Improving TDWZ correlation noise estimation: A deep learning based approach
Transform domain Wyner-Ziv video coding (TDWZ) has shown its benefits in compressing video applications with limited resources such as visual surveillance systems, remote sensing and wireless sensor networks. In TDWZ, the correlation noise model (CNM) plays a vital role since it directly affects to the number of bits needed to send from the encoder and thus the overall TDWZ compression performance. To achieve CNM with high accurate for TDWZ, we propose in this paper a novel CNM estimation approach in which the CNM with Laplacian distribution is adaptively estimated based on a deep learning (DL) mechanism. The proposed DL based CNM includes two hidden layers and a linear activation function to adaptively update the Laplacian parameter. Experimental results showed that the proposed TDWZ codec significantly outperforms the relevant benchmarks, notably by around 35% bitrate saving when compared to the DISCOVER codec and around 22% bitrate saving when compared to the HEVC Intra benchmark while providing a similar perceptual quality
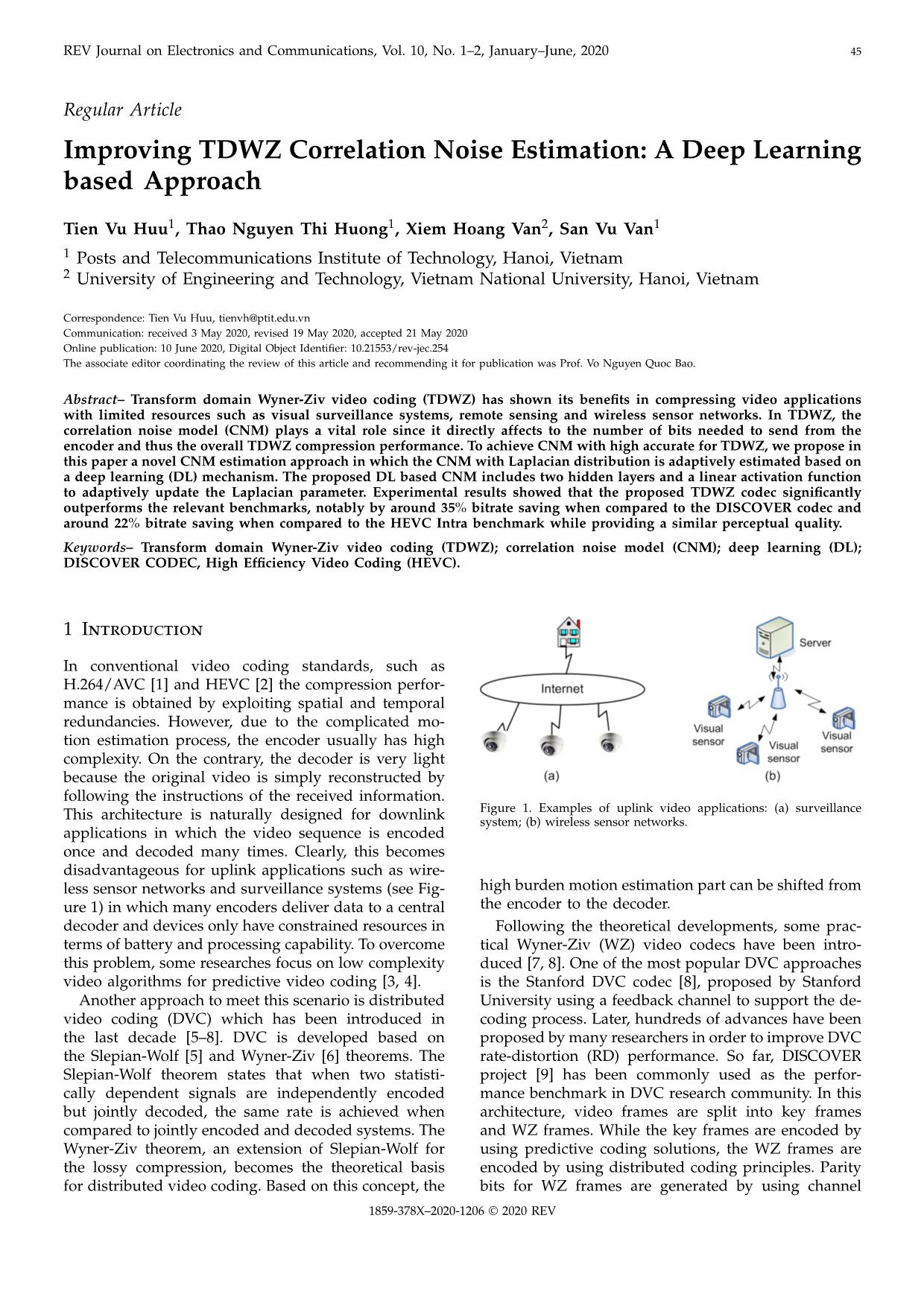
Trang 1
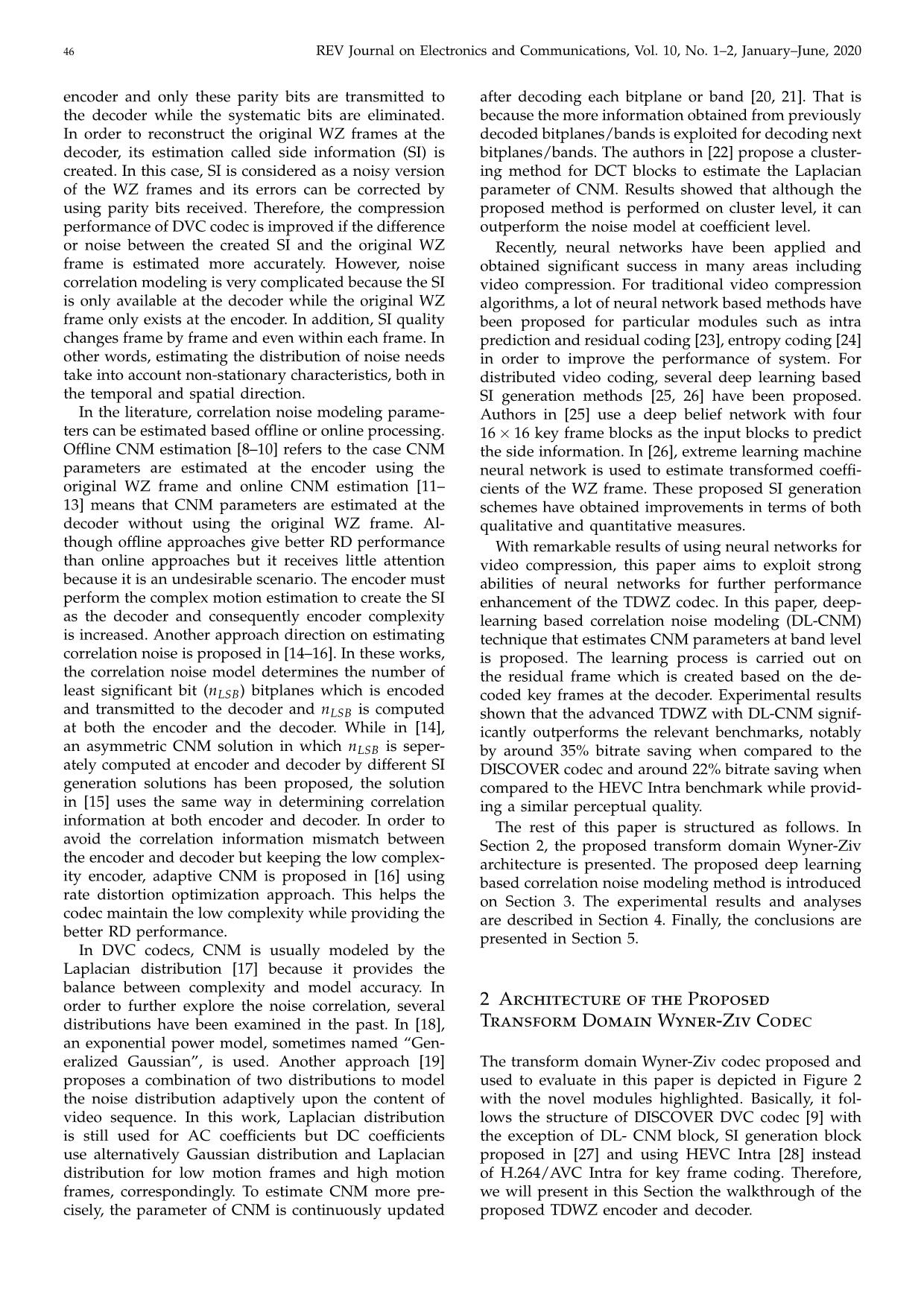
Trang 2
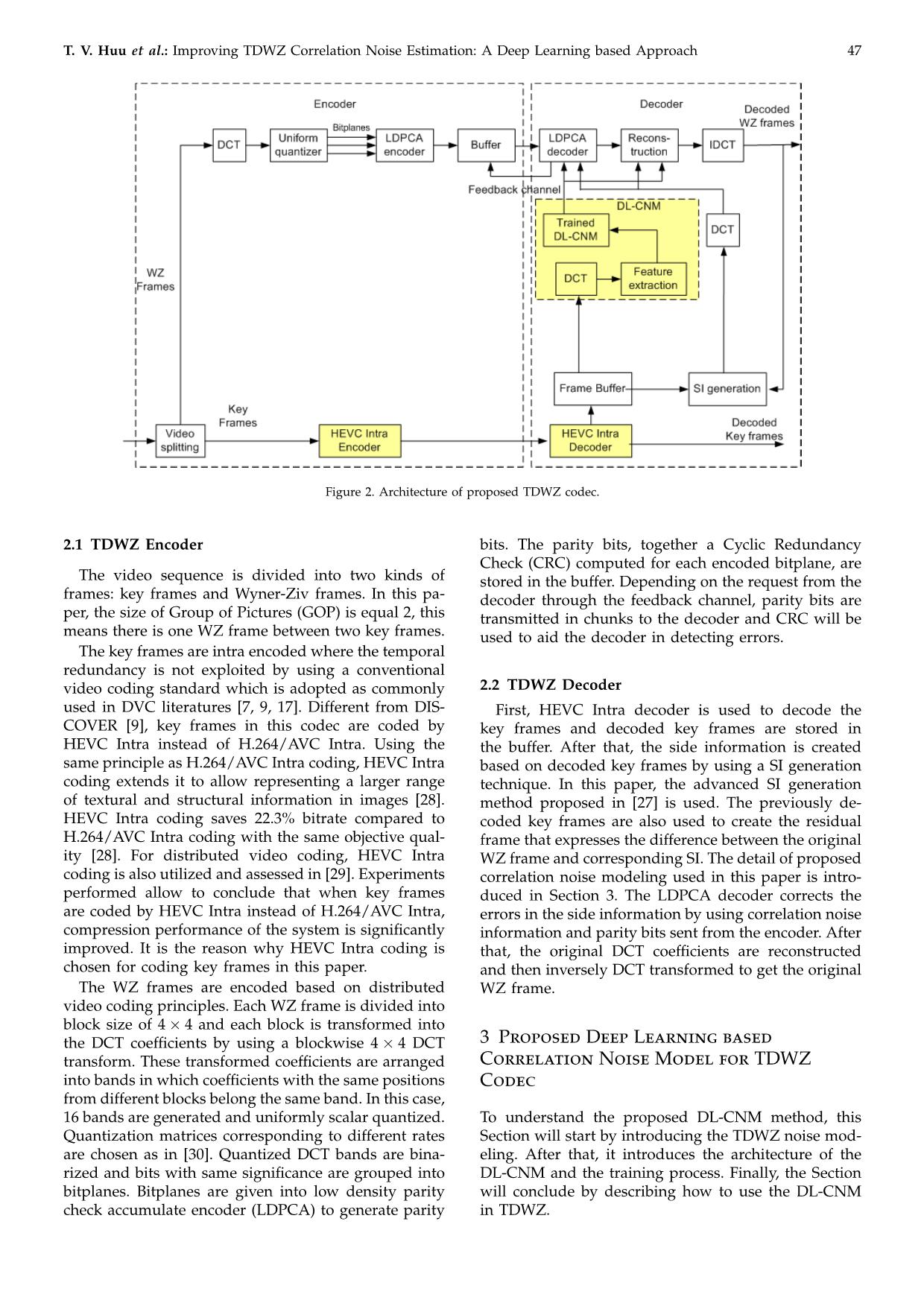
Trang 3
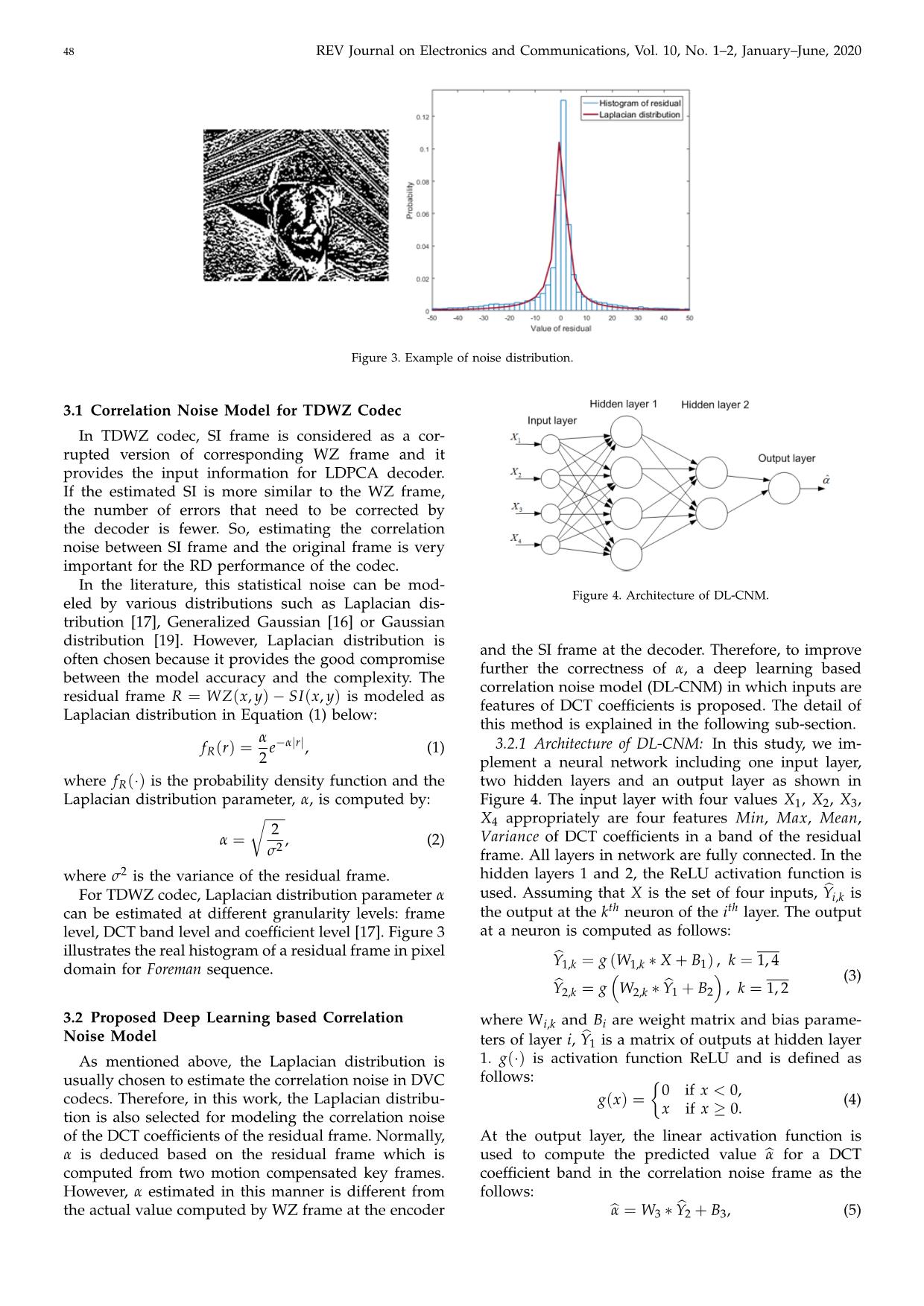
Trang 4
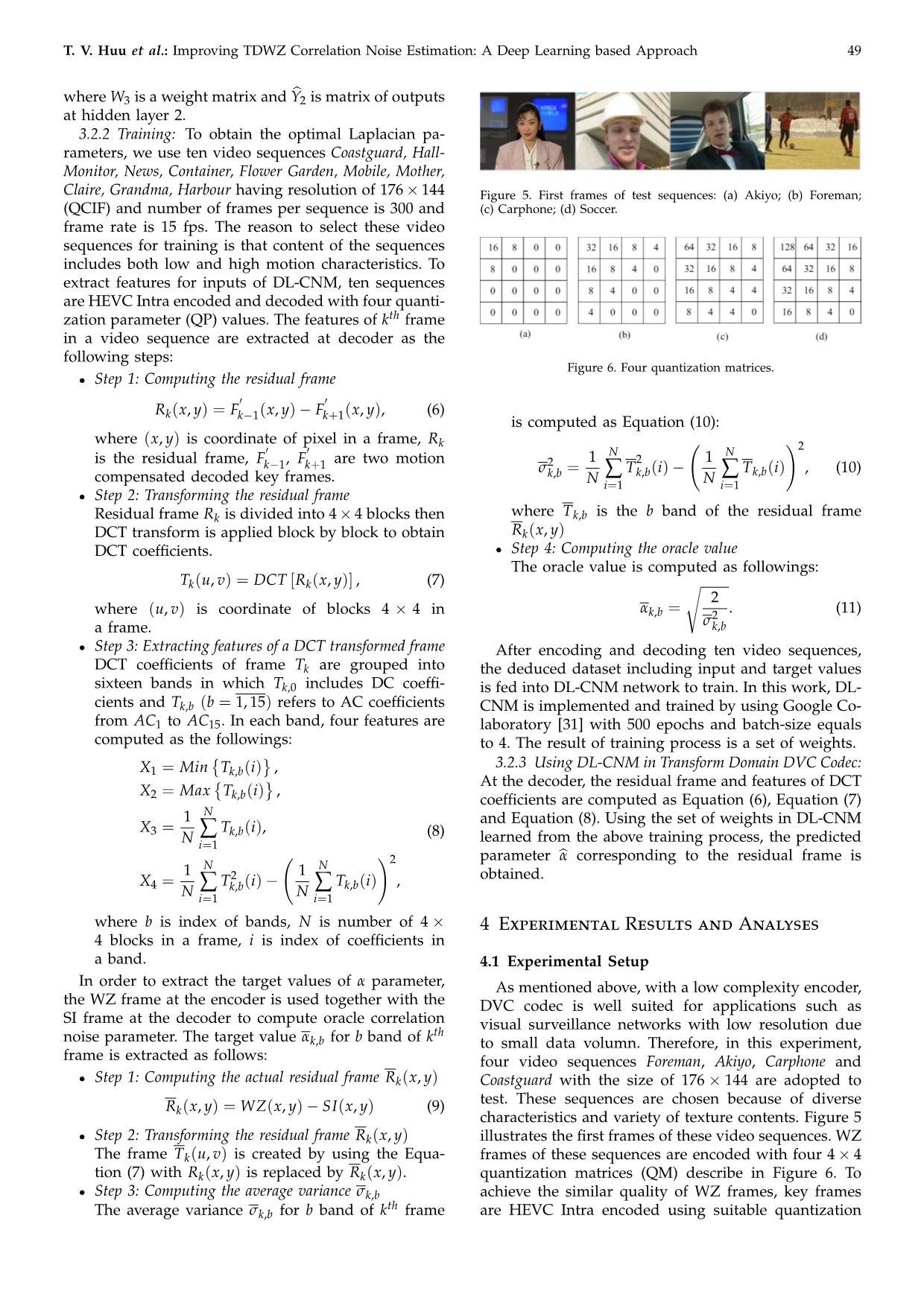
Trang 5
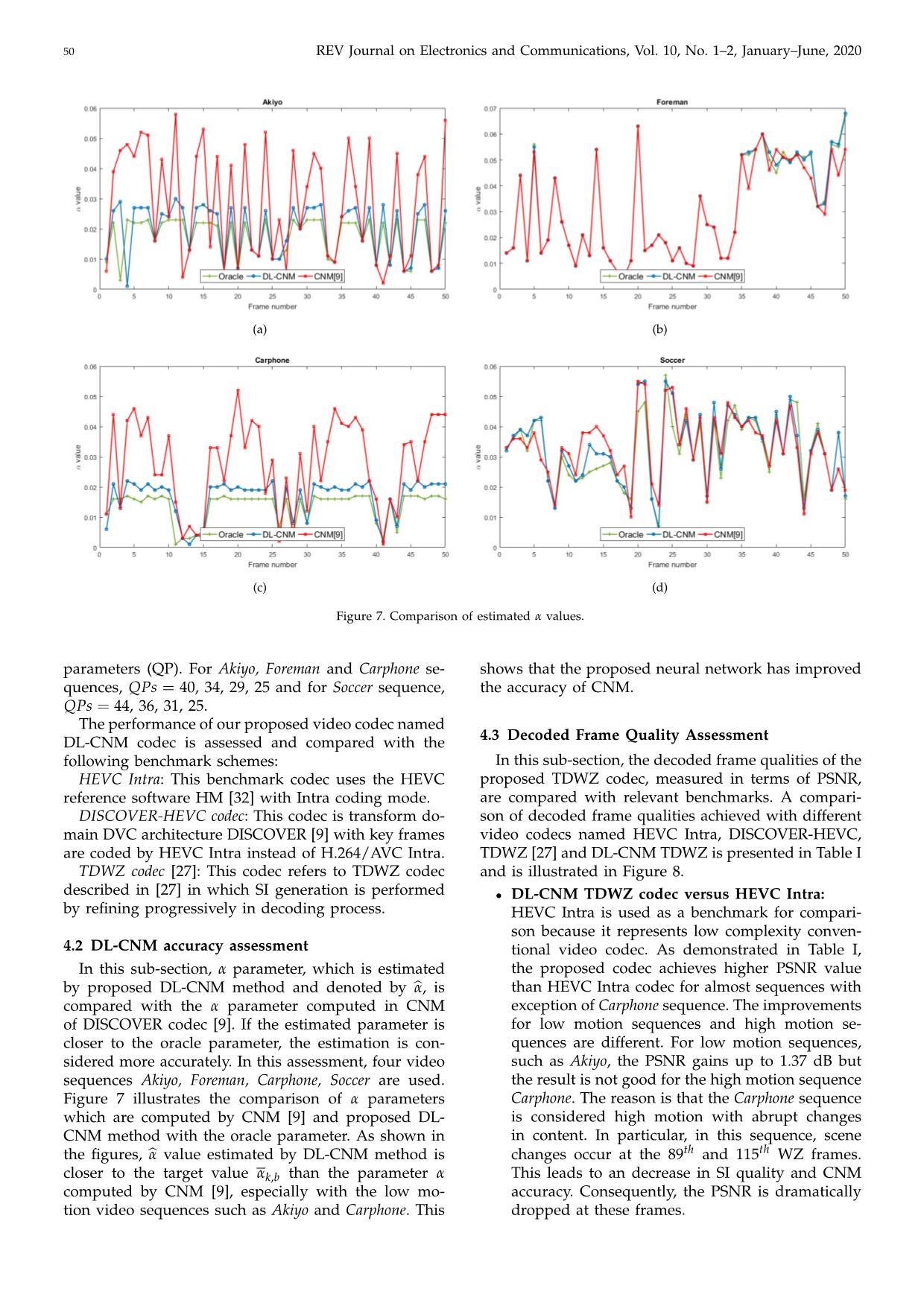
Trang 6
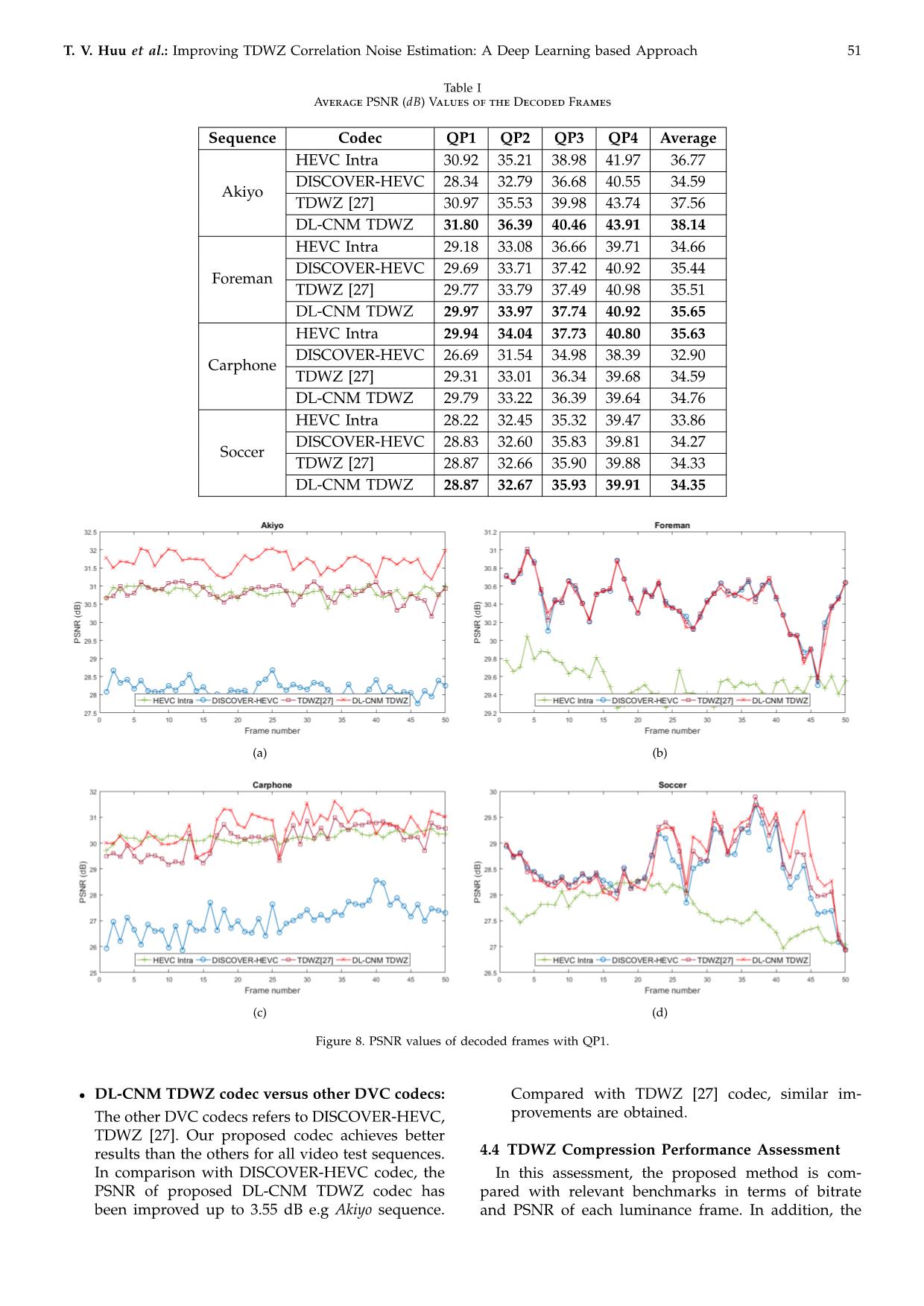
Trang 7
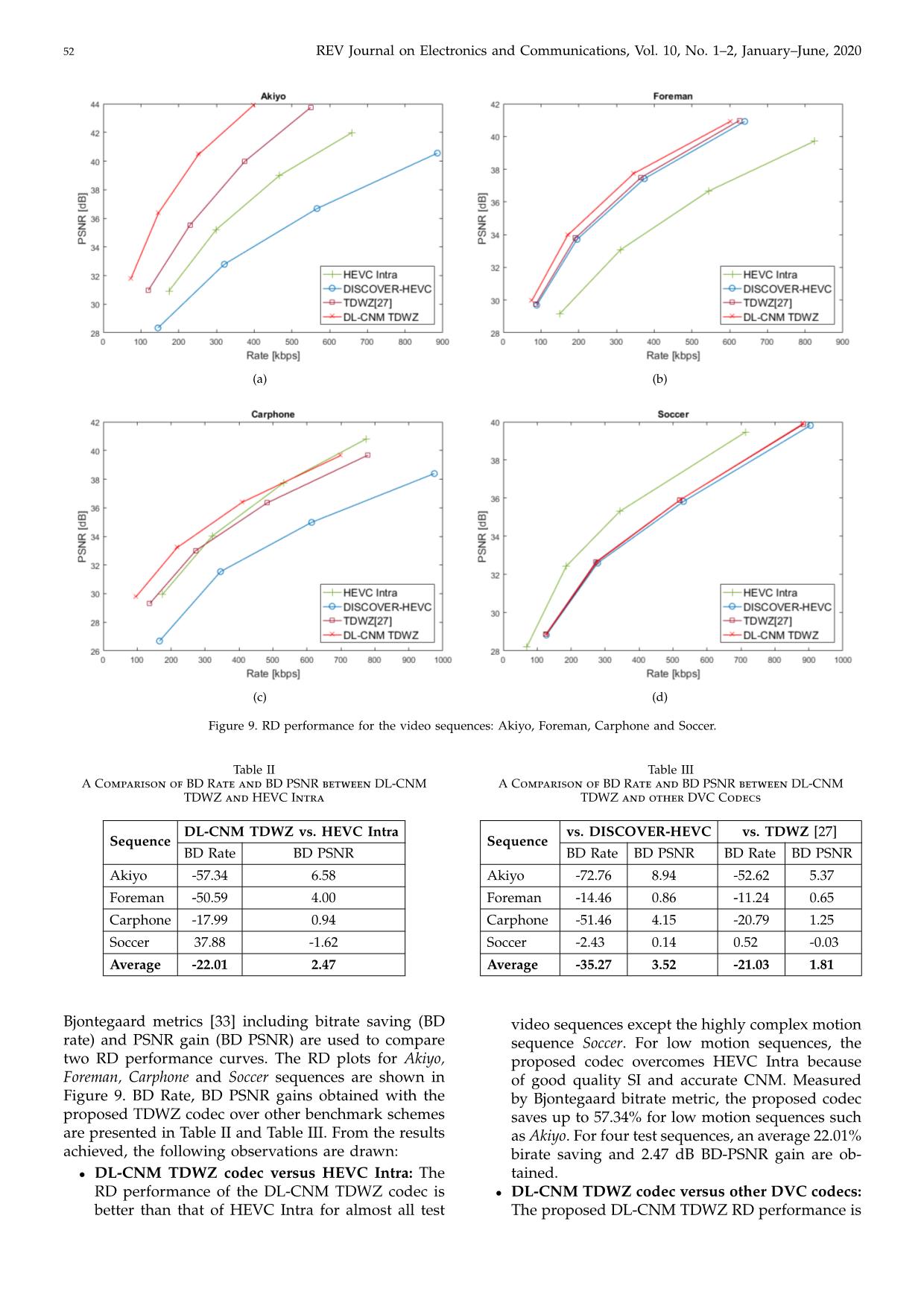
Trang 8
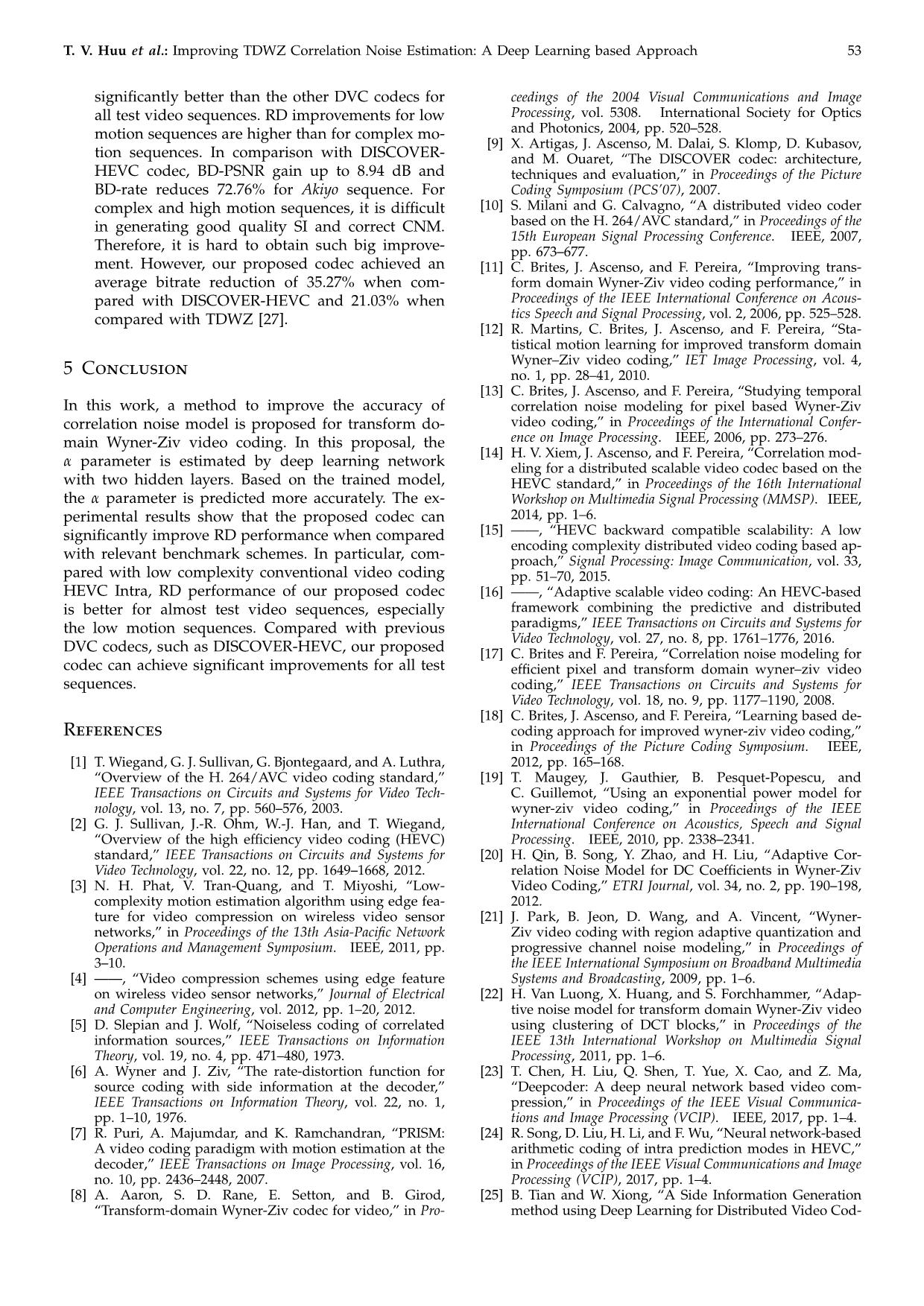
Trang 9
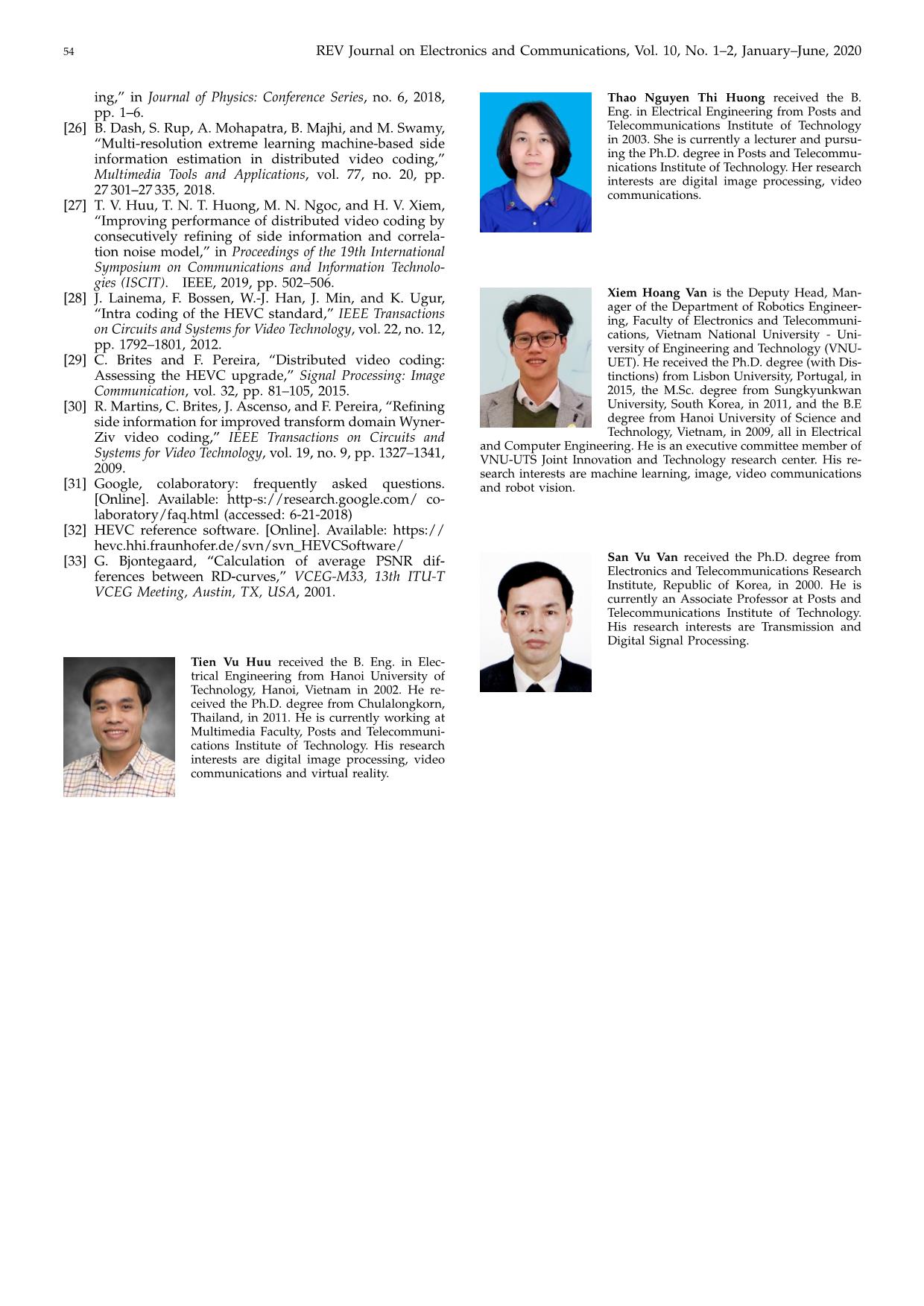
Trang 10
Tóm tắt nội dung tài liệu: Improving TDWZ correlation noise estimation: A deep learning based approach
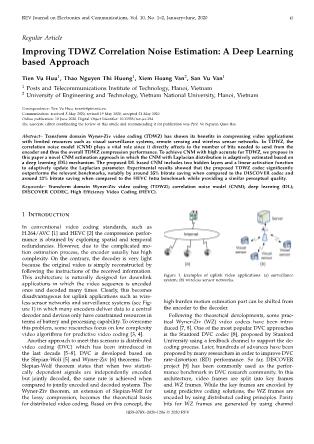
e α parameter computed in CNM exception of Carphone sequence. The improvements of DISCOVER codec [9]. If the estimated parameter is for low motion sequences and high motion se- closer to the oracle parameter, the estimation is con- quences are different. For low motion sequences, sidered more accurately. In this assessment, four video such as Akiyo, the PSNR gains up to 1.37 dB but sequences Akiyo, Foreman, Carphone, Soccer are used. the result is not good for the high motion sequence Figure 7 illustrates the comparison of α parameters Carphone. The reason is that the Carphone sequence which are computed by CNM [9] and proposed DL- is considered high motion with abrupt changes CNM method with the oracle parameter. As shown in in content. In particular, in this sequence, scene th th the figures, bα value estimated by DL-CNM method is changes occur at the 89 and 115 WZ frames. closer to the target value αk,b than the parameter α This leads to an decrease in SI quality and CNM computed by CNM [9], especially with the low mo- accuracy. Consequently, the PSNR is dramatically tion video sequences such as Akiyo and Carphone. This dropped at these frames. T. V. Huu et al.: Improving TDWZ Correlation Noise Estimation: A Deep Learning based Approach 51 Table I Average PSNR (dB)Values of the Decoded Frames Sequence Codec QP1 QP2 QP3 QP4 Average HEVC Intra 30.92 35.21 38.98 41.97 36.77 DISCOVER-HEVC 28.34 32.79 36.68 40.55 34.59 Akiyo TDWZ [27] 30.97 35.53 39.98 43.74 37.56 DL-CNM TDWZ 31.80 36.39 40.46 43.91 38.14 HEVC Intra 29.18 33.08 36.66 39.71 34.66 DISCOVER-HEVC 29.69 33.71 37.42 40.92 35.44 Foreman TDWZ [27] 29.77 33.79 37.49 40.98 35.51 DL-CNM TDWZ 29.97 33.97 37.74 40.92 35.65 HEVC Intra 29.94 34.04 37.73 40.80 35.63 DISCOVER-HEVC 26.69 31.54 34.98 38.39 32.90 Carphone TDWZ [27] 29.31 33.01 36.34 39.68 34.59 DL-CNM TDWZ 29.79 33.22 36.39 39.64 34.76 HEVC Intra 28.22 32.45 35.32 39.47 33.86 DISCOVER-HEVC 28.83 32.60 35.83 39.81 34.27 Soccer TDWZ [27] 28.87 32.66 35.90 39.88 34.33 DL-CNM TDWZ 28.87 32.67 35.93 39.91 34.35 (a) (b) (c) (d) Figure 8. PSNR values of decoded frames with QP1. • DL-CNM TDWZ codec versus other DVC codecs: Compared with TDWZ [27] codec, similar im- The other DVC codecs refers to DISCOVER-HEVC, provements are obtained. TDWZ [27]. Our proposed codec achieves better results than the others for all video test sequences. 4.4 TDWZ Compression Performance Assessment In comparison with DISCOVER-HEVC codec, the In this assessment, the proposed method is com- PSNR of proposed DL-CNM TDWZ codec has pared with relevant benchmarks in terms of bitrate been improved up to 3.55 dB e.g Akiyo sequence. and PSNR of each luminance frame. In addition, the 52 REV Journal on Electronics and Communications, Vol. 10, No. 1–2, January–June, 2020 (a) (b) (c) (d) Figure 9. RD performance for the video sequences: Akiyo, Foreman, Carphone and Soccer. Table II Table III AComparison of BD Rate and BD PSNR between DL-CNM AComparison of BD Rate and BD PSNR between DL-CNM TDWZ and HEVC Intra TDWZ and other DVC Codecs DL-CNM TDWZ vs. HEVC Intra vs. DISCOVER-HEVC vs. TDWZ [27] Sequence Sequence BD Rate BD PSNR BD Rate BD PSNR BD Rate BD PSNR Akiyo -57.34 6.58 Akiyo -72.76 8.94 -52.62 5.37 Foreman -50.59 4.00 Foreman -14.46 0.86 -11.24 0.65 Carphone -17.99 0.94 Carphone -51.46 4.15 -20.79 1.25 Soccer 37.88 -1.62 Soccer -2.43 0.14 0.52 -0.03 Average -22.01 2.47 Average -35.27 3.52 -21.03 1.81 Bjontegaard metrics [33] including bitrate saving (BD video sequences except the highly complex motion rate) and PSNR gain (BD PSNR) are used to compare sequence Soccer. For low motion sequences, the two RD performance curves. The RD plots for Akiyo, proposed codec overcomes HEVC Intra because Foreman, Carphone and Soccer sequences are shown in of good quality SI and accurate CNM. Measured Figure 9. BD Rate, BD PSNR gains obtained with the by Bjontegaard bitrate metric, the proposed codec proposed TDWZ codec over other benchmark schemes saves up to 57.34% for low motion sequences such are presented in Table II and Table III. From the results as Akiyo. For four test sequences, an average 22.01% achieved, the following observations are drawn: birate saving and 2.47 dB BD-PSNR gain are ob- • DL-CNM TDWZ codec versus HEVC Intra: The tained. RD performance of the DL-CNM TDWZ codec is • DL-CNM TDWZ codec versus other DVC codecs: better than that of HEVC Intra for almost all test The proposed DL-CNM TDWZ RD performance is T. V. Huu et al.: Improving TDWZ Correlation Noise Estimation: A Deep Learning based Approach 53 significantly better than the other DVC codecs for ceedings of the 2004 Visual Communications and Image all test video sequences. RD improvements for low Processing, vol. 5308. International Society for Optics motion sequences are higher than for complex mo- and Photonics, 2004, pp. 520–528. [9] X. Artigas, J. Ascenso, M. Dalai, S. Klomp, D. Kubasov, tion sequences. In comparison with DISCOVER- and M. Ouaret, “The DISCOVER codec: architecture, HEVC codec, BD-PSNR gain up to 8.94 dB and techniques and evaluation,” in Proceedings of the Picture BD-rate reduces 72.76% for Akiyo sequence. For Coding Symposium (PCS’07), 2007. complex and high motion sequences, it is difficult [10] S. Milani and G. Calvagno, “A distributed video coder in generating good quality SI and correct CNM. based on the H. 264/AVC standard,” in Proceedings of the 15th European Signal Processing Conference. IEEE, 2007, Therefore, it is hard to obtain such big improve- pp. 673–677. ment. However, our proposed codec achieved an [11] C. Brites, J. Ascenso, and F. Pereira, “Improving trans- average bitrate reduction of 35.27% when com- form domain Wyner-Ziv video coding performance,” in pared with DISCOVER-HEVC and 21.03% when Proceedings of the IEEE International Conference on Acous- compared with TDWZ [27]. tics Speech and Signal Processing, vol. 2, 2006, pp. 525–528. [12] R. Martins, C. Brites, J. Ascenso, and F. Pereira, “Sta- tistical motion learning for improved transform domain Wyner–Ziv video coding,” IET Image Processing, vol. 4, 5 Conclusion no. 1, pp. 28–41, 2010. [13] C. Brites, J. Ascenso, and F. Pereira, “Studying temporal In this work, a method to improve the accuracy of correlation noise modeling for pixel based Wyner-Ziv correlation noise model is proposed for transform do- video coding,” in Proceedings of the International Confer- main Wyner-Ziv video coding. In this proposal, the ence on Image Processing. IEEE, 2006, pp. 273–276. [14] H. V. Xiem, J. Ascenso, and F. Pereira, “Correlation mod- α parameter is estimated by deep learning network eling for a distributed scalable video codec based on the with two hidden layers. Based on the trained model, HEVC standard,” in Proceedings of the 16th International the α parameter is predicted more accurately. The ex- Workshop on Multimedia Signal Processing (MMSP). IEEE, perimental results show that the proposed codec can 2014, pp. 1–6. significantly improve RD performance when compared [15] ——, “HEVC backward compatible scalability: A low encoding complexity distributed video coding based ap- with relevant benchmark schemes. In particular, com- proach,” Signal Processing: Image Communication, vol. 33, pared with low complexity conventional video coding pp. 51–70, 2015. HEVC Intra, RD performance of our proposed codec [16] ——, “Adaptive scalable video coding: An HEVC-based is better for almost test video sequences, especially framework combining the predictive and distributed the low motion sequences. Compared with previous paradigms,” IEEE Transactions on Circuits and Systems for Video Technology, vol. 27, no. 8, pp. 1761–1776, 2016. DVC codecs, such as DISCOVER-HEVC, our proposed [17] C. Brites and F. Pereira, “Correlation noise modeling for codec can achieve significant improvements for all test efficient pixel and transform domain wyner–ziv video sequences. coding,” IEEE Transactions on Circuits and Systems for Video Technology, vol. 18, no. 9, pp. 1177–1190, 2008. [18] C. Brites, J. Ascenso, and F. Pereira, “Learning based de- References coding approach for improved wyner-ziv video coding,” in Proceedings of the Picture Coding Symposium. IEEE, [1] T. Wiegand, G. J. Sullivan, G. Bjontegaard, and A. Luthra, 2012, pp. 165–168. “Overview of the H. 264/AVC video coding standard,” [19] T. Maugey, J. Gauthier, B. Pesquet-Popescu, and IEEE Transactions on Circuits and Systems for Video Tech- C. Guillemot, “Using an exponential power model for nology, vol. 13, no. 7, pp. 560–576, 2003. wyner-ziv video coding,” in Proceedings of the IEEE [2] G. J. Sullivan, J.-R. Ohm, W.-J. Han, and T. Wiegand, International Conference on Acoustics, Speech and Signal “Overview of the high efficiency video coding (HEVC) Processing. IEEE, 2010, pp. 2338–2341. standard,” IEEE Transactions on Circuits and Systems for [20] H. Qin, B. Song, Y. Zhao, and H. Liu, “Adaptive Cor- Video Technology, vol. 22, no. 12, pp. 1649–1668, 2012. relation Noise Model for DC Coefficients in Wyner-Ziv [3] N. H. Phat, V. Tran-Quang, and T. Miyoshi, “Low- Video Coding,” ETRI Journal, vol. 34, no. 2, pp. 190–198, complexity motion estimation algorithm using edge fea- 2012. ture for video compression on wireless video sensor [21] J. Park, B. Jeon, D. Wang, and A. Vincent, “Wyner- networks,” in Proceedings of the 13th Asia-Pacific Network Ziv video coding with region adaptive quantization and Operations and Management Symposium. IEEE, 2011, pp. progressive channel noise modeling,” in Proceedings of 3–10. the IEEE International Symposium on Broadband Multimedia [4] ——, “Video compression schemes using edge feature Systems and Broadcasting, 2009, pp. 1–6. on wireless video sensor networks,” Journal of Electrical [22] H. Van Luong, X. Huang, and S. Forchhammer, “Adap- and Computer Engineering, vol. 2012, pp. 1–20, 2012. tive noise model for transform domain Wyner-Ziv video [5] D. Slepian and J. Wolf, “Noiseless coding of correlated using clustering of DCT blocks,” in Proceedings of the information sources,” IEEE Transactions on Information IEEE 13th International Workshop on Multimedia Signal Theory, vol. 19, no. 4, pp. 471–480, 1973. Processing, 2011, pp. 1–6. [6] A. Wyner and J. Ziv, “The rate-distortion function for [23] T. Chen, H. Liu, Q. Shen, T. Yue, X. Cao, and Z. Ma, source coding with side information at the decoder,” “Deepcoder: A deep neural network based video com- IEEE Transactions on Information Theory, vol. 22, no. 1, pression,” in Proceedings of the IEEE Visual Communica- pp. 1–10, 1976. tions and Image Processing (VCIP). IEEE, 2017, pp. 1–4. [7] R. Puri, A. Majumdar, and K. Ramchandran, “PRISM: [24] R. Song, D. Liu, H. Li, and F. Wu, “Neural network-based A video coding paradigm with motion estimation at the arithmetic coding of intra prediction modes in HEVC,” decoder,” IEEE Transactions on Image Processing, vol. 16, in Proceedings of the IEEE Visual Communications and Image no. 10, pp. 2436–2448, 2007. Processing (VCIP), 2017, pp. 1–4. [8] A. Aaron, S. D. Rane, E. Setton, and B. Girod, [25] B. Tian and W. Xiong, “A Side Information Generation “Transform-domain Wyner-Ziv codec for video,” in Pro- method using Deep Learning for Distributed Video Cod- 54 REV Journal on Electronics and Communications, Vol. 10, No. 1–2, January–June, 2020 ing,” in Journal of Physics: Conference Series, no. 6, 2018, Thao Nguyen Thi Huong received the B. pp. 1–6. Eng. in Electrical Engineering from Posts and [26] B. Dash, S. Rup, A. Mohapatra, B. Majhi, and M. Swamy, Telecommunications Institute of Technology “Multi-resolution extreme learning machine-based side in 2003. She is currently a lecturer and pursu- information estimation in distributed video coding,” ing the Ph.D. degree in Posts and Telecommu- nications Institute of Technology. Her research Multimedia Tools and Applications, vol. 77, no. 20, pp. interests are digital image processing, video 27 301–27 335, 2018. communications. [27] T. V. Huu, T. N. T. Huong, M. N. Ngoc, and H. V. Xiem, “Improving performance of distributed video coding by consecutively refining of side information and correla- tion noise model,” in Proceedings of the 19th International Symposium on Communications and Information Technolo- gies (ISCIT). IEEE, 2019, pp. 502–506. [28] J. Lainema, F. Bossen, W.-J. Han, J. Min, and K. Ugur, Xiem Hoang Van is the Deputy Head, Man- IEEE Transactions ager of the Department of Robotics Engineer- “Intra coding of the HEVC standard,” ing, Faculty of Electronics and Telecommuni- on Circuits and Systems for Video Technology, vol. 22, no. 12, cations, Vietnam National University - Uni- pp. 1792–1801, 2012. versity of Engineering and Technology (VNU- [29] C. Brites and F. Pereira, “Distributed video coding: UET). He received the Ph.D. degree (with Dis- Assessing the HEVC upgrade,” Signal Processing: Image tinctions) from Lisbon University, Portugal, in Communication, vol. 32, pp. 81–105, 2015. 2015, the M.Sc. degree from Sungkyunkwan [30] R. Martins, C. Brites, J. Ascenso, and F. Pereira, “Refining University, South Korea, in 2011, and the B.E side information for improved transform domain Wyner- degree from Hanoi University of Science and Ziv video coding,” IEEE Transactions on Circuits and Technology, Vietnam, in 2009, all in Electrical Systems for Video Technology and Computer Engineering. He is an executive committee member of , vol. 19, no. 9, pp. 1327–1341, VNU-UTS Joint Innovation and Technology research center. His re- 2009. search interests are machine learning, image, video communications [31] Google, colaboratory: frequently asked questions. and robot vision. [Online]. Available: http-s://research.google.com/ co- laboratory/faq.html (accessed: 6-21-2018) [32] HEVC reference software. [Online]. Available: https:// hevc.hhi.fraunhofer.de/svn/svn_HEVCSoftware/ [33] G. Bjontegaard, “Calculation of average PSNR dif- San Vu Van received the Ph.D. degree from Electronics and Telecommunications Research ferences between RD-curves,” VCEG-M33, 13th ITU-T VCEG Meeting, Austin, TX, USA Institute, Republic of Korea, in 2000. He is , 2001. currently an Associate Professor at Posts and Telecommunications Institute of Technology. His research interests are Transmission and Digital Signal Processing. Tien Vu Huu received the B. Eng. in Elec- trical Engineering from Hanoi University of Technology, Hanoi, Vietnam in 2002. He re- ceived the Ph.D. degree from Chulalongkorn, Thailand, in 2011. He is currently working at Multimedia Faculty, Posts and Telecommuni- cations Institute of Technology. His research interests are digital image processing, video communications and virtual reality.
File đính kèm:
improving_tdwz_correlation_noise_estimation_a_deep_learning.pdf