Development and implementation of polygenic risk score in Vietnamese population
Abstract: Recent technological advancements and availability of genetic databases have facilitated the integration of genetic factors into risk prediction models. A Polygenic Risk Score (PRS) combines the effect of many Single Nucleotide Polymorphisms (SNP) into a single score. This score has lately been shown to have a clinically predictive value in various common diseases. Some clinical interpretations of PRS are summarized in this review for coronary artery disease, breast cancer, prostate cancer, diabetes mellitus, and Alzheimer’s disease. While these findings gave support to the implementation of PRS in clinical settings, the populations of interest were derived mainly from European ancestry. Therefore, applying these findings to non-European ancestry (Vietnamese in this context) requires many efforts and cautions. This review aims to articulate the evidence supporting the clinical use of PRS, the concepts behind the validity of PRS, approach to implement PRS in Vietnamese population, and cautions in selecting methods and thresholds to develop an appropriate PRS
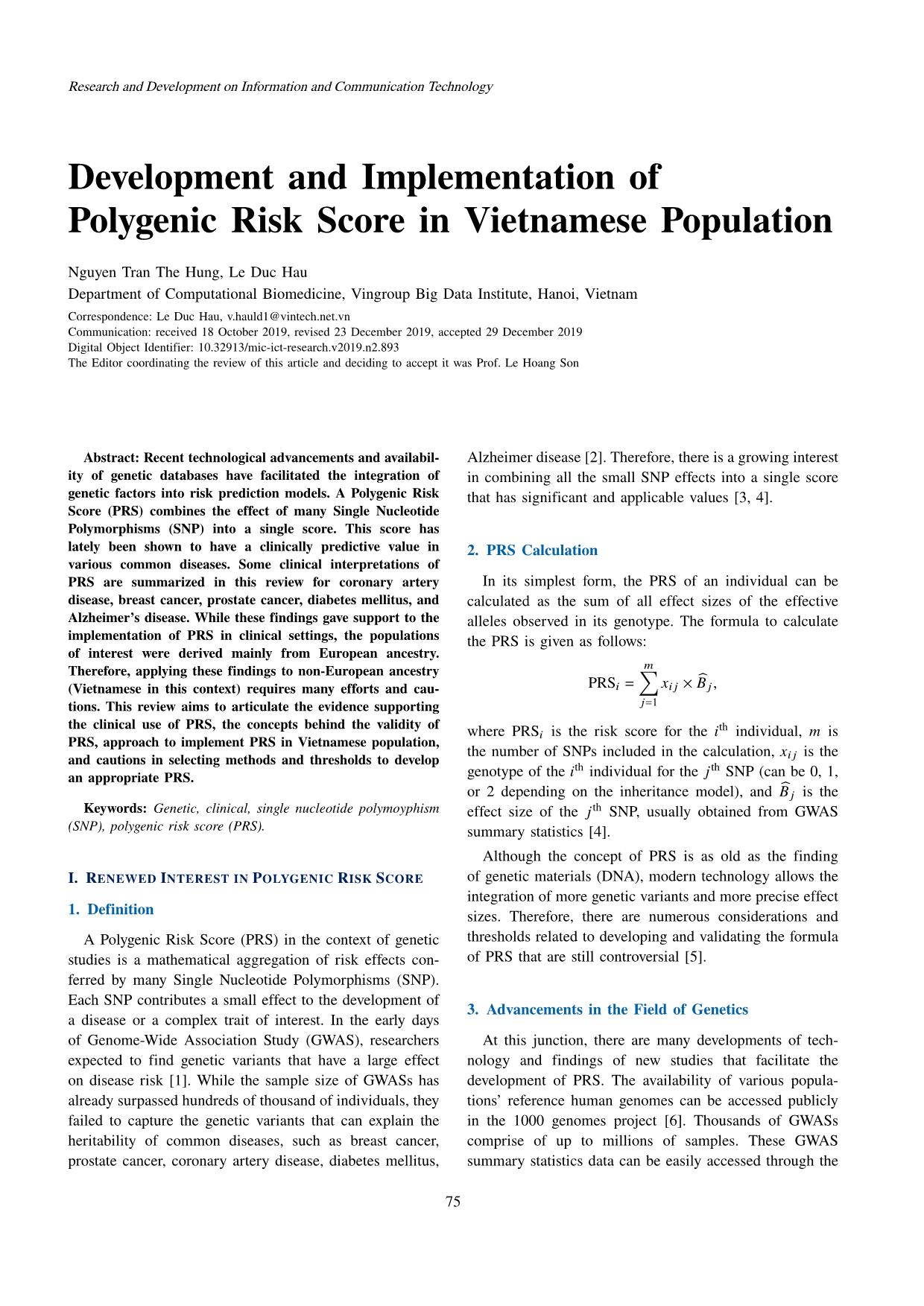
Trang 1
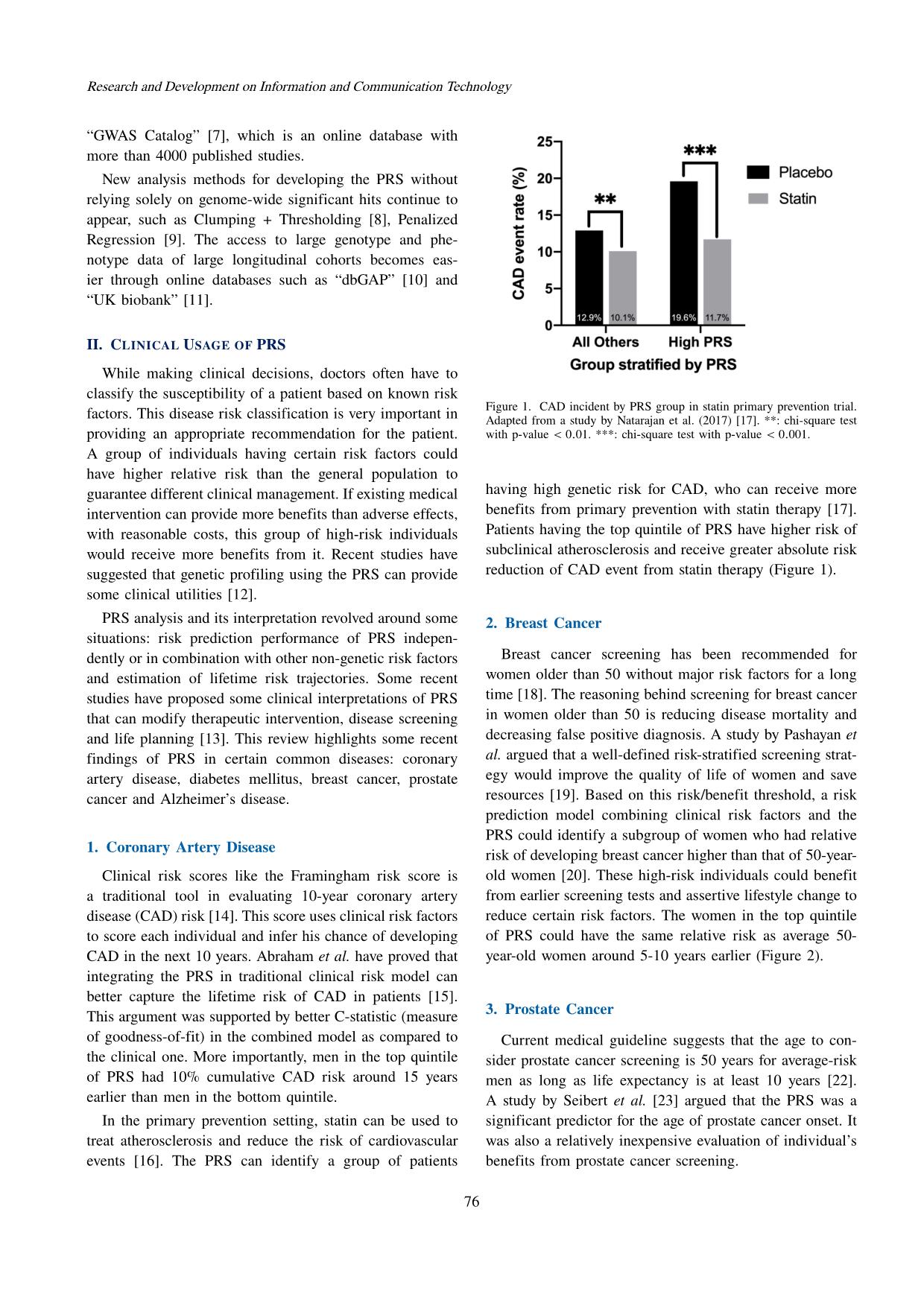
Trang 2
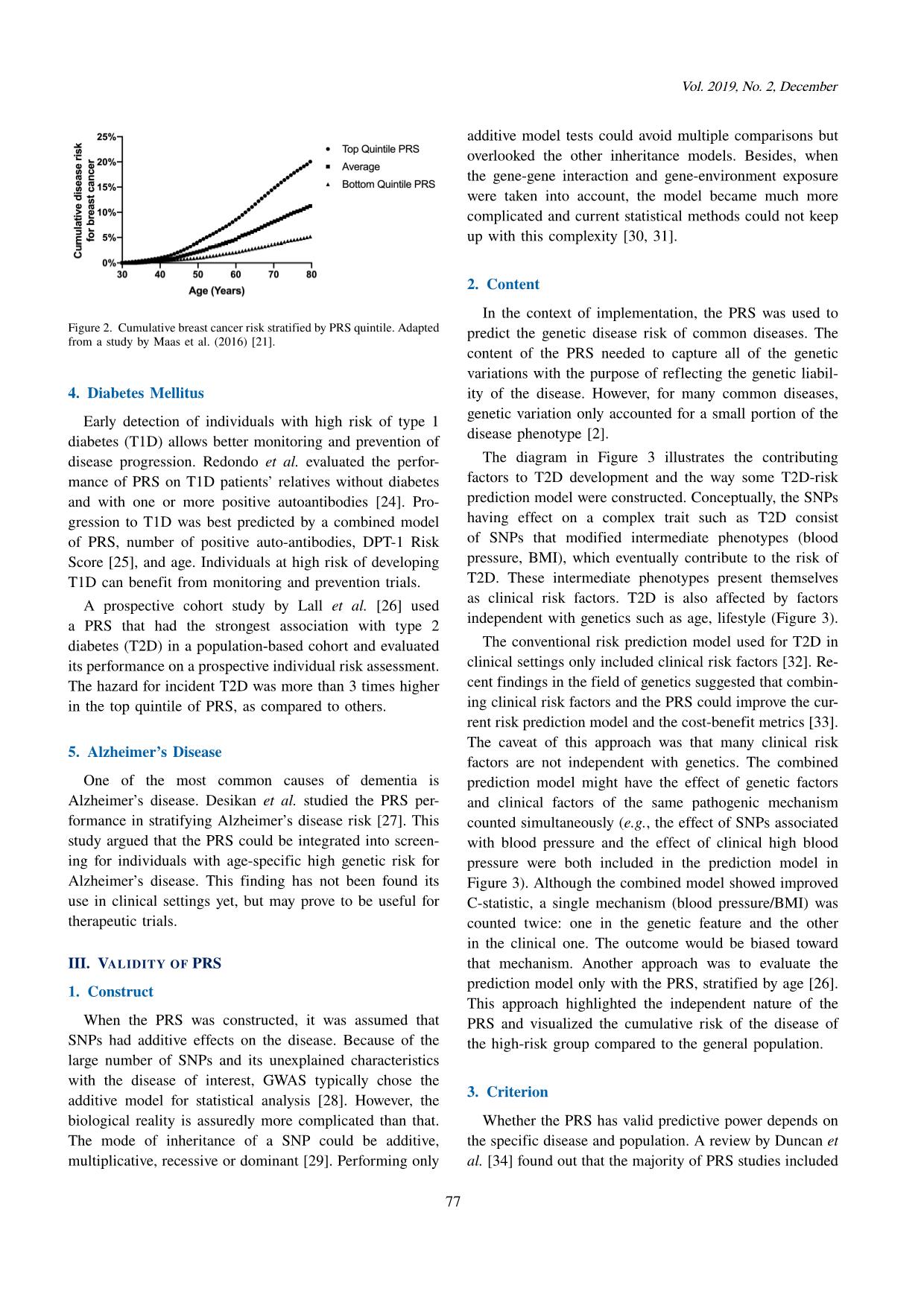
Trang 3
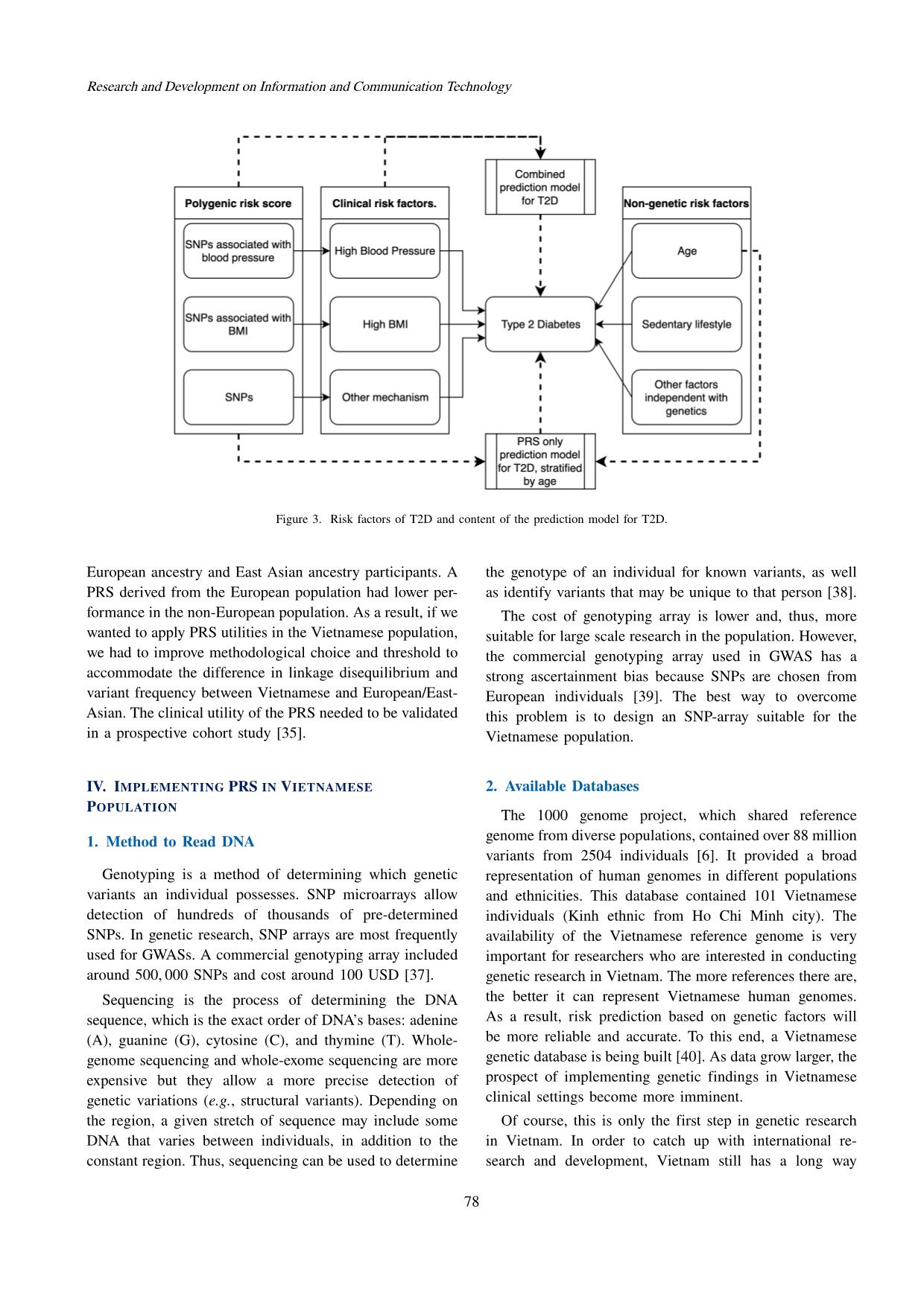
Trang 4
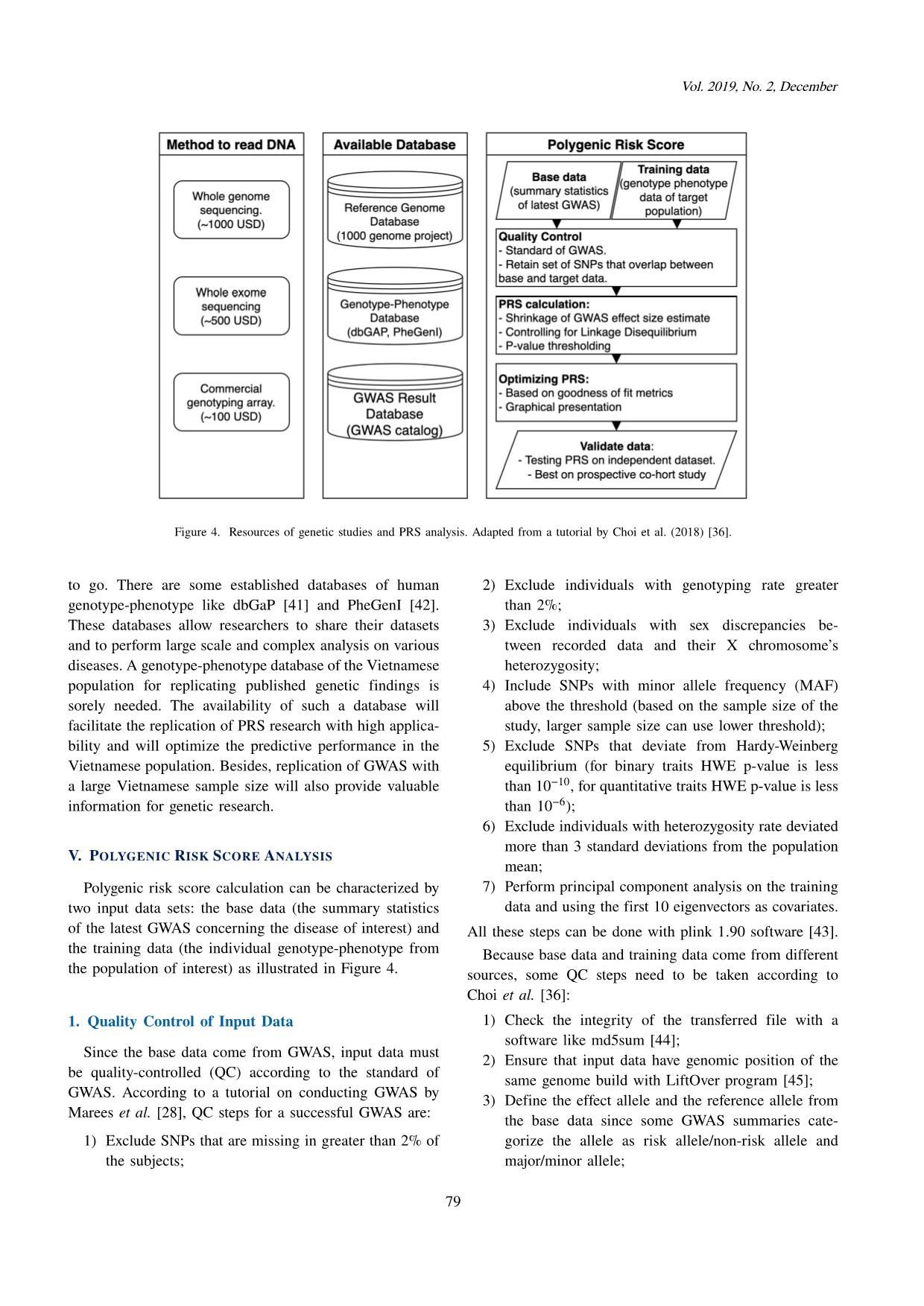
Trang 5
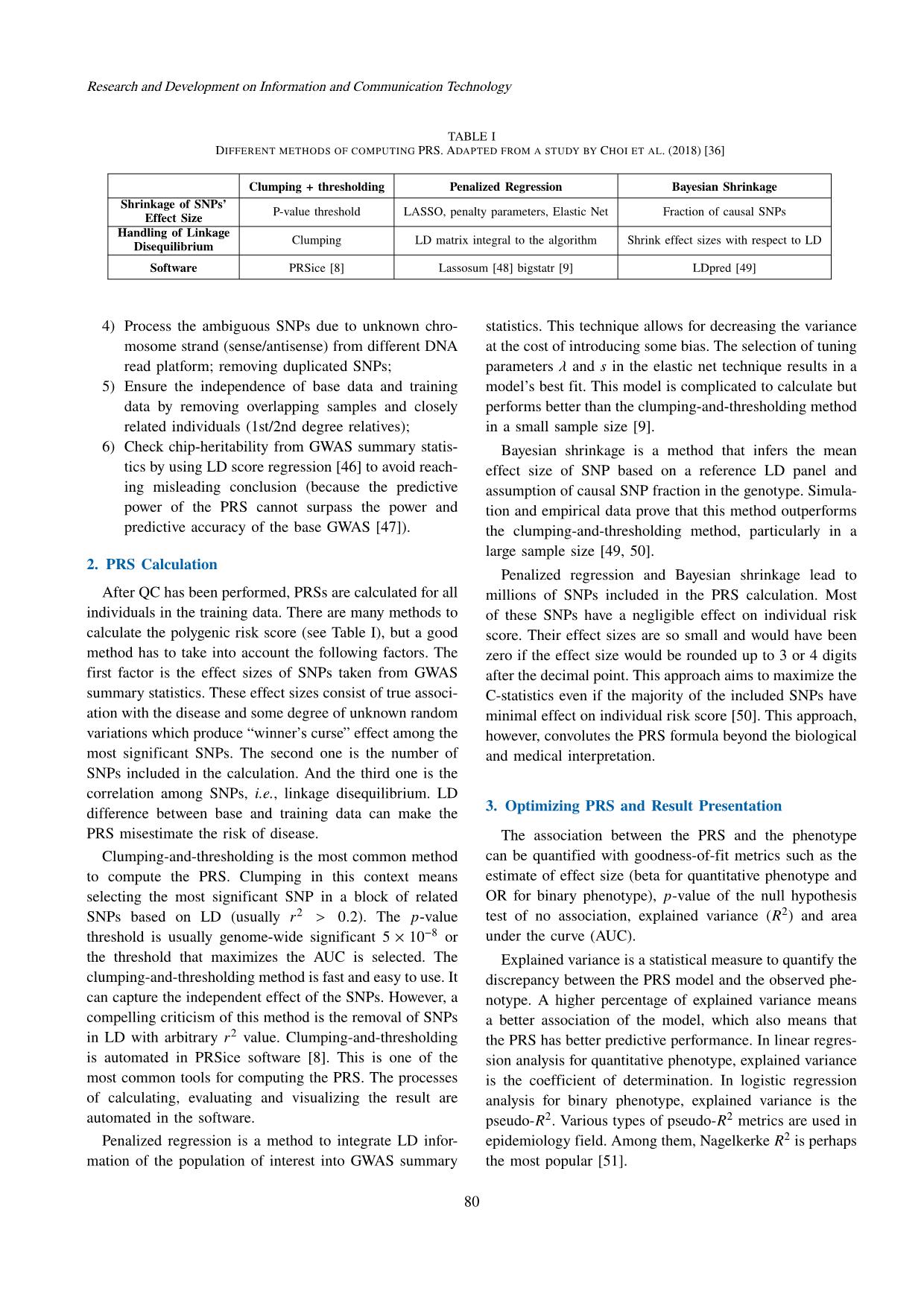
Trang 6
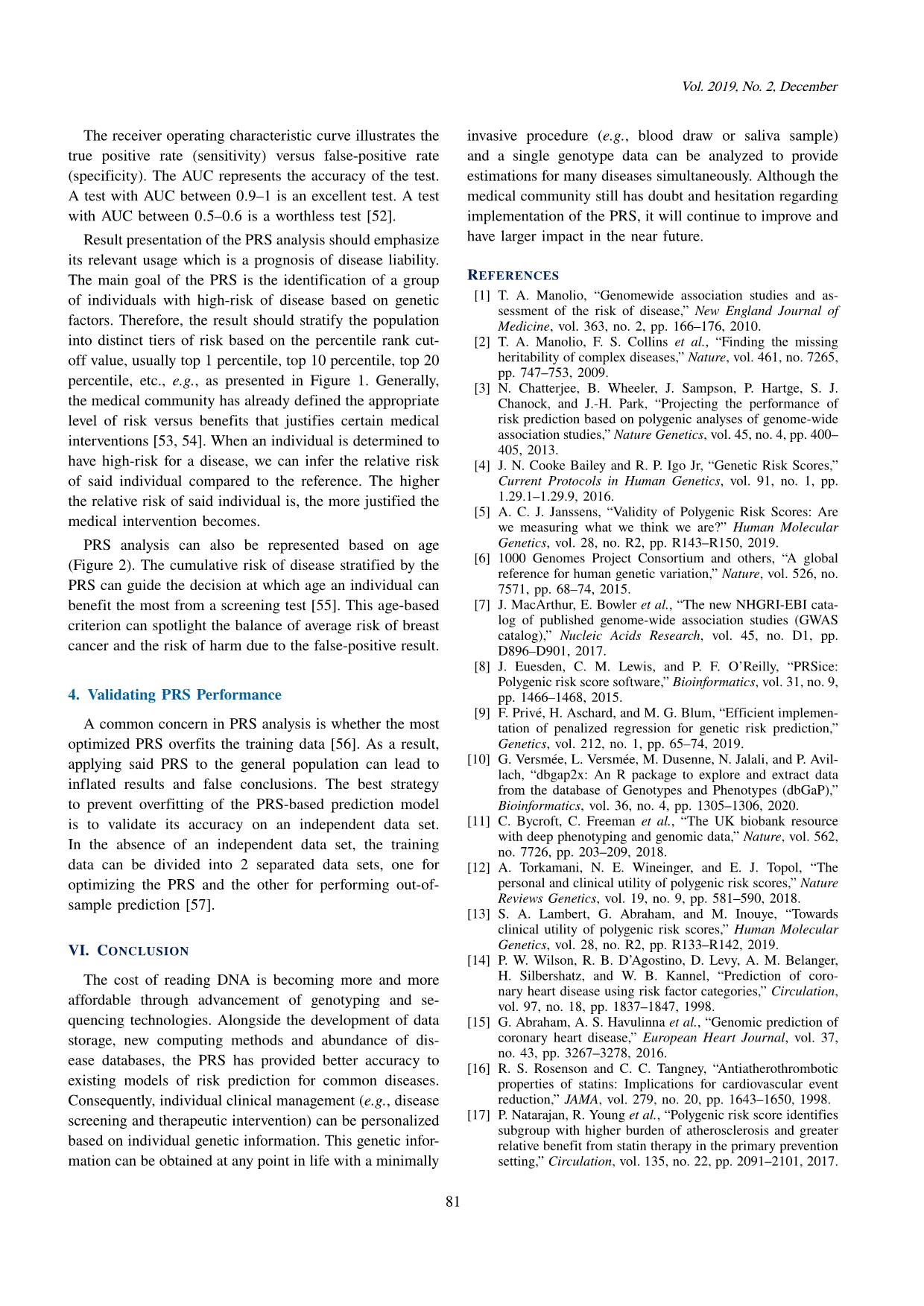
Trang 7
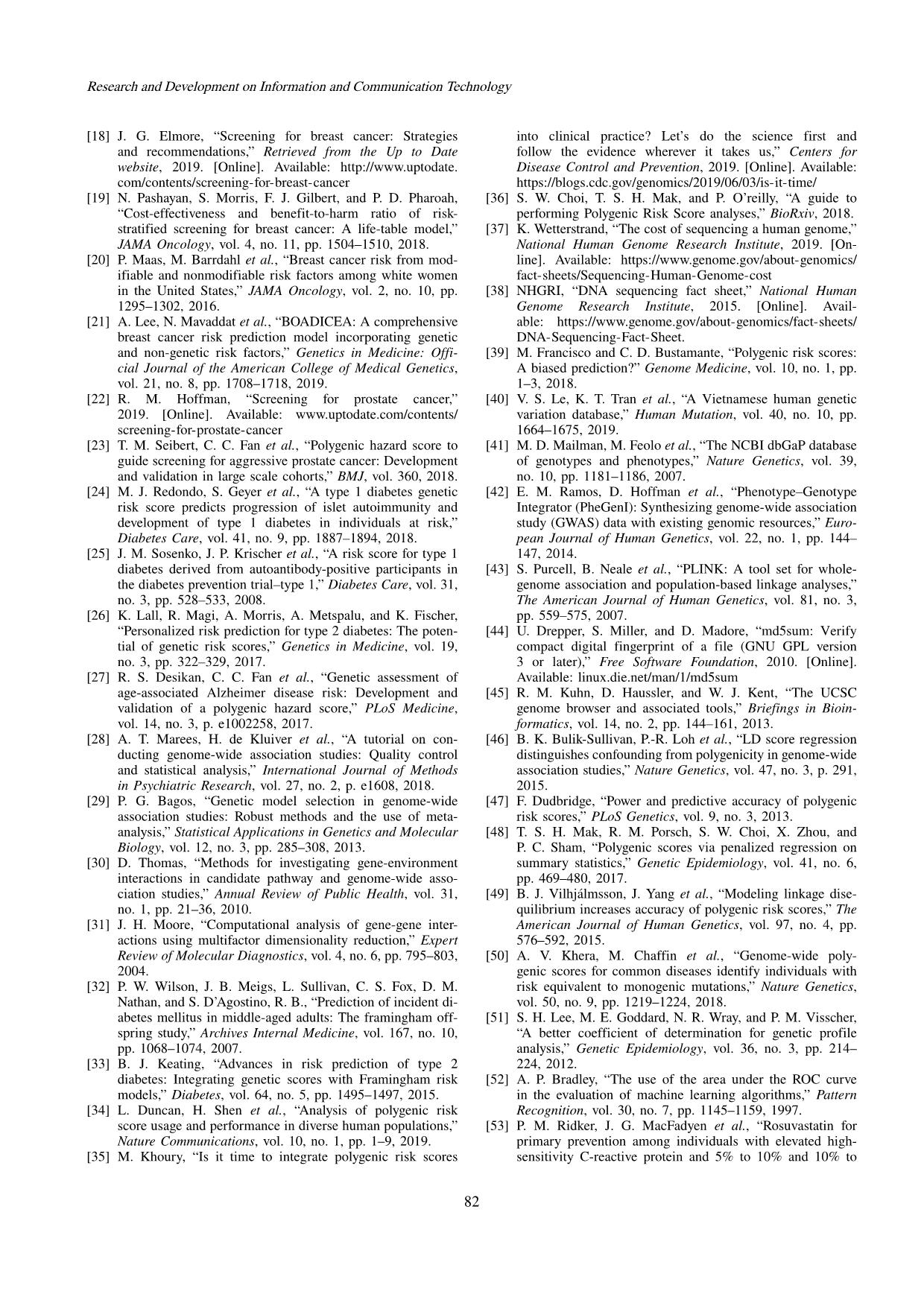
Trang 8
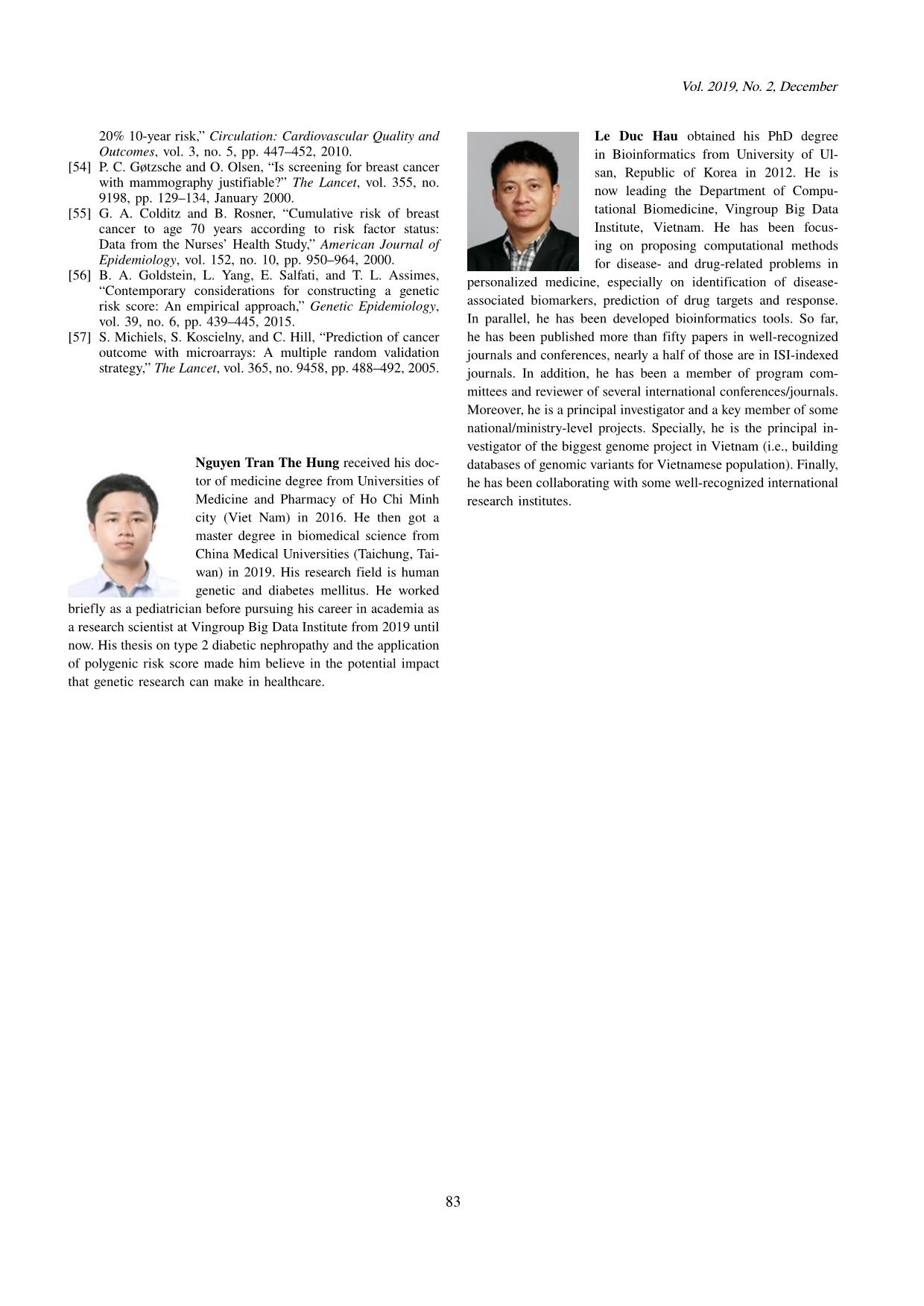
Trang 9
Tóm tắt nội dung tài liệu: Development and implementation of polygenic risk score in Vietnamese population
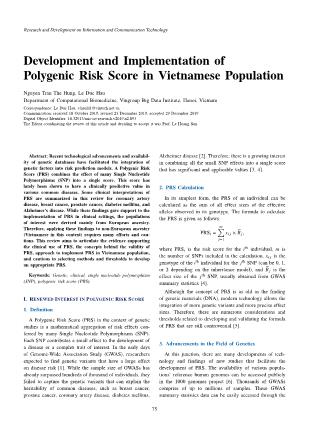
he higher the relative risk of said individual is, the more justified the medical intervention becomes. PRS analysis can also be represented based on age (Figure 2). The cumulative risk of disease stratified by the PRS can guide the decision at which age an individual can benefit the most from a screening test [55]. This age-based criterion can spotlight the balance of average risk of breast cancer and the risk of harm due to the false-positive result. 4. Validating PRS Performance A common concern in PRS analysis is whether the most optimized PRS overfits the training data [56]. As a result, applying said PRS to the general population can lead to inflated results and false conclusions. The best strategy to prevent overfitting of the PRS-based prediction model is to validate its accuracy on an independent data set. In the absence of an independent data set, the training data can be divided into 2 separated data sets, one for optimizing the PRS and the other for performing out-of- sample prediction [57]. VI. CONCLUSION The cost of reading DNA is becoming more and more affordable through advancement of genotyping and se- quencing technologies. Alongside the development of data storage, new computing methods and abundance of dis- ease databases, the PRS has provided better accuracy to existing models of risk prediction for common diseases. Consequently, individual clinical management (e.g., disease screening and therapeutic intervention) can be personalized based on individual genetic information. This genetic infor- mation can be obtained at any point in life with a minimally invasive procedure (e.g., blood draw or saliva sample) and a single genotype data can be analyzed to provide estimations for many diseases simultaneously. Although the medical community still has doubt and hesitation regarding implementation of the PRS, it will continue to improve and have larger impact in the near future. REFERENCES [1] T. A. Manolio, “Genomewide association studies and as- sessment of the risk of disease,” New England Journal of Medicine, vol. 363, no. 2, pp. 166–176, 2010. [2] T. A. Manolio, F. S. Collins et al., “Finding the missing heritability of complex diseases,” Nature, vol. 461, no. 7265, pp. 747–753, 2009. [3] N. Chatterjee, B. Wheeler, J. Sampson, P. Hartge, S. J. Chanock, and J.-H. Park, “Projecting the performance of risk prediction based on polygenic analyses of genome-wide association studies,” Nature Genetics, vol. 45, no. 4, pp. 400– 405, 2013. [4] J. N. Cooke Bailey and R. P. Igo Jr, “Genetic Risk Scores,” Current Protocols in Human Genetics, vol. 91, no. 1, pp. 1.29.1–1.29.9, 2016. [5] A. C. J. Janssens, “Validity of Polygenic Risk Scores: Are we measuring what we think we are?” Human Molecular Genetics, vol. 28, no. R2, pp. R143–R150, 2019. [6] 1000 Genomes Project Consortium and others, “A global reference for human genetic variation,” Nature, vol. 526, no. 7571, pp. 68–74, 2015. [7] J. MacArthur, E. Bowler et al., “The new NHGRI-EBI cata- log of published genome-wide association studies (GWAS catalog),” Nucleic Acids Research, vol. 45, no. D1, pp. D896–D901, 2017. [8] J. Euesden, C. M. Lewis, and P. F. O’Reilly, “PRSice: Polygenic risk score software,” Bioinformatics, vol. 31, no. 9, pp. 1466–1468, 2015. [9] F. Privé, H. Aschard, and M. G. Blum, “Efficient implemen- tation of penalized regression for genetic risk prediction,” Genetics, vol. 212, no. 1, pp. 65–74, 2019. [10] G. Versmée, L. Versmée, M. Dusenne, N. Jalali, and P. Avil- lach, “dbgap2x: An R package to explore and extract data from the database of Genotypes and Phenotypes (dbGaP),” Bioinformatics, vol. 36, no. 4, pp. 1305–1306, 2020. [11] C. Bycroft, C. Freeman et al., “The UK biobank resource with deep phenotyping and genomic data,” Nature, vol. 562, no. 7726, pp. 203–209, 2018. [12] A. Torkamani, N. E. Wineinger, and E. J. Topol, “The personal and clinical utility of polygenic risk scores,” Nature Reviews Genetics, vol. 19, no. 9, pp. 581–590, 2018. [13] S. A. Lambert, G. Abraham, and M. Inouye, “Towards clinical utility of polygenic risk scores,” Human Molecular Genetics, vol. 28, no. R2, pp. R133–R142, 2019. [14] P. W. Wilson, R. B. D’Agostino, D. Levy, A. M. Belanger, H. Silbershatz, and W. B. Kannel, “Prediction of coro- nary heart disease using risk factor categories,” Circulation, vol. 97, no. 18, pp. 1837–1847, 1998. [15] G. Abraham, A. S. Havulinna et al., “Genomic prediction of coronary heart disease,” European Heart Journal, vol. 37, no. 43, pp. 3267–3278, 2016. [16] R. S. Rosenson and C. C. Tangney, “Antiatherothrombotic properties of statins: Implications for cardiovascular event reduction,” JAMA, vol. 279, no. 20, pp. 1643–1650, 1998. [17] P. Natarajan, R. Young et al., “Polygenic risk score identifies subgroup with higher burden of atherosclerosis and greater relative benefit from statin therapy in the primary prevention setting,” Circulation, vol. 135, no. 22, pp. 2091–2101, 2017. 81 Research and Development on Information and Communication Technology [18] J. G. Elmore, “Screening for breast cancer: Strategies and recommendations,” Retrieved from the Up to Date website, 2019. [Online]. Available: com/contents/screening-for-breast-cancer [19] N. Pashayan, S. Morris, F. J. Gilbert, and P. D. Pharoah, “Cost-effectiveness and benefit-to-harm ratio of risk- stratified screening for breast cancer: A life-table model,” JAMA Oncology, vol. 4, no. 11, pp. 1504–1510, 2018. [20] P. Maas, M. Barrdahl et al., “Breast cancer risk from mod- ifiable and nonmodifiable risk factors among white women in the United States,” JAMA Oncology, vol. 2, no. 10, pp. 1295–1302, 2016. [21] A. Lee, N. Mavaddat et al., “BOADICEA: A comprehensive breast cancer risk prediction model incorporating genetic and non-genetic risk factors,” Genetics in Medicine: Offi- cial Journal of the American College of Medical Genetics, vol. 21, no. 8, pp. 1708–1718, 2019. [22] R. M. Hoffman, “Screening for prostate cancer,” 2019. [Online]. Available: www.uptodate.com/contents/ screening-for-prostate-cancer [23] T. M. Seibert, C. C. Fan et al., “Polygenic hazard score to guide screening for aggressive prostate cancer: Development and validation in large scale cohorts,” BMJ, vol. 360, 2018. [24] M. J. Redondo, S. Geyer et al., “A type 1 diabetes genetic risk score predicts progression of islet autoimmunity and development of type 1 diabetes in individuals at risk,” Diabetes Care, vol. 41, no. 9, pp. 1887–1894, 2018. [25] J. M. Sosenko, J. P. Krischer et al., “A risk score for type 1 diabetes derived from autoantibody-positive participants in the diabetes prevention trial–type 1,” Diabetes Care, vol. 31, no. 3, pp. 528–533, 2008. [26] K. Lall, R. Magi, A. Morris, A. Metspalu, and K. Fischer, “Personalized risk prediction for type 2 diabetes: The poten- tial of genetic risk scores,” Genetics in Medicine, vol. 19, no. 3, pp. 322–329, 2017. [27] R. S. Desikan, C. C. Fan et al., “Genetic assessment of age-associated Alzheimer disease risk: Development and validation of a polygenic hazard score,” PLoS Medicine, vol. 14, no. 3, p. e1002258, 2017. [28] A. T. Marees, H. de Kluiver et al., “A tutorial on con- ducting genome-wide association studies: Quality control and statistical analysis,” International Journal of Methods in Psychiatric Research, vol. 27, no. 2, p. e1608, 2018. [29] P. G. Bagos, “Genetic model selection in genome-wide association studies: Robust methods and the use of meta- analysis,” Statistical Applications in Genetics and Molecular Biology, vol. 12, no. 3, pp. 285–308, 2013. [30] D. Thomas, “Methods for investigating gene-environment interactions in candidate pathway and genome-wide asso- ciation studies,” Annual Review of Public Health, vol. 31, no. 1, pp. 21–36, 2010. [31] J. H. Moore, “Computational analysis of gene-gene inter- actions using multifactor dimensionality reduction,” Expert Review of Molecular Diagnostics, vol. 4, no. 6, pp. 795–803, 2004. [32] P. W. Wilson, J. B. Meigs, L. Sullivan, C. S. Fox, D. M. Nathan, and S. D’Agostino, R. B., “Prediction of incident di- abetes mellitus in middle-aged adults: The framingham off- spring study,” Archives Internal Medicine, vol. 167, no. 10, pp. 1068–1074, 2007. [33] B. J. Keating, “Advances in risk prediction of type 2 diabetes: Integrating genetic scores with Framingham risk models,” Diabetes, vol. 64, no. 5, pp. 1495–1497, 2015. [34] L. Duncan, H. Shen et al., “Analysis of polygenic risk score usage and performance in diverse human populations,” Nature Communications, vol. 10, no. 1, pp. 1–9, 2019. [35] M. Khoury, “Is it time to integrate polygenic risk scores into clinical practice? Let’s do the science first and follow the evidence wherever it takes us,” Centers for Disease Control and Prevention, 2019. [Online]. Available: https://blogs.cdc.gov/genomics/2019/06/03/is-it-time/ [36] S. W. Choi, T. S. H. Mak, and P. O’reilly, “A guide to performing Polygenic Risk Score analyses,” BioRxiv, 2018. [37] K. Wetterstrand, “The cost of sequencing a human genome,” National Human Genome Research Institute, 2019. [On- line]. Available: https://www.genome.gov/about-genomics/ fact-sheets/Sequencing-Human-Genome-cost [38] NHGRI, “DNA sequencing fact sheet,” National Human Genome Research Institute, 2015. [Online]. Avail- able: https://www.genome.gov/about-genomics/fact-sheets/ DNA-Sequencing-Fact-Sheet. [39] M. Francisco and C. D. Bustamante, “Polygenic risk scores: A biased prediction?” Genome Medicine, vol. 10, no. 1, pp. 1–3, 2018. [40] V. S. Le, K. T. Tran et al., “A Vietnamese human genetic variation database,” Human Mutation, vol. 40, no. 10, pp. 1664–1675, 2019. [41] M. D. Mailman, M. Feolo et al., “The NCBI dbGaP database of genotypes and phenotypes,” Nature Genetics, vol. 39, no. 10, pp. 1181–1186, 2007. [42] E. M. Ramos, D. Hoffman et al., “Phenotype–Genotype Integrator (PheGenI): Synthesizing genome-wide association study (GWAS) data with existing genomic resources,” Euro- pean Journal of Human Genetics, vol. 22, no. 1, pp. 144– 147, 2014. [43] S. Purcell, B. Neale et al., “PLINK: A tool set for whole- genome association and population-based linkage analyses,” The American Journal of Human Genetics, vol. 81, no. 3, pp. 559–575, 2007. [44] U. Drepper, S. Miller, and D. Madore, “md5sum: Verify compact digital fingerprint of a file (GNU GPL version 3 or later),” Free Software Foundation, 2010. [Online]. Available: linux.die.net/man/1/md5sum [45] R. M. Kuhn, D. Haussler, and W. J. Kent, “The UCSC genome browser and associated tools,” Briefings in Bioin- formatics, vol. 14, no. 2, pp. 144–161, 2013. [46] B. K. Bulik-Sullivan, P.-R. Loh et al., “LD score regression distinguishes confounding from polygenicity in genome-wide association studies,” Nature Genetics, vol. 47, no. 3, p. 291, 2015. [47] F. Dudbridge, “Power and predictive accuracy of polygenic risk scores,” PLoS Genetics, vol. 9, no. 3, 2013. [48] T. S. H. Mak, R. M. Porsch, S. W. Choi, X. Zhou, and P. C. Sham, “Polygenic scores via penalized regression on summary statistics,” Genetic Epidemiology, vol. 41, no. 6, pp. 469–480, 2017. [49] B. J. Vilhjálmsson, J. Yang et al., “Modeling linkage dise- quilibrium increases accuracy of polygenic risk scores,” The American Journal of Human Genetics, vol. 97, no. 4, pp. 576–592, 2015. [50] A. V. Khera, M. Chaffin et al., “Genome-wide poly- genic scores for common diseases identify individuals with risk equivalent to monogenic mutations,” Nature Genetics, vol. 50, no. 9, pp. 1219–1224, 2018. [51] S. H. Lee, M. E. Goddard, N. R. Wray, and P. M. Visscher, “A better coefficient of determination for genetic profile analysis,” Genetic Epidemiology, vol. 36, no. 3, pp. 214– 224, 2012. [52] A. P. Bradley, “The use of the area under the ROC curve in the evaluation of machine learning algorithms,” Pattern Recognition, vol. 30, no. 7, pp. 1145–1159, 1997. [53] P. M. Ridker, J. G. MacFadyen et al., “Rosuvastatin for primary prevention among individuals with elevated high- sensitivity C-reactive protein and 5% to 10% and 10% to 82 Vol. 2019, No. 2, December 20% 10-year risk,” Circulation: Cardiovascular Quality and Outcomes, vol. 3, no. 5, pp. 447–452, 2010. [54] P. C. Gøtzsche and O. Olsen, “Is screening for breast cancer with mammography justifiable?” The Lancet, vol. 355, no. 9198, pp. 129–134, January 2000. [55] G. A. Colditz and B. Rosner, “Cumulative risk of breast cancer to age 70 years according to risk factor status: Data from the Nurses’ Health Study,” American Journal of Epidemiology, vol. 152, no. 10, pp. 950–964, 2000. [56] B. A. Goldstein, L. Yang, E. Salfati, and T. L. Assimes, “Contemporary considerations for constructing a genetic risk score: An empirical approach,” Genetic Epidemiology, vol. 39, no. 6, pp. 439–445, 2015. [57] S. Michiels, S. Koscielny, and C. Hill, “Prediction of cancer outcome with microarrays: A multiple random validation strategy,” The Lancet, vol. 365, no. 9458, pp. 488–492, 2005. Nguyen Tran The Hung received his doc- tor of medicine degree from Universities of Medicine and Pharmacy of Ho Chi Minh city (Viet Nam) in 2016. He then got a master degree in biomedical science from China Medical Universities (Taichung, Tai- wan) in 2019. His research field is human genetic and diabetes mellitus. He worked briefly as a pediatrician before pursuing his career in academia as a research scientist at Vingroup Big Data Institute from 2019 until now. His thesis on type 2 diabetic nephropathy and the application of polygenic risk score made him believe in the potential impact that genetic research can make in healthcare. Le Duc Hau obtained his PhD degree in Bioinformatics from University of Ul- san, Republic of Korea in 2012. He is now leading the Department of Compu- tational Biomedicine, Vingroup Big Data Institute, Vietnam. He has been focus- ing on proposing computational methods for disease- and drug-related problems in personalized medicine, especially on identification of disease- associated biomarkers, prediction of drug targets and response. In parallel, he has been developed bioinformatics tools. So far, he has been published more than fifty papers in well-recognized journals and conferences, nearly a half of those are in ISI-indexed journals. In addition, he has been a member of program com- mittees and reviewer of several international conferences/journals. Moreover, he is a principal investigator and a key member of some national/ministry-level projects. Specially, he is the principal in- vestigator of the biggest genome project in Vietnam (i.e., building databases of genomic variants for Vietnamese population). Finally, he has been collaborating with some well-recognized international research institutes. 83
File đính kèm:
development_and_implementation_of_polygenic_risk_score_in_vi.pdf