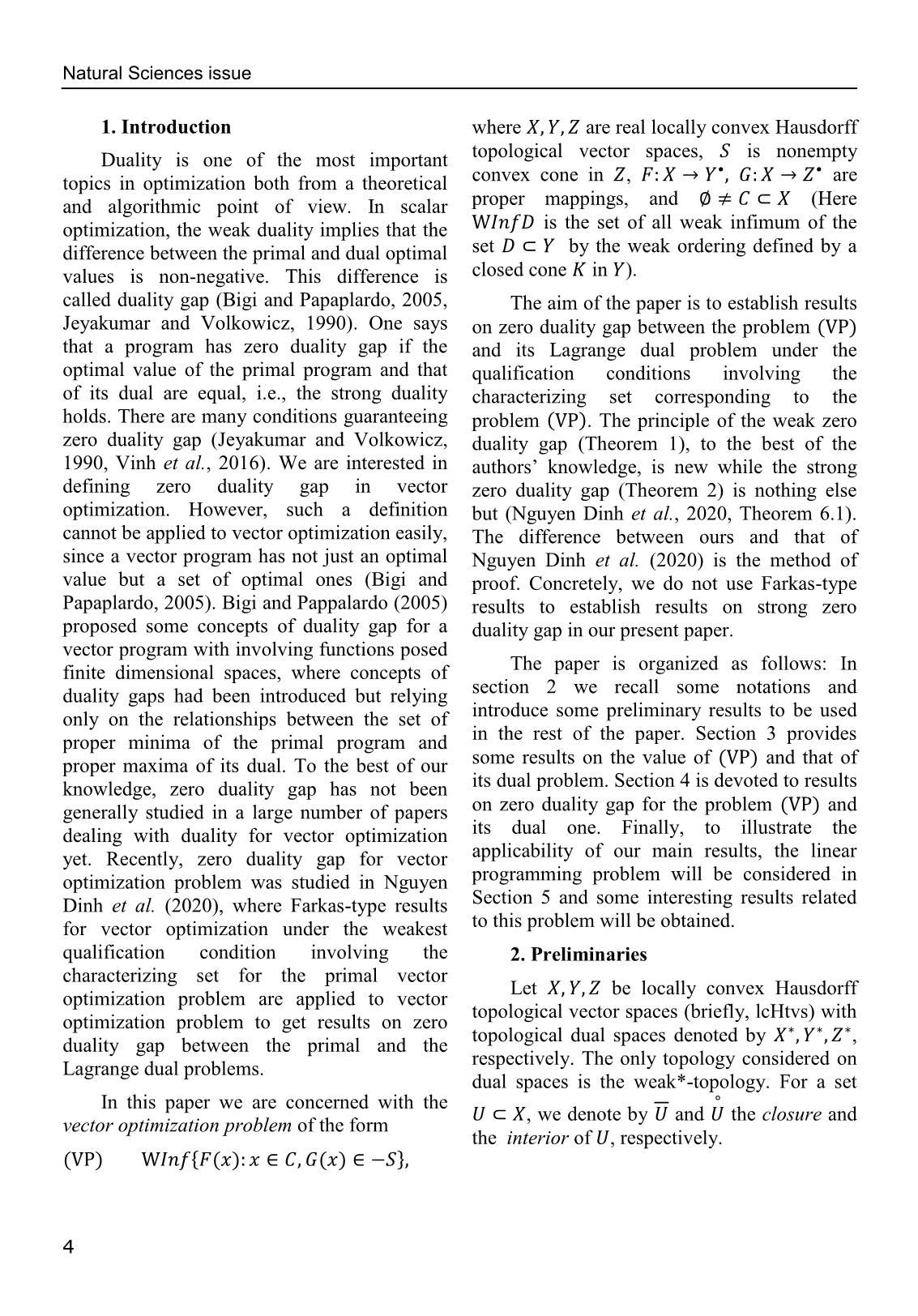
Trang 2
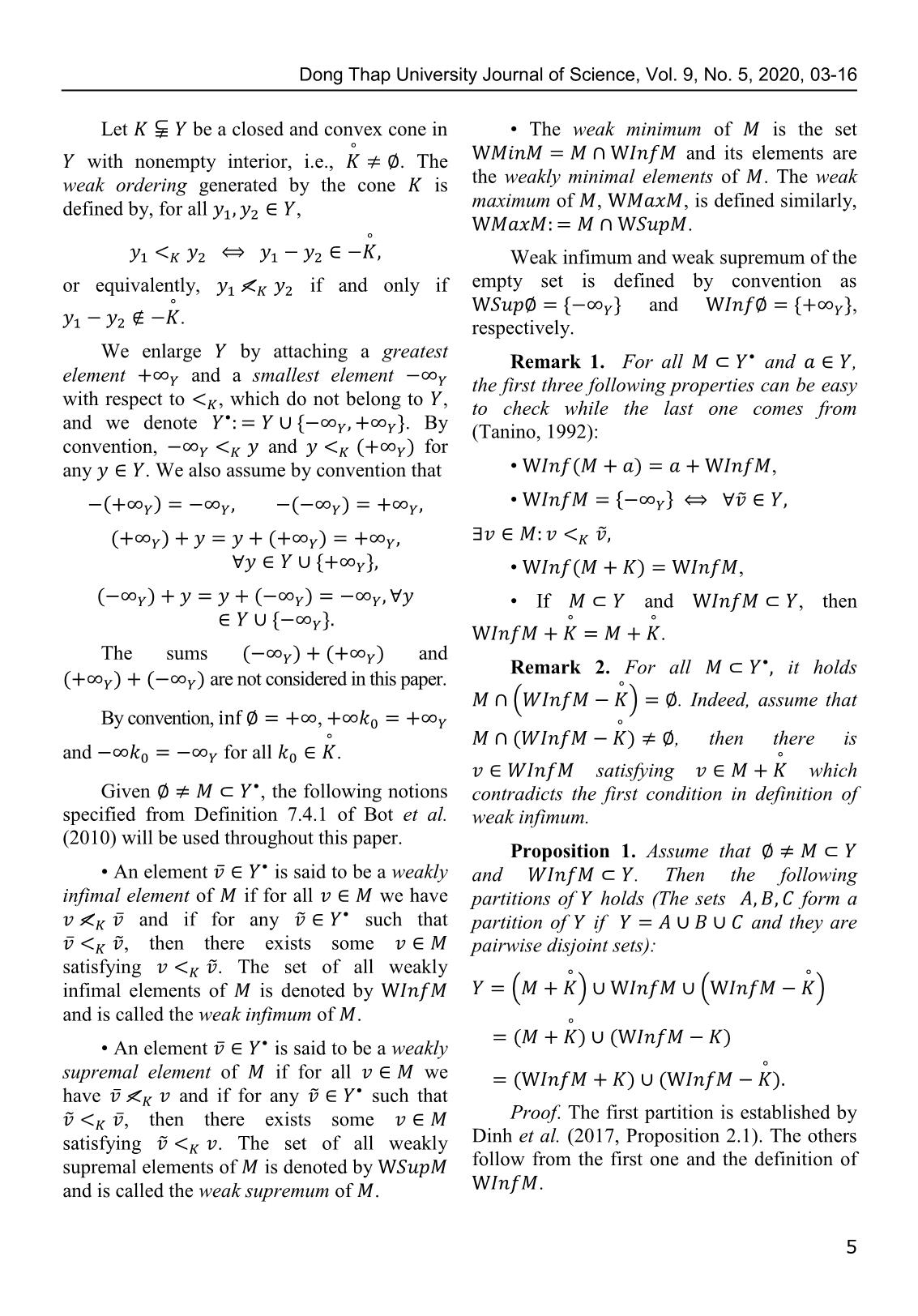
Trang 3
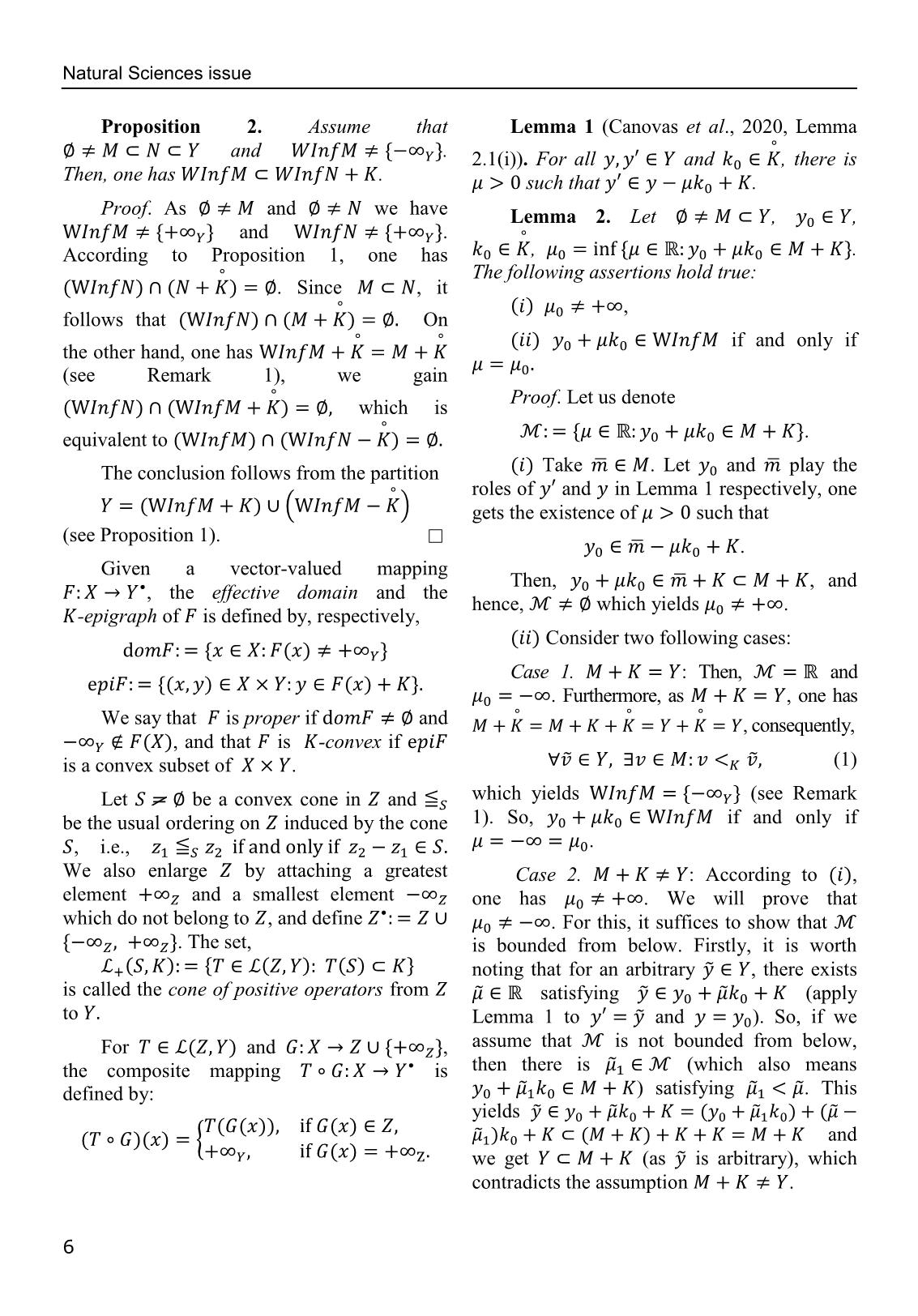
Trang 4
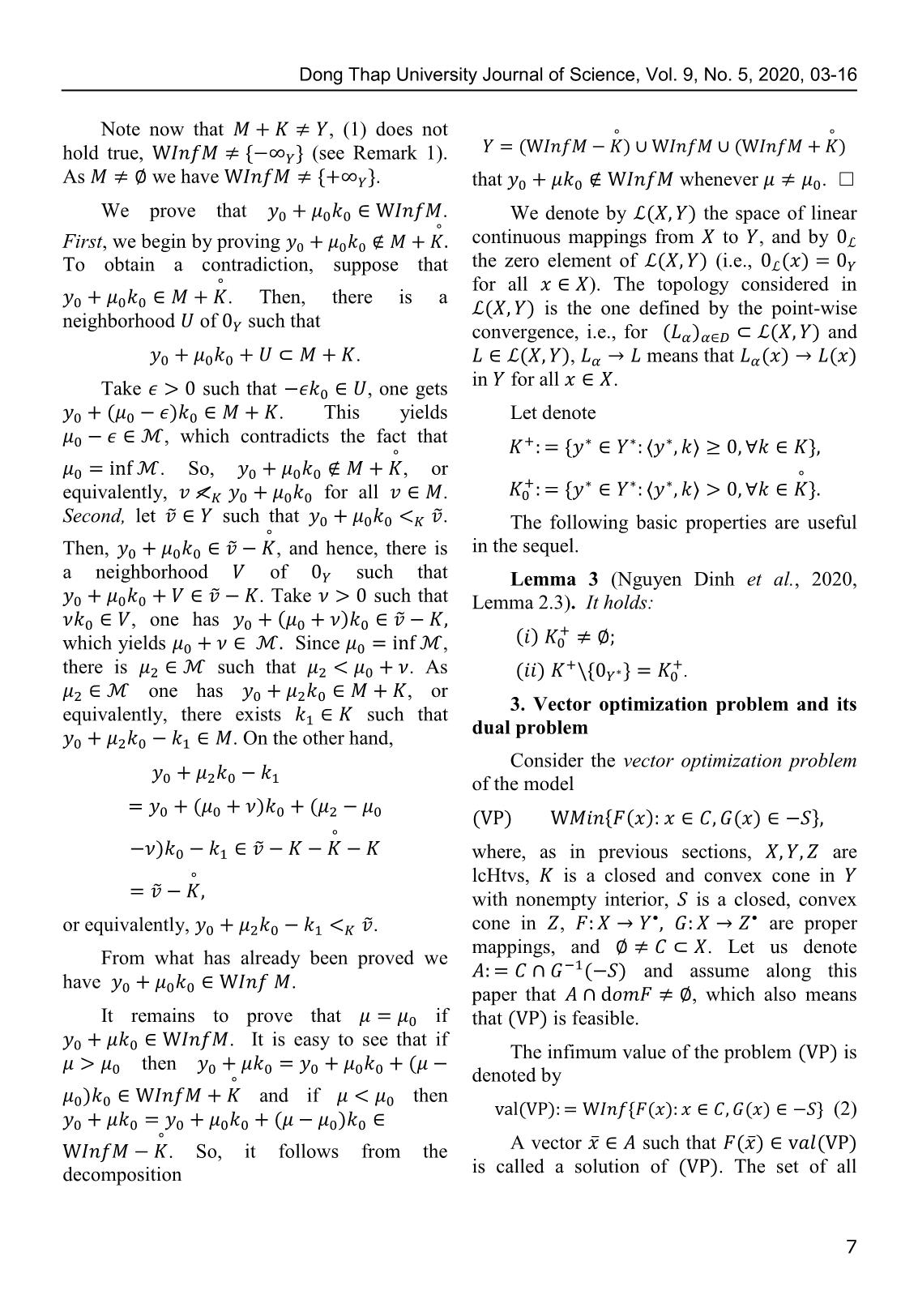
Trang 5
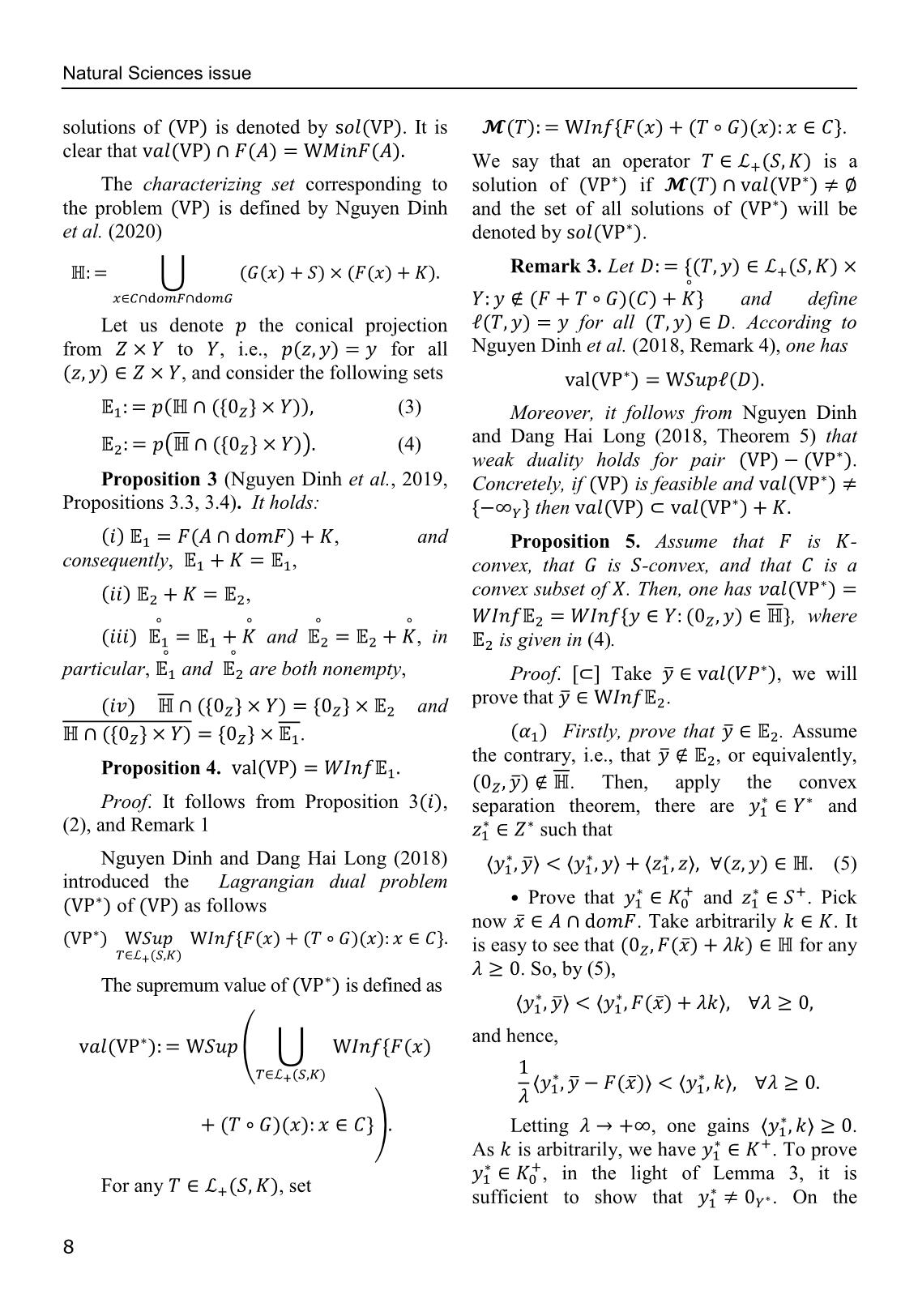
Trang 6
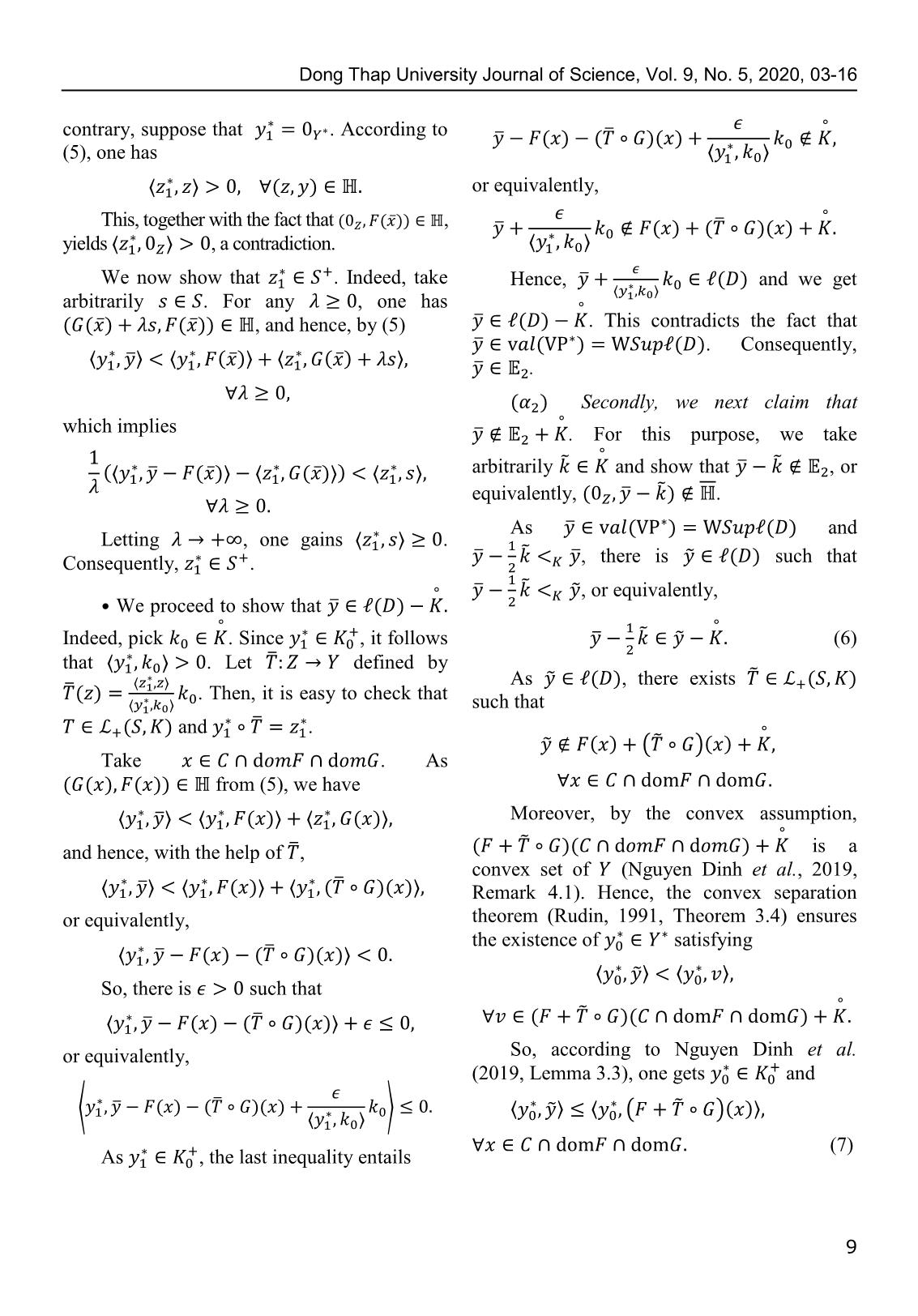
Trang 7
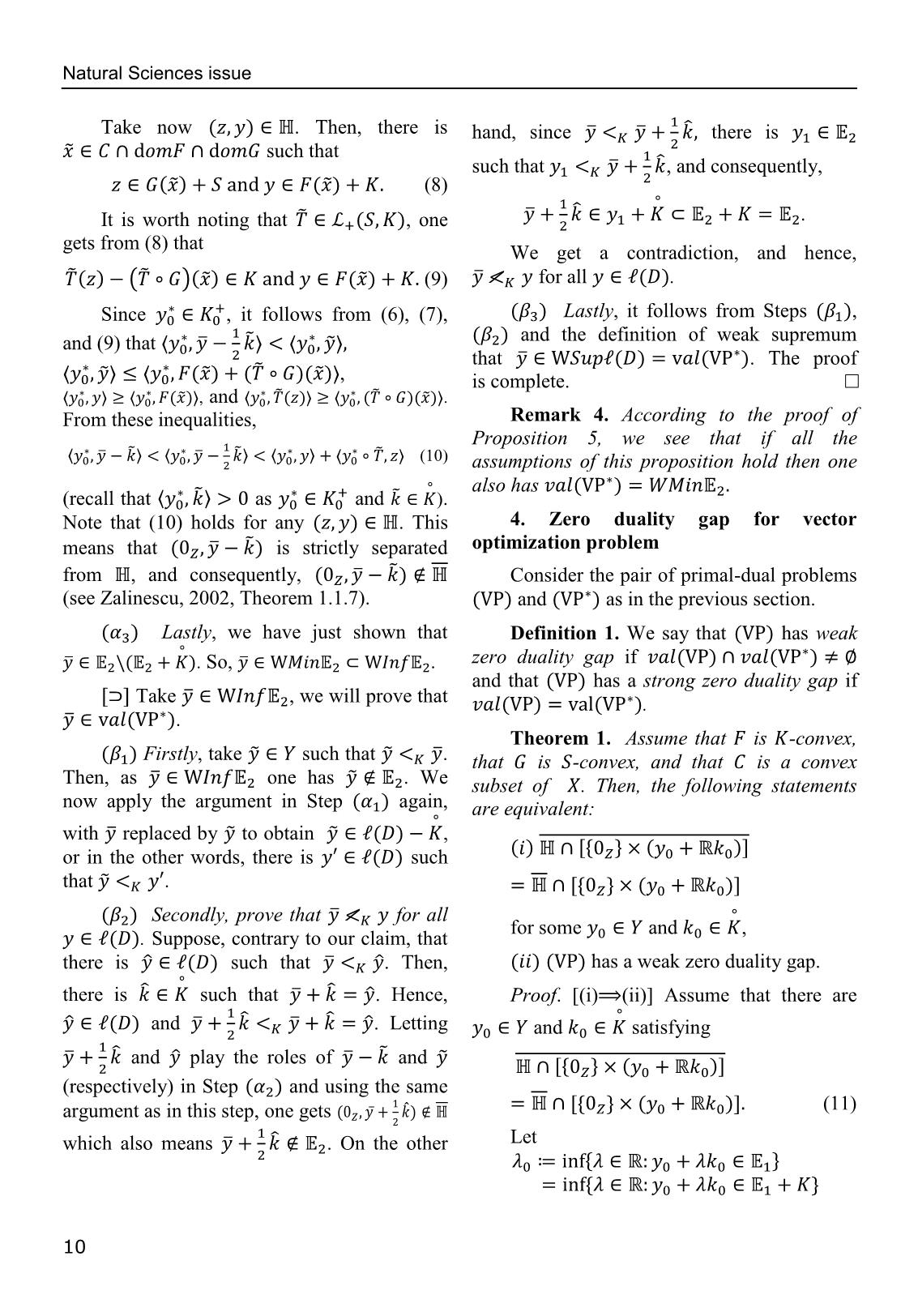
Trang 8
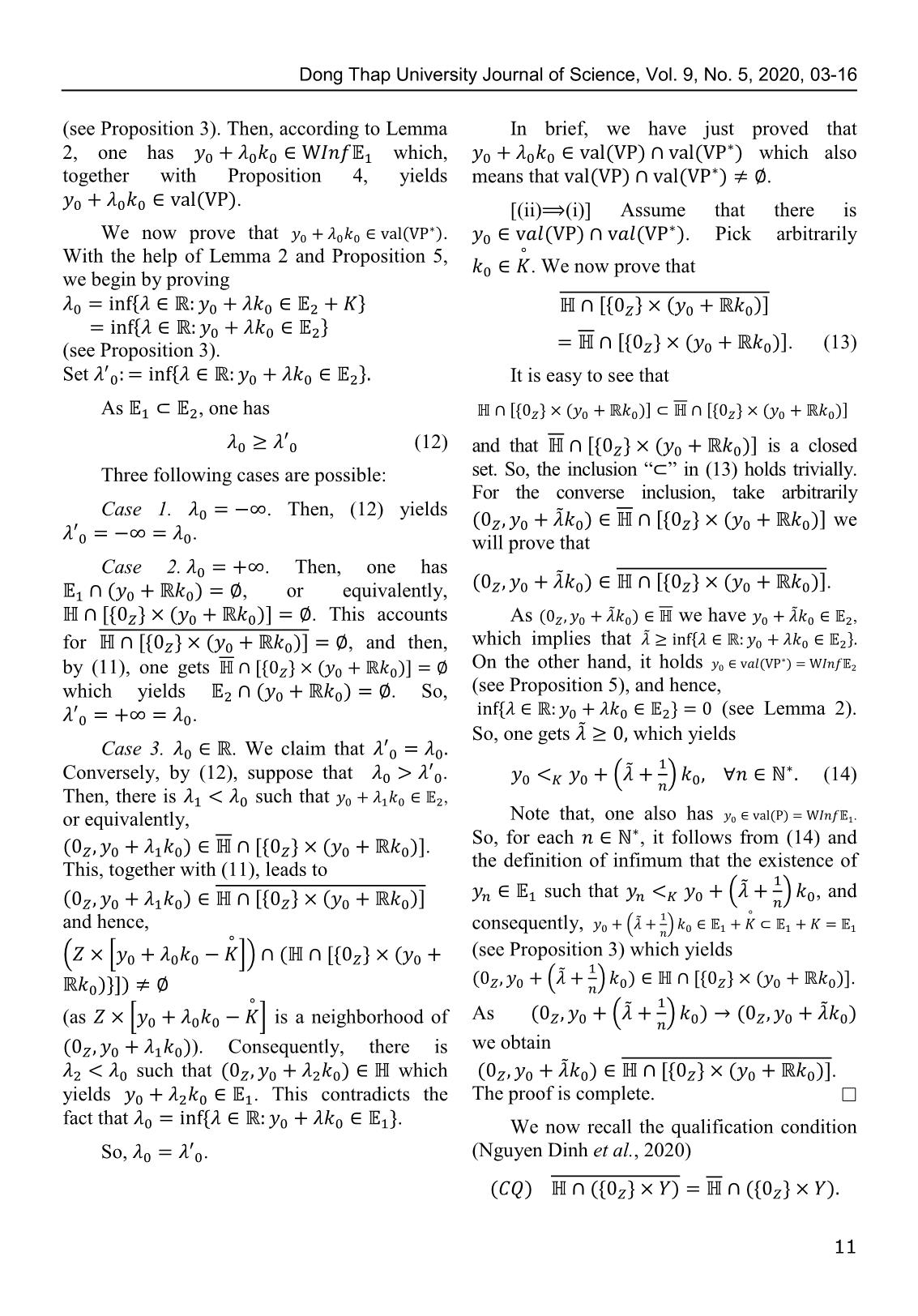
Trang 9
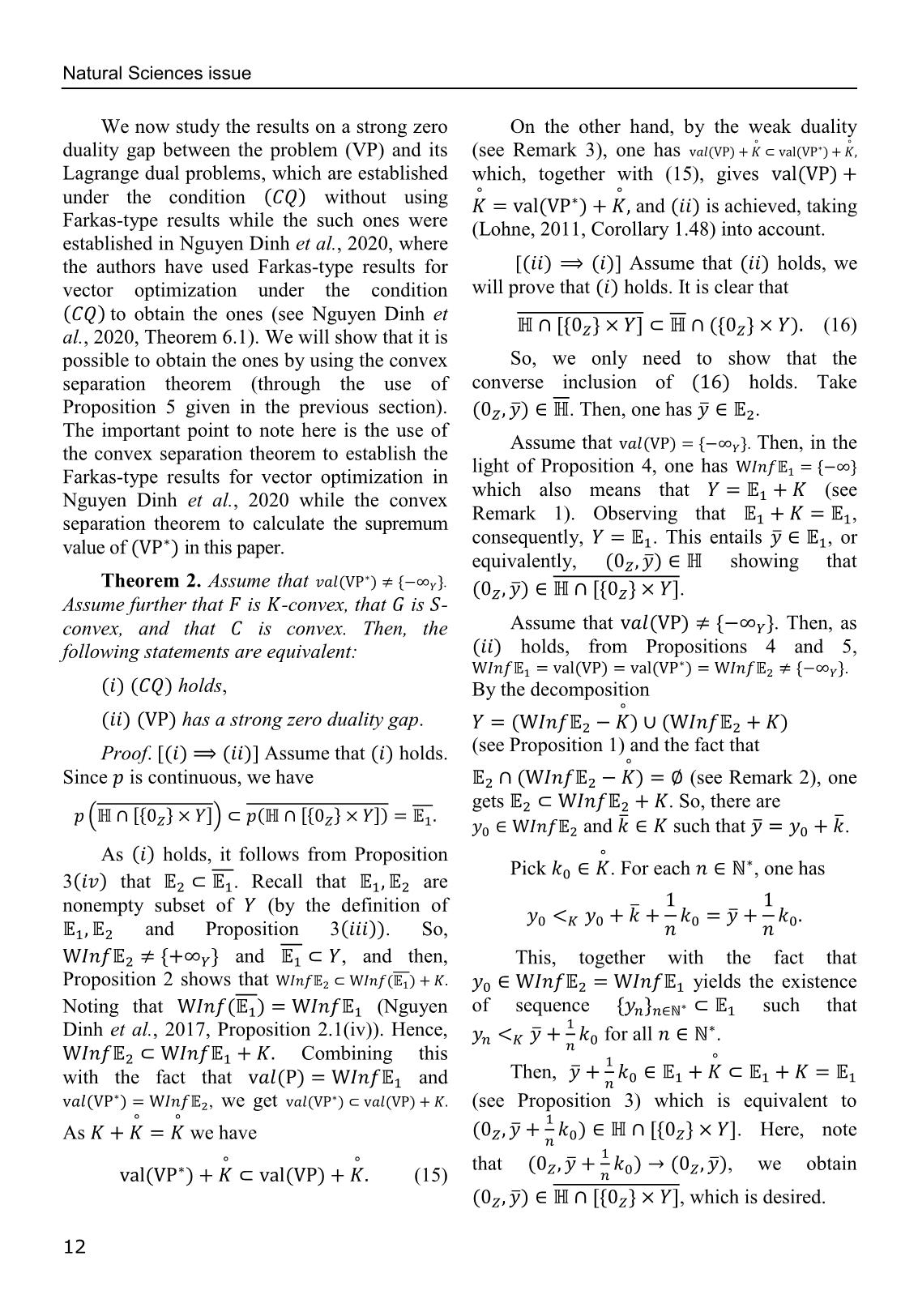
Trang 10
Tải về để xem bản đầy đủ
Bạn đang xem 10 trang mẫu của tài liệu "Một cách tiếp cận mới cho khoảng cách đối ngẫu bằng không của bài toán tối ưu véctơ sử dụng tập đặc trưng", để tải tài liệu gốc về máy hãy click vào nút Download ở trên
Tóm tắt nội dung tài liệu: Một cách tiếp cận mới cho khoảng cách đối ngẫu bằng không của bài toán tối ưu véctơ sử dụng tập đặc trưng
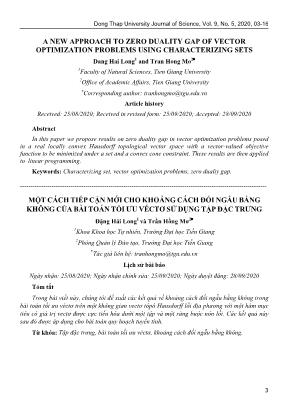
, a contradiction. We now show that . Indeed, take Hence, ̅ and we get arbitrarily . For any , one has ̅ ̅ , and hence, by (5) ̅ . This contradicts the fact that ̅ . Consequently, ̅ ̅ ̅ ̅ . Secondly, we next claim that which implies ̅ . For this purpose, we take arbitrarily ̃ and show that ̅ ̃ , or ̅ ̅ ̅ equivalently, ̅ ̃ . As ̅ and Letting , one gains . ̃ ̅ ̅, there is ̃ such that Consequently, . ̅ ̃ ̃, or equivalently, We proceed to show that ̅ ̃ Indeed, pick . Since , it follows ̅ ̃ (6) that . Let ̅ defined by As ̃ , there exists ̃ ̅ . Then, it is easy to check that such that and ̅ . ̃ ( ̃ ) Take . As from (5), we have ̅ Moreover, by the convex assumption, ̃ and hence, with the help of ̅, is a convex set of (Nguyen Dinh et al., 2019, ̅ ̅ Remark 4.1). Hence, the convex separation or equivalently, theorem (Rudin, 1991, Theorem 3.4) ensures the existence of satisfying ̅ ̅ ̃ So, there is such that ̃ ̅ ̅ or equivalently, So, according to Nguyen Dinh et al. (2019, Lemma 3.3), one gets and ̅ ⟨ ̅ ⟩ ̃ ( ̃ ) (7) As , the last inequality entails 9 Natural Sciences issue Take now . Then, there is ̂ hand, since ̅ ̅ there is ̃ such that ̂ such that ̅ , and consequently, ̃ ̃ (8) ̅ ̂ . It is worth noting that ̃ , one gets from (8) that We get a contradiction, and hence, ̃ ( ̃ ) ̃ ̃ (9) ̅ for all . Since , it follows from (6), (7), Lastly, it follows from Steps , and (9) that ̅ ̃ ̃ and the definition of weak supremum that ̅ . The proof ̃ ̃ ̃ ̃ , is complete. ̃ , and ̃ ̃ ̃ . From these inequalities, Remark 4. According to the proof of Proposition 5, we see that if all the ̅ ̃ ̅ ̃ ̃ (10) assumptions of this proposition hold then one ̃ ̃ also has (recall that as and ). Note that (10) holds for any . This 4. Zero duality gap for vector ̃ optimization problem means that ̅ is strictly separated ̃ from , and consequently, ̅ Consider the pair of primal-dual problems (see Zalinescu, 2002, Theorem 1.1.7). and as in the previous section. Lastly, we have just shown that Definition 1. We say that has weak zero duality gap if ̅ . So, ̅ . and that has a strong zero duality gap if Take ̅ , we will prove that . ̅ . Theorem 1. Assume that is -convex, Firstly, take ̃ such that ̃ ̅. that is -convex, and that is a convex Then, as ̅ one has ̃ . We subset of . Then, the following statements now apply the argument in Step again, are equivalent: with ̅ replaced by ̃ to obtain ̃ , or in the other words, there is such { } that ̃ . { } Secondly, prove that ̅ for all for some and , . Suppose, contrary to our claim, that there is ̂ such that ̅ ̂. Then, has a weak zero duality gap. there is ̂ such that ̅ ̂ ̂. Hence, Proof. [(i) (ii)] Assume that there are ̂ and ̅ ̂ ̅ ̂ ̂. Letting and satisfying ̅ ̂ and ̂ play the roles of ̅ ̃ and ̃ { } (respectively) in Step and using the same argument as in this step, one gets ̅ ̂ { } (11) which also means ̅ ̂ . On the other Let { } { } 10 Dong Thap University Journal of Science, Vol. 9, No. 5, 2020, 03-16 (see Proposition 3). Then, according to Lemma In brief, we have just proved that 2, one has which, which also together with Proposition 4, yields means that . . [(ii) (i)] Assume that there is We now prove that . . Pick arbitrarily With the help of Lemma 2 and Proposition 5, . We now prove that we begin by proving { } { } { } (see Proposition 3). { } . (13) Set { } It is easy to see that As , one has { } { } (12) and that { } is a closed Three following cases are possible: set. So, the inclusion “ ” in (13) holds trivially. For the converse inclusion, take arbitrarily Case 1. . Then, (12) yields ̃ { } we . will prove that Case 2. . Then, one has ̃ { } . , or equivalently, ̃ ̃ { } . This accounts As we have , ̃ { } for { } , and then, which implies that On the other hand, it holds by (11), one gets { } (see Proposition 5), and hence, which yields . So, { } (see Lemma 2). . So, one gets ̃ which yields Case 3. . We claim that Conversely, by (12), suppose that . ( ̃ ) (14) Then, there is such that , or equivalently, Note that, one also has . So, for each , it follows from (14) and { } . This, together with (11), leads to the definition of infimum that the existence of ̃ such that ( ) , and { } and hence, consequently, ( ̃ ) (see Proposition 3) which yields ( * +) { } ( ̃ ) { } . } As ( ̃ ) ̃ (as * + is a neighborhood of ). Consequently, there is we obtain ̃ such that which { } . yields . This contradicts the The proof is complete. fact that { }. We now recall the qualification condition So, . (Nguyen Dinh et al., 2020) { } { } 11 Natural Sciences issue We now study the results on a strong zero On the other hand, by the weak duality duality gap between the problem (VP) and its (see Remark 3), one has Lagrange dual problems, which are established which, together with (15), gives under the condition without using and is achieved, taking Farkas-type results while the such ones were (Lohne, 2011, Corollary 1.48) into account. established in Nguyen Dinh et al., 2020, where the authors have used Farkas-type results for [ ] Assume that holds, we vector optimization under the condition will prove that holds. It is clear that to obtain the ones (see Nguyen Dinh et { } { } (16) al., 2020, Theorem 6.1). We will show that it is possible to obtain the ones by using the convex So, we only need to show that the separation theorem (through the use of converse inclusion of holds. Take Proposition 5 given in the previous section). ̅ . Then, one has ̅ . The important point to note here is the use of Assume that { }. Then, in the the convex separation theorem to establish the light of Proposition 4, one has { } Farkas-type results for vector optimization in which also means that (see Nguyen Dinh et al., 2020 while the convex separation theorem to calculate the supremum Remark 1). Observing that , consequently, . This entails ̅ , or value of in this paper. equivalently, ̅ showing that Theorem 2. Assume that { }. ̅ { } . Assume further that is -convex, that is - convex, and that is convex. Then, the Assume that { }. Then, as following statements are equivalent: holds, from Propositions 4 and 5, { }. holds, By the decomposition has a strong zero duality gap. (see Proposition 1) and the fact that Proof. [ ] Assume that holds. Since is continuous, we have (see Remark 2), one gets . So, there are ( { } ) { } ̅ ̅ and such that ̅ . As holds, it follows from Proposition Pick . For each , one has 3 that . Recall that are nonempty subset of (by the definition of ̅ ̅ and Proposition 3 . So, { } and , and then, This, together with the fact that Proposition 2 shows that yields the existence Noting that (Nguyen of sequence { } such that Dinh et al., 2017, Proposition 2.1(iv)). Hence, ̅ for all . Combining this Then, ̅ with the fact that and , we get (see Proposition 3) which is equivalent to ̅ { } . Here, note As we have that ̅ ̅ , we obtain (15) ̅ { } , which is desired. 12 Dong Thap University Journal of Science, Vol. 9, No. 5, 2020, 03-16 Remark 5. It is worth mentioning that when we take , K , , { } the problem collapses to the problem in Pham Duy Khanh et al. { } (2019). Then, the result on strong duality for the { } { } problem in Pham Duy Khanh et al. { } (2019, Theorem 4.3) follows from Theorem 2. { }, and Let us now introduce the second qualification condition, saying that is closed regarding the set { } concretely, { } { } { } { } Theorem 3. Assume that the problem { } is feasible and { }. It is clear that the converse implication in Assume further that is -convex, that is - Theorem 3 does not hold. convex, and that is a convex set of . If the 5. A special case: Linear programming condition holds then the problem has a strong zero duality gap. In the this section, as an illustrate example for the results established above, we consider a Proof. According to Proposition 4 and special case of the problem (VP), that is the Proposition 5, we have linear programming: and . As holds, one finds that . Consequently, one has . where , , and . The following example shows that the Observing that the problem collapses to converse implication in Theorem 3 does not hold. the problem when we take , K , , . Example 1 Let Then, the corresponding characterizing set of and . Let is and be such that and for all . It is easy to { } see that is -convex, that is -convex, and The qualification condition now is is convex. In this case, we have { } { } ⋃ Recall that the Lagrange dual problem of denoted by is ⋃ It is worth mentioning that the problem By some calculations, we obtain is a special case of the linear programming problem (IP) in Anderson (1983) { } { } { and the problem (ILP) in Pham Duy Khanh et } al. (2019) where . The duality for the { } { } { problem was considered in Anderson } (1983) under the closedness conditions. Recently, Pham Duy Khanh et al. (2019) had On the other hand, 13 Natural Sciences issue studied the duality for the problem under (i) holds, some necessary and sufficient conditions. (ii) We now introduce a new type of dual Proof. Firstly, by Proposition 6, is problem of called the sequential dual equivalent to The problem as follows: conclusion now follows from Theorem 2. [ ] We next introduce a sufficient condition, which ensures the fulfillment of the condition , and then, leads to the results on zero The relations between the values of the duality gap for the pairs problem and its dual problems are given by the following proposition. Proposition 7. Assume that there are Proposition 6. It holds: and such that . (14) Proof. (15) Prove that : It is easy to see that Then, holds. { } Proof. It is sufficient to prove that { } { } . To do { } this, take . We will show that where { } . Indeed, since { , it follows that there exists a net } such that { (16) } . (17) Obviously, . So, . Prove that : Take Assume that there are and such that (14) and (15) holds. This, together and such that with (17), leads to the fact that Then, for all , and hence, for all . , Since and , it follows from or, . the above inequality that The desired inequality follows from the This, together with the last one of (15) and definition of the problems and . (14), one gets The next result extends (Pham Duy Khanh et al., 2019, Theorem 4.3) in the case when taking Corollary 3. The following statements are equivalent: or equivalently, . From this and the first one of (15), we obtain 14 Dong Thap University Journal of Science, Vol. 9, No. 5, 2020, 03-16 { } , References and hence, { } Anderson, E.J. (1983). A review of duality as desired. theory for linear programming over The next result is a direct consequence of topological vector spaces. J. Math. Anal. Proposition 7 and Corollary 3. Appl., 97(2), 380-392. Corollary 4. Assume all the assumptions Andreas, L. (2011). Vector optimization with of Proposition 7 hold. Then, one has infimum and supremum. Berlin: Springer- Verlag. Bot, R.I. (2010). Conjugate duality in convex Corollary 5. Assume that the following optimization. Berlin: Springer. conditions hold: Bot, R.I., Grad, S.M., and Wanka, G. (2009): Duality in Vector Optimization. Berlin: The problem is feasible, i.e., Springer-Verlag. there is such that . Canovas, M.J., Nguyen Dinh, Dang Hai Long, . and Parra, J. (2020). A new approach to strong duality for composite vector Then, optimization problems. Optimization. [10.1080/02331934.2020.1745796]. Proof. The fulfillment of means that there is such that (14). As holds, Elvira, H., Andreas, L., Luis, R., and Tammer, there exists such that . This C. (2013). Lagrange duality, stability and leads to the fact that (15) holds. The subdifferentials in vector optimization. conclusion now follows from Corollary 4. Optimization , 62(3), 415-428. Jeyakumar, V. and Volkowicz, H. (1990). Zero Corollary 6. Assume that and one of duality gap in infinite-dimensional the following condition holds: programming. J. Optim. Theory Appl., 67(1), 88-108. . Khan, A., Tammer, C., and Zalinescu, C. is a surjection. (2005). Set-valued optimization: An Then, introduction with applications. Heidelberg: Springer. Proof. It is easy to see that if at least one Nguyen Dinh, Dang Hai Long, Tran Hong Mo, of the conditions and holds then and Yao, J.-C. (2020). Approximate holds as well. So, Corollary 6 is a consequence Farkas lemmas for vector systems with of Corollary 5. applications to convex vector optimization Acknowledgements: The work is problems. J. Non. Con. Anal., 21(5), supported, in part, by the national budget of 1225-1246. Tien Giang town, under the project “Necessary Nguyen Dinh and Dang Hai Long. (2018). and sufficient conditions for duality in vector Complete characterizations of robust optimization and applications ”, Tien Giang, strong duality for robust vector Vietnam./. optimization problems. Vietnam J. Math., 46(2), 293-328. 15 Natural Sciences issue Nguyen Dinh, Goberna, M.A., Lopez, M.A., sufficient conditions for qualitative and Tran Hong Mo. (2017). Farkas-type properties of infinite dimensional linear results for vector-valued functions with programming problems. Numer. Func. applications. J. Optim. Theory Appl., Anal. Opt., 40(8), 924-943. 173(2), 357-390. Rudin, W. (1991). Functional analysis (2nd Nguyen Dinh, Goberna, M.A., Dang Hai Long, Edition). New York: McGraw-Hill. and Lopez, M.A. (2019). New Farkas-type Tanino, T. (1992). Conjugate duality in vector results for vector-valued functions: A non- optimization. J. Math. Anal. Appl., 167(1), abstract approach. J. Optim. Theory Appl., 84-97. 82(1), 4-29. Wen, S. (1998). Duality in set-valued Nguyen Thi Vinh, Kim, D.S., Nguyen Nang optimization. Warszawa: Instytut Tam, and Nguyen Dong Yen. (2016). Matematyczny Polskiej Akademi Nauk. Duality gap function in infinite dimensional linear programming. J. Math. Zalinescu, C. (2002). Convex analysis in Anal. Appl., 437(1), 1-15. general vector spaces. Singapore: World Scientificc Publishing. Pham Duy Khanh, Tran Hong Mo, and Tran Thi Tu Trinh. (2019). Necessary and 16
File đính kèm:
mot_cach_tiep_can_moi_cho_khoang_cach_doi_ngau_bang_khong_cu.pdf