Efficient market hypothesis and calendar effects: Empirical evidences from the Vietnam stock markets
Vietnam’s stock market although has small scale without a long history of development but the
exchange has just started for a massive development. There have also been a number of anomalies,
suggested that the market is not efficient. Therefore, there is a possibility that active investors with
right strategy can consistently achieve higher profit than the market portfolio. This paper analyzes the
statistical and economic significance of the calendar anomalies to propose appropriate strategies or
recommendations. Studying the calendar anomalies in Vietnam also diversifies the research scope and
validates some hypotheses in the past. In this research, the authors just analyze the monthly effects and
the experimental results of this study may have significant implications not only for financial managers,
financial advisers and investors but also for government to implement policy on stock market.
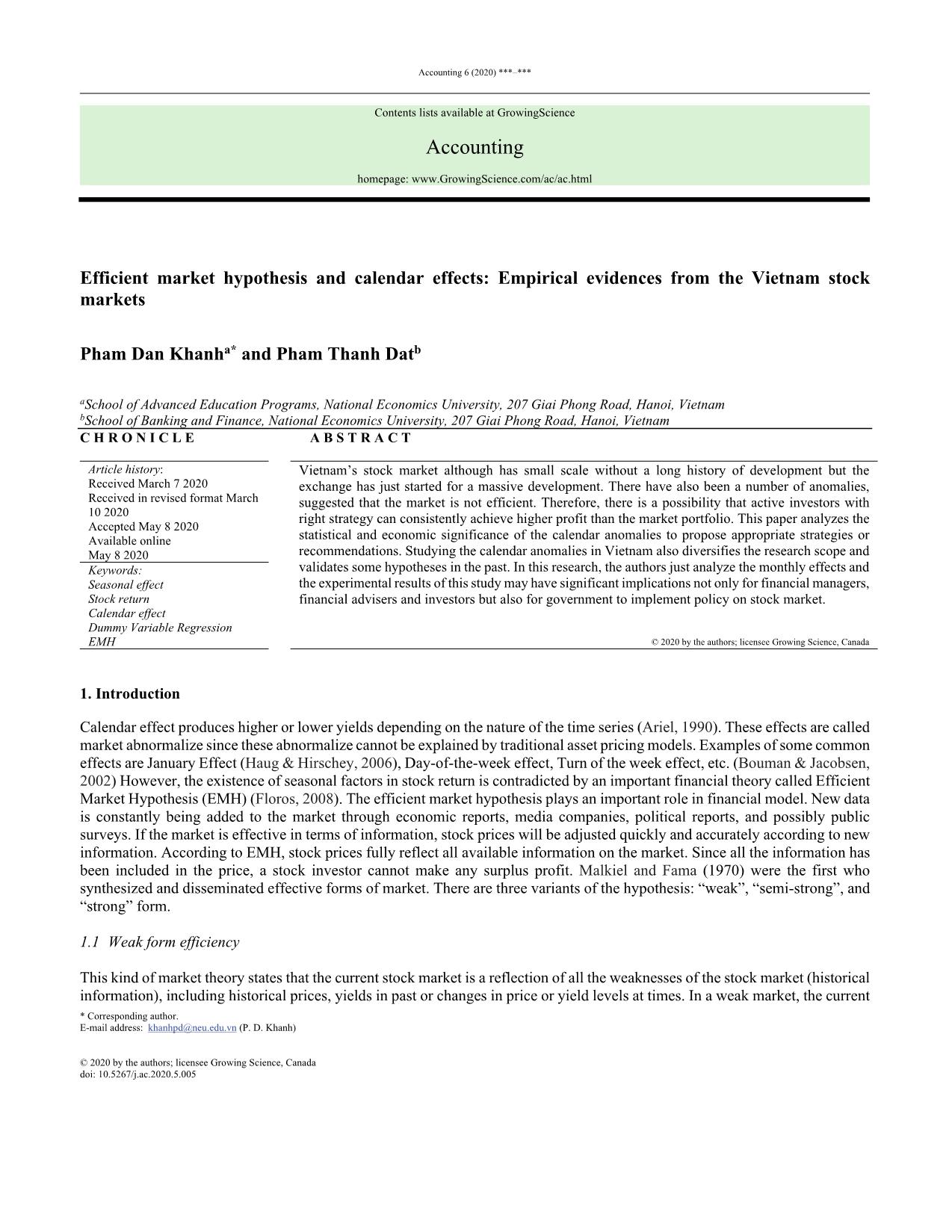
Trang 1
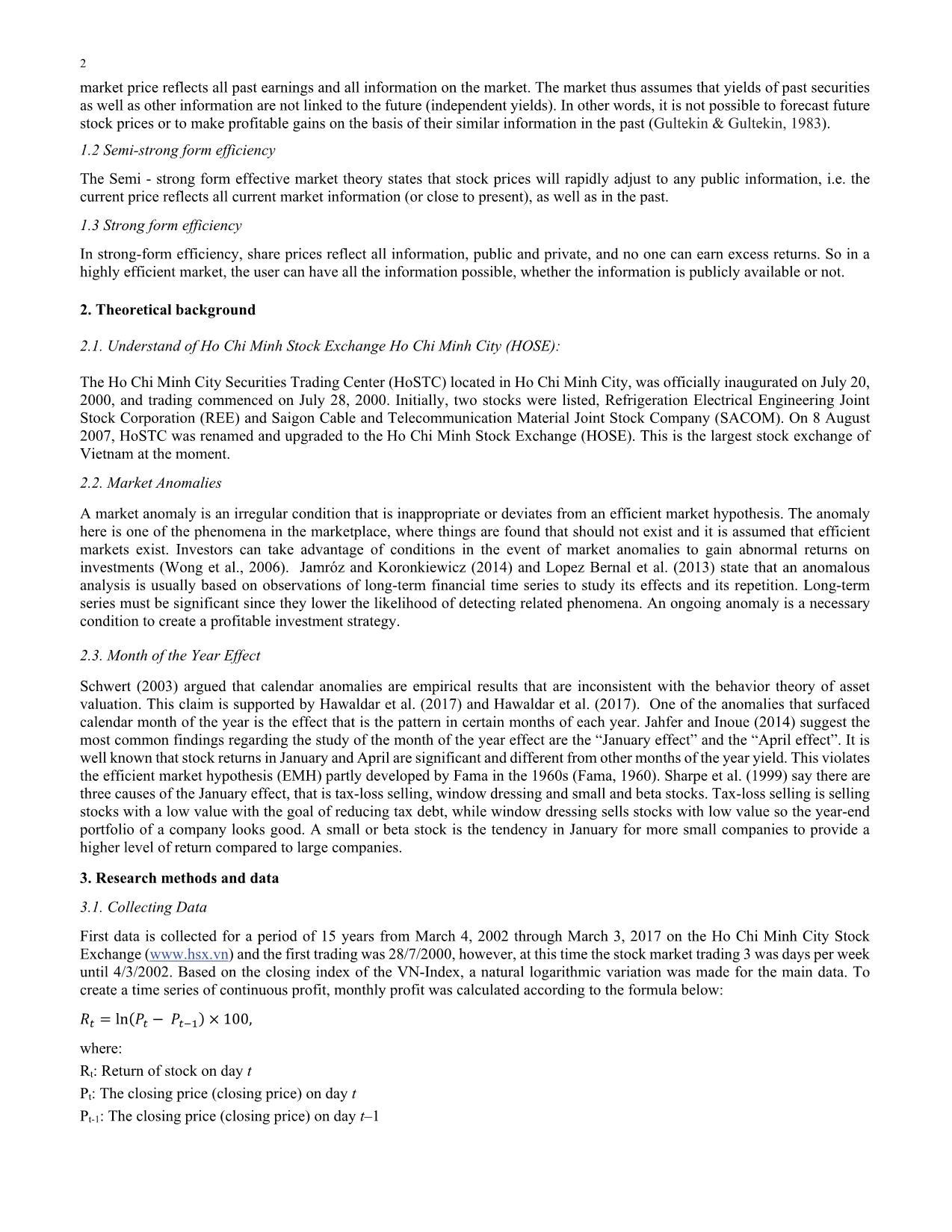
Trang 2
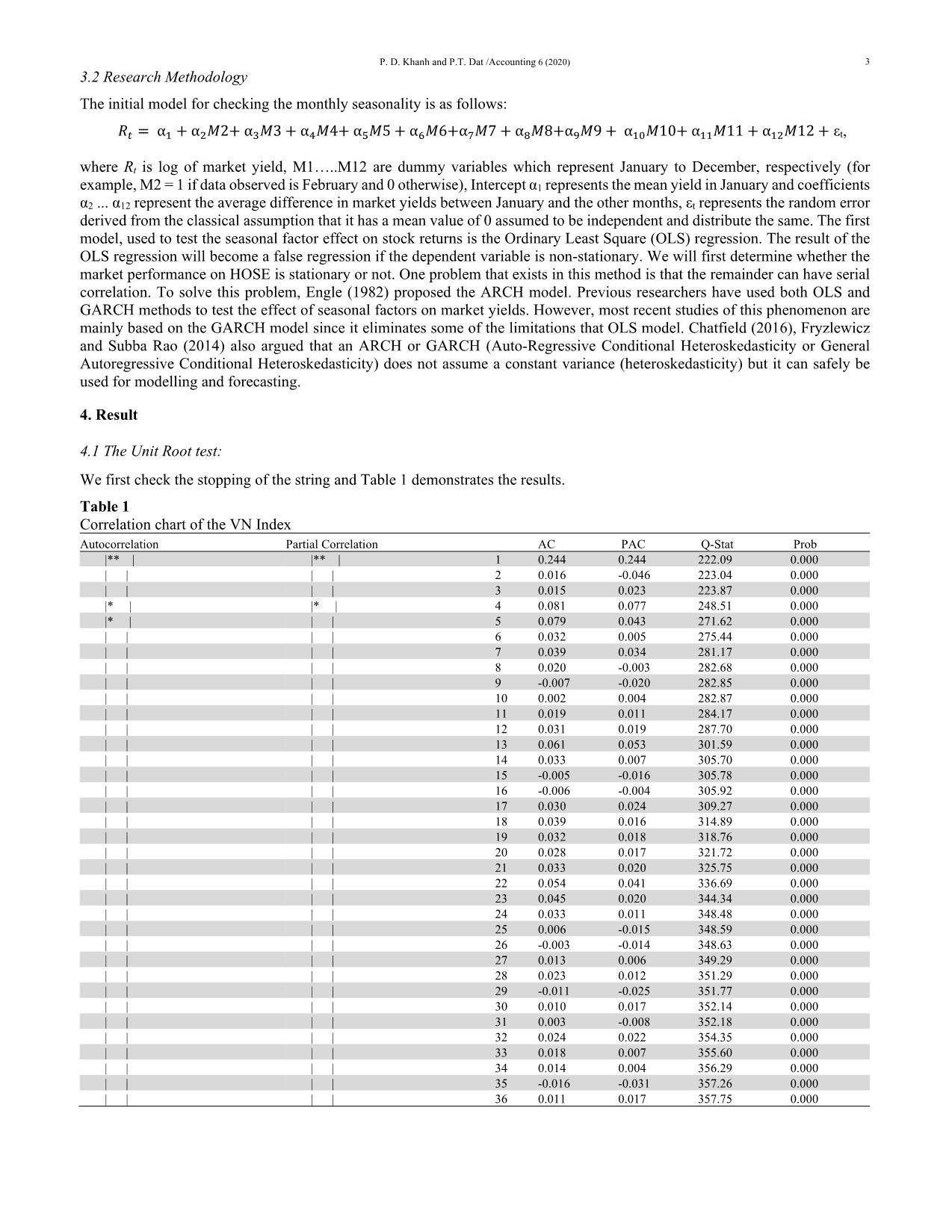
Trang 3
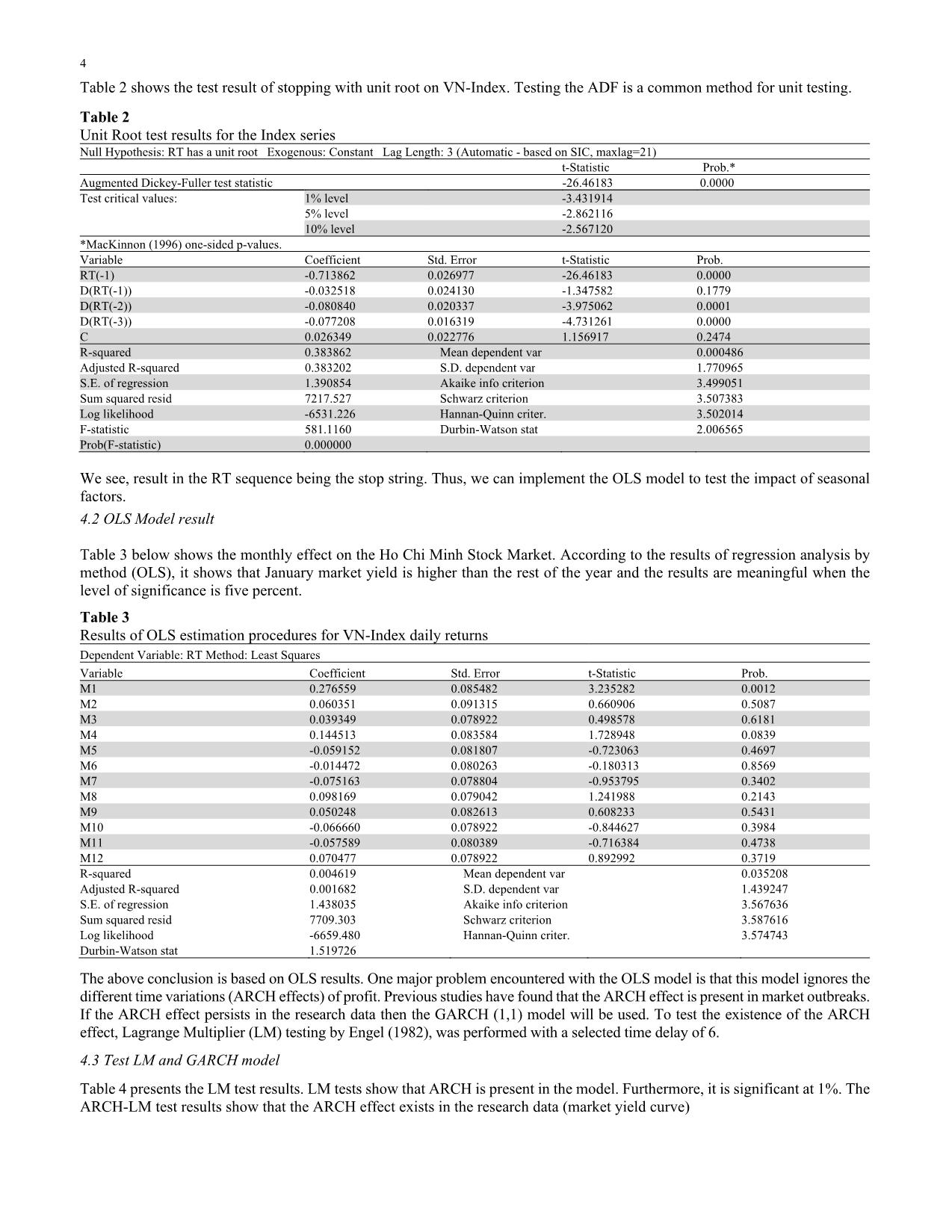
Trang 4
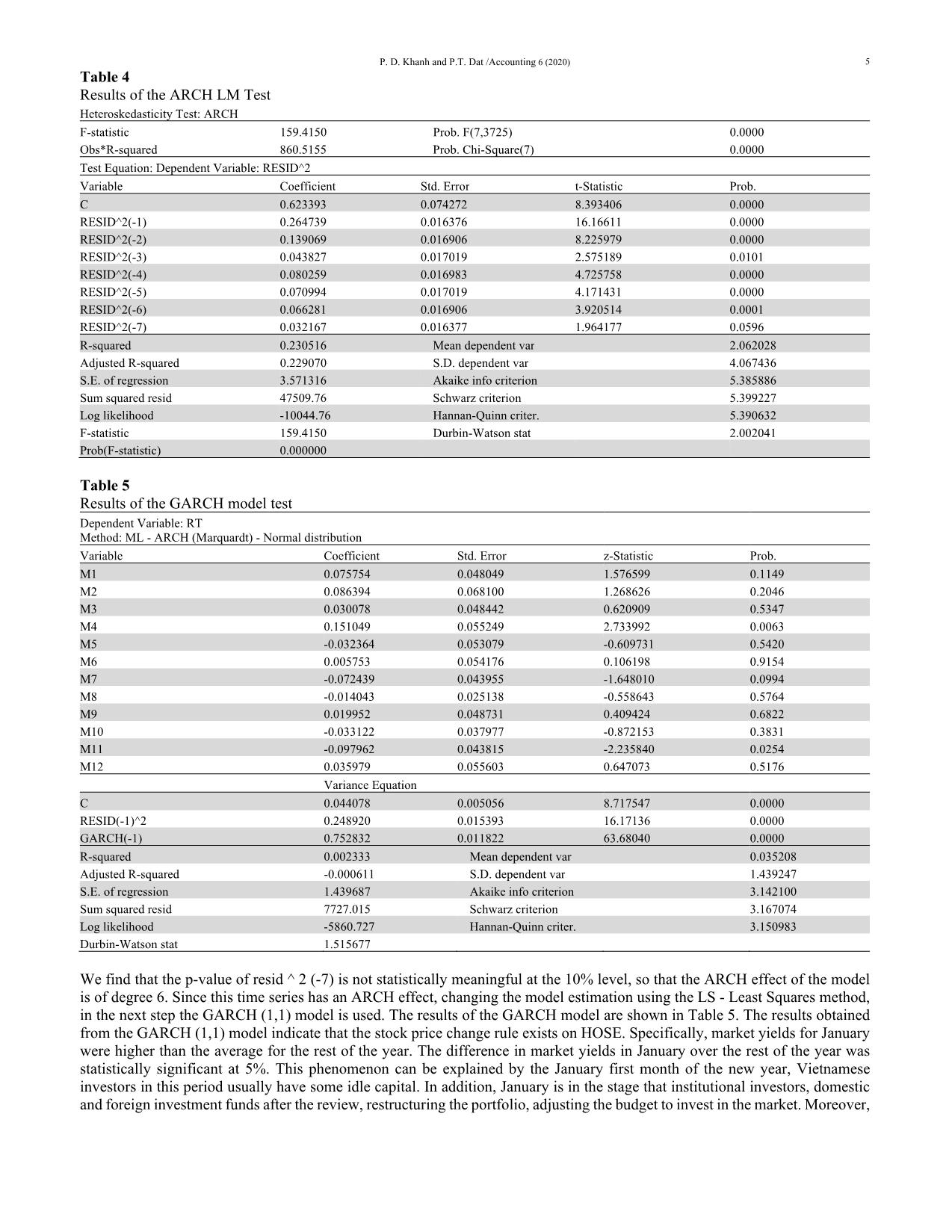
Trang 5
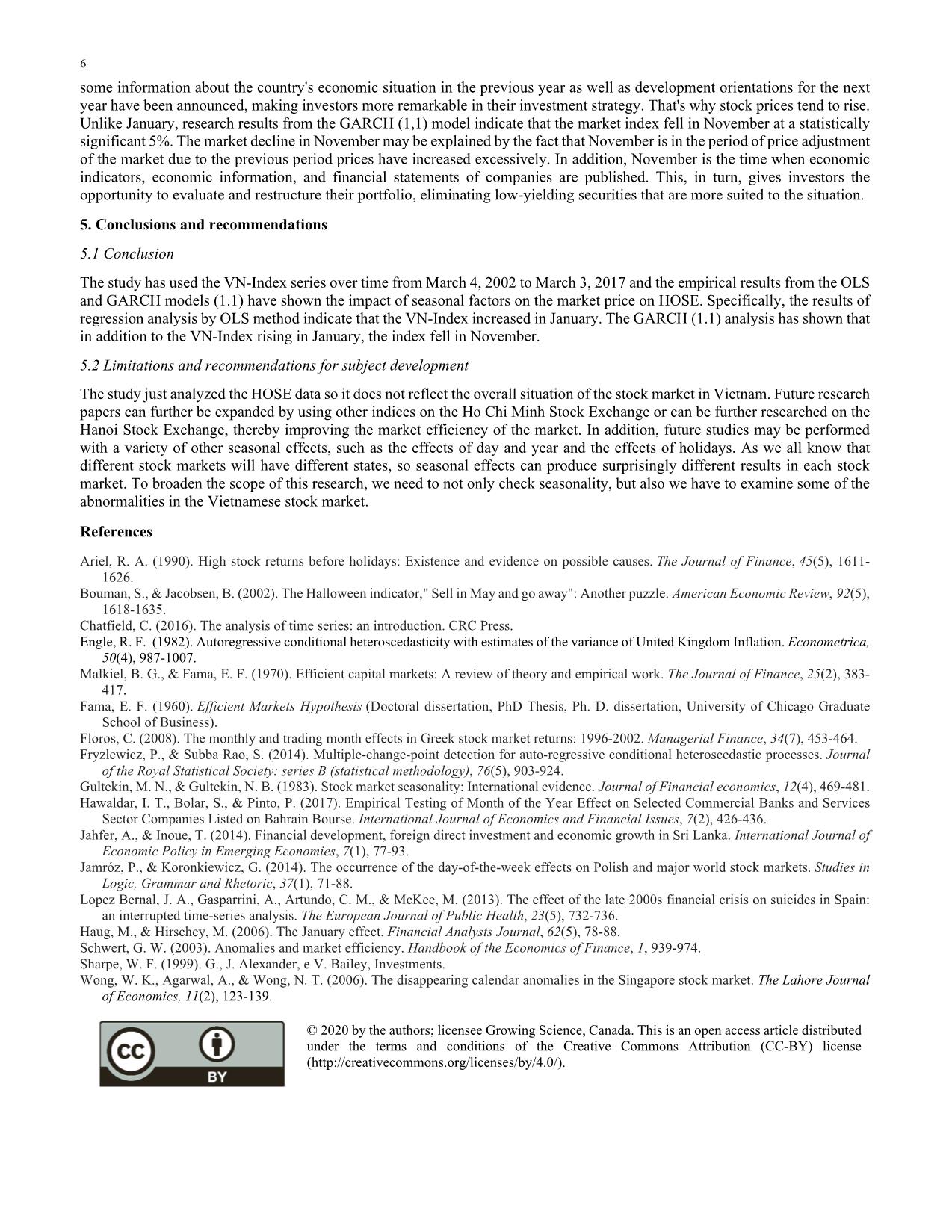
Trang 6
Tóm tắt nội dung tài liệu: Efficient market hypothesis and calendar effects: Empirical evidences from the Vietnam stock markets
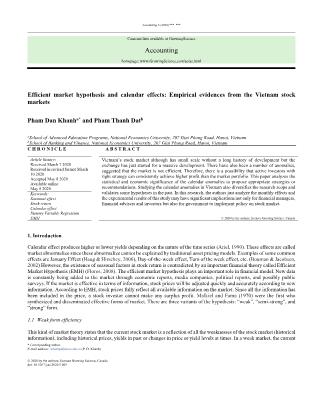
as follows: 𝑅௧ = αଵ + αଶ𝑀2+ αଷ𝑀3 + αସ𝑀4+ αହ𝑀5 + α𝑀6+α𝑀7 + α଼𝑀8+αଽ𝑀9 + αଵ𝑀10+ αଵଵ𝑀11 + αଵଶ𝑀12 + εt, where Rt is log of market yield, M1..M12 are dummy variables which represent January to December, respectively (for example, M2 = 1 if data observed is February and 0 otherwise), Intercept α1 represents the mean yield in January and coefficients α2 ... α12 represent the average difference in market yields between January and the other months, εt represents the random error derived from the classical assumption that it has a mean value of 0 assumed to be independent and distribute the same. The first model, used to test the seasonal factor effect on stock returns is the Ordinary Least Square (OLS) regression. The result of the OLS regression will become a false regression if the dependent variable is non-stationary. We will first determine whether the market performance on HOSE is stationary or not. One problem that exists in this method is that the remainder can have serial correlation. To solve this problem, Engle (1982) proposed the ARCH model. Previous researchers have used both OLS and GARCH methods to test the effect of seasonal factors on market yields. However, most recent studies of this phenomenon are mainly based on the GARCH model since it eliminates some of the limitations that OLS model. Chatfield (2016), Fryzlewicz and Subba Rao (2014) also argued that an ARCH or GARCH (Auto-Regressive Conditional Heteroskedasticity or General Autoregressive Conditional Heteroskedasticity) does not assume a constant variance (heteroskedasticity) but it can safely be used for modelling and forecasting. 4. Result 4.1 The Unit Root test: We first check the stopping of the string and Table 1 demonstrates the results. Table 1 Correlation chart of the VN Index Autocorrelation Partial Correlation AC PAC Q-Stat Prob |** | |** | 1 0.244 0.244 222.09 0.000 | | | | 2 0.016 -0.046 223.04 0.000 | | | | 3 0.015 0.023 223.87 0.000 |* | |* | 4 0.081 0.077 248.51 0.000 |* | | | 5 0.079 0.043 271.62 0.000 | | | | 6 0.032 0.005 275.44 0.000 | | | | 7 0.039 0.034 281.17 0.000 | | | | 8 0.020 -0.003 282.68 0.000 | | | | 9 -0.007 -0.020 282.85 0.000 | | | | 10 0.002 0.004 282.87 0.000 | | | | 11 0.019 0.011 284.17 0.000 | | | | 12 0.031 0.019 287.70 0.000 | | | | 13 0.061 0.053 301.59 0.000 | | | | 14 0.033 0.007 305.70 0.000 | | | | 15 -0.005 -0.016 305.78 0.000 | | | | 16 -0.006 -0.004 305.92 0.000 | | | | 17 0.030 0.024 309.27 0.000 | | | | 18 0.039 0.016 314.89 0.000 | | | | 19 0.032 0.018 318.76 0.000 | | | | 20 0.028 0.017 321.72 0.000 | | | | 21 0.033 0.020 325.75 0.000 | | | | 22 0.054 0.041 336.69 0.000 | | | | 23 0.045 0.020 344.34 0.000 | | | | 24 0.033 0.011 348.48 0.000 | | | | 25 0.006 -0.015 348.59 0.000 | | | | 26 -0.003 -0.014 348.63 0.000 | | | | 27 0.013 0.006 349.29 0.000 | | | | 28 0.023 0.012 351.29 0.000 | | | | 29 -0.011 -0.025 351.77 0.000 | | | | 30 0.010 0.017 352.14 0.000 | | | | 31 0.003 -0.008 352.18 0.000 | | | | 32 0.024 0.022 354.35 0.000 | | | | 33 0.018 0.007 355.60 0.000 | | | | 34 0.014 0.004 356.29 0.000 | | | | 35 -0.016 -0.031 357.26 0.000 | | | | 36 0.011 0.017 357.75 0.000 4 Table 2 shows the test result of stopping with unit root on VN-Index. Testing the ADF is a common method for unit testing. Table 2 Unit Root test results for the Index series Null Hypothesis: RT has a unit root Exogenous: Constant Lag Length: 3 (Automatic - based on SIC, maxlag=21) t-Statistic Prob.* Augmented Dickey-Fuller test statistic -26.46183 0.0000 Test critical values: 1% level -3.431914 5% level -2.862116 10% level -2.567120 *MacKinnon (1996) one-sided p-values. Variable Coefficient Std. Error t-Statistic Prob. RT(-1) -0.713862 0.026977 -26.46183 0.0000 D(RT(-1)) -0.032518 0.024130 -1.347582 0.1779 D(RT(-2)) -0.080840 0.020337 -3.975062 0.0001 D(RT(-3)) -0.077208 0.016319 -4.731261 0.0000 C 0.026349 0.022776 1.156917 0.2474 R-squared 0.383862 Mean dependent var 0.000486 Adjusted R-squared 0.383202 S.D. dependent var 1.770965 S.E. of regression 1.390854 Akaike info criterion 3.499051 Sum squared resid 7217.527 Schwarz criterion 3.507383 Log likelihood -6531.226 Hannan-Quinn criter. 3.502014 F-statistic 581.1160 Durbin-Watson stat 2.006565 Prob(F-statistic) 0.000000 We see, result in the RT sequence being the stop string. Thus, we can implement the OLS model to test the impact of seasonal factors. 4.2 OLS Model result Table 3 below shows the monthly effect on the Ho Chi Minh Stock Market. According to the results of regression analysis by method (OLS), it shows that January market yield is higher than the rest of the year and the results are meaningful when the level of significance is five percent. Table 3 Results of OLS estimation procedures for VN-Index daily returns Dependent Variable: RT Method: Least Squares Variable Coefficient Std. Error t-Statistic Prob. M1 0.276559 0.085482 3.235282 0.0012 M2 0.060351 0.091315 0.660906 0.5087 M3 0.039349 0.078922 0.498578 0.6181 M4 0.144513 0.083584 1.728948 0.0839 M5 -0.059152 0.081807 -0.723063 0.4697 M6 -0.014472 0.080263 -0.180313 0.8569 M7 -0.075163 0.078804 -0.953795 0.3402 M8 0.098169 0.079042 1.241988 0.2143 M9 0.050248 0.082613 0.608233 0.5431 M10 -0.066660 0.078922 -0.844627 0.3984 M11 -0.057589 0.080389 -0.716384 0.4738 M12 0.070477 0.078922 0.892992 0.3719 R-squared 0.004619 Mean dependent var 0.035208 Adjusted R-squared 0.001682 S.D. dependent var 1.439247 S.E. of regression 1.438035 Akaike info criterion 3.567636 Sum squared resid 7709.303 Schwarz criterion 3.587616 Log likelihood -6659.480 Hannan-Quinn criter. 3.574743 Durbin-Watson stat 1.519726 The above conclusion is based on OLS results. One major problem encountered with the OLS model is that this model ignores the different time variations (ARCH effects) of profit. Previous studies have found that the ARCH effect is present in market outbreaks. If the ARCH effect persists in the research data then the GARCH (1,1) model will be used. To test the existence of the ARCH effect, Lagrange Multiplier (LM) testing by Engel (1982), was performed with a selected time delay of 6. 4.3 Test LM and GARCH model Table 4 presents the LM test results. LM tests show that ARCH is present in the model. Furthermore, it is significant at 1%. The ARCH-LM test results show that the ARCH effect exists in the research data (market yield curve) P. D. Khanh and P.T. Dat /Accounting 6 (2020) 5 Table 4 Results of the ARCH LM Test Heteroskedasticity Test: ARCH F-statistic 159.4150 Prob. F(7,3725) 0.0000 Obs*R-squared 860.5155 Prob. Chi-Square(7) 0.0000 Test Equation: Dependent Variable: RESID^2 Variable Coefficient Std. Error t-Statistic Prob. C 0.623393 0.074272 8.393406 0.0000 RESID^2(-1) 0.264739 0.016376 16.16611 0.0000 RESID^2(-2) 0.139069 0.016906 8.225979 0.0000 RESID^2(-3) 0.043827 0.017019 2.575189 0.0101 RESID^2(-4) 0.080259 0.016983 4.725758 0.0000 RESID^2(-5) 0.070994 0.017019 4.171431 0.0000 RESID^2(-6) 0.066281 0.016906 3.920514 0.0001 RESID^2(-7) 0.032167 0.016377 1.964177 0.0596 R-squared 0.230516 Mean dependent var 2.062028 Adjusted R-squared 0.229070 S.D. dependent var 4.067436 S.E. of regression 3.571316 Akaike info criterion 5.385886 Sum squared resid 47509.76 Schwarz criterion 5.399227 Log likelihood -10044.76 Hannan-Quinn criter. 5.390632 F-statistic 159.4150 Durbin-Watson stat 2.002041 Prob(F-statistic) 0.000000 Table 5 Results of the GARCH model test Dependent Variable: RT Method: ML - ARCH (Marquardt) - Normal distribution Variable Coefficient Std. Error z-Statistic Prob. M1 0.075754 0.048049 1.576599 0.1149 M2 0.086394 0.068100 1.268626 0.2046 M3 0.030078 0.048442 0.620909 0.5347 M4 0.151049 0.055249 2.733992 0.0063 M5 -0.032364 0.053079 -0.609731 0.5420 M6 0.005753 0.054176 0.106198 0.9154 M7 -0.072439 0.043955 -1.648010 0.0994 M8 -0.014043 0.025138 -0.558643 0.5764 M9 0.019952 0.048731 0.409424 0.6822 M10 -0.033122 0.037977 -0.872153 0.3831 M11 -0.097962 0.043815 -2.235840 0.0254 M12 0.035979 0.055603 0.647073 0.5176 Variance Equation C 0.044078 0.005056 8.717547 0.0000 RESID(-1)^2 0.248920 0.015393 16.17136 0.0000 GARCH(-1) 0.752832 0.011822 63.68040 0.0000 R-squared 0.002333 Mean dependent var 0.035208 Adjusted R-squared -0.000611 S.D. dependent var 1.439247 S.E. of regression 1.439687 Akaike info criterion 3.142100 Sum squared resid 7727.015 Schwarz criterion 3.167074 Log likelihood -5860.727 Hannan-Quinn criter. 3.150983 Durbin-Watson stat 1.515677 We find that the p-value of resid ^ 2 (-7) is not statistically meaningful at the 10% level, so that the ARCH effect of the model is of degree 6. Since this time series has an ARCH effect, changing the model estimation using the LS - Least Squares method, in the next step the GARCH (1,1) model is used. The results of the GARCH model are shown in Table 5. The results obtained from the GARCH (1,1) model indicate that the stock price change rule exists on HOSE. Specifically, market yields for January were higher than the average for the rest of the year. The difference in market yields in January over the rest of the year was statistically significant at 5%. This phenomenon can be explained by the January first month of the new year, Vietnamese investors in this period usually have some idle capital. In addition, January is in the stage that institutional investors, domestic and foreign investment funds after the review, restructuring the portfolio, adjusting the budget to invest in the market. Moreover, 6 some information about the country's economic situation in the previous year as well as development orientations for the next year have been announced, making investors more remarkable in their investment strategy. That's why stock prices tend to rise. Unlike January, research results from the GARCH (1,1) model indicate that the market index fell in November at a statistically significant 5%. The market decline in November may be explained by the fact that November is in the period of price adjustment of the market due to the previous period prices have increased excessively. In addition, November is the time when economic indicators, economic information, and financial statements of companies are published. This, in turn, gives investors the opportunity to evaluate and restructure their portfolio, eliminating low-yielding securities that are more suited to the situation. 5. Conclusions and recommendations 5.1 Conclusion The study has used the VN-Index series over time from March 4, 2002 to March 3, 2017 and the empirical results from the OLS and GARCH models (1.1) have shown the impact of seasonal factors on the market price on HOSE. Specifically, the results of regression analysis by OLS method indicate that the VN-Index increased in January. The GARCH (1.1) analysis has shown that in addition to the VN-Index rising in January, the index fell in November. 5.2 Limitations and recommendations for subject development The study just analyzed the HOSE data so it does not reflect the overall situation of the stock market in Vietnam. Future research papers can further be expanded by using other indices on the Ho Chi Minh Stock Exchange or can be further researched on the Hanoi Stock Exchange, thereby improving the market efficiency of the market. In addition, future studies may be performed with a variety of other seasonal effects, such as the effects of day and year and the effects of holidays. As we all know that different stock markets will have different states, so seasonal effects can produce surprisingly different results in each stock market. To broaden the scope of this research, we need to not only check seasonality, but also we have to examine some of the abnormalities in the Vietnamese stock market. References Ariel, R. A. (1990). High stock returns before holidays: Existence and evidence on possible causes. The Journal of Finance, 45(5), 1611- 1626. Bouman, S., & Jacobsen, B. (2002). The Halloween indicator," Sell in May and go away": Another puzzle. American Economic Review, 92(5), 1618-1635. Chatfield, C. (2016). The analysis of time series: an introduction. CRC Press. Engle, R. F. (1982). Autoregressive conditional heteroscedasticity with estimates of the variance of United Kingdom Inflation. Econometrica, 50(4), 987-1007. Malkiel, B. G., & Fama, E. F. (1970). Efficient capital markets: A review of theory and empirical work. The Journal of Finance, 25(2), 383- 417. Fama, E. F. (1960). Efficient Markets Hypothesis (Doctoral dissertation, PhD Thesis, Ph. D. dissertation, University of Chicago Graduate School of Business). Floros, C. (2008). The monthly and trading month effects in Greek stock market returns: 1996‐2002. Managerial Finance, 34(7), 453-464. Fryzlewicz, P., & Subba Rao, S. (2014). Multiple‐change‐point detection for auto‐regressive conditional heteroscedastic processes. Journal of the Royal Statistical Society: series B (statistical methodology), 76(5), 903-924. Gultekin, M. N., & Gultekin, N. B. (1983). Stock market seasonality: International evidence. Journal of Financial economics, 12(4), 469-481. Hawaldar, I. T., Bolar, S., & Pinto, P. (2017). Empirical Testing of Month of the Year Effect on Selected Commercial Banks and Services Sector Companies Listed on Bahrain Bourse. International Journal of Economics and Financial Issues, 7(2), 426-436. Jahfer, A., & Inoue, T. (2014). Financial development, foreign direct investment and economic growth in Sri Lanka. International Journal of Economic Policy in Emerging Economies, 7(1), 77-93. Jamróz, P., & Koronkiewicz, G. (2014). The occurrence of the day-of-the-week effects on Polish and major world stock markets. Studies in Logic, Grammar and Rhetoric, 37(1), 71-88. Lopez Bernal, J. A., Gasparrini, A., Artundo, C. M., & McKee, M. (2013). The effect of the late 2000s financial crisis on suicides in Spain: an interrupted time-series analysis. The European Journal of Public Health, 23(5), 732-736. Haug, M., & Hirschey, M. (2006). The January effect. Financial Analysts Journal, 62(5), 78-88. Schwert, G. W. (2003). Anomalies and market efficiency. Handbook of the Economics of Finance, 1, 939-974. Sharpe, W. F. (1999). G., J. Alexander, e V. Bailey, Investments. Wong, W. K., Agarwal, A., & Wong, N. T. (2006). The disappearing calendar anomalies in the Singapore stock market. The Lahore Journal of Economics, 11(2), 123-139. © 2020 by the authors; licensee Growing Science, Canada. This is an open access article distributed under the terms and conditions of the Creative Commons Attribution (CC-BY) license (
File đính kèm:
efficient_market_hypothesis_and_calendar_effects_empirical_e.pdf