Computational personalized medicine in cancer research in the-omics data era
Abstract: Omics data (e.g., genomics, transcriptomics, proteomics, epigenomics, etc . . . ) generated from high-Throughput next-generation sequencers in the big human genome, and cancer genome projects have changed the way to study personalized medicine. In the future, personalized medicine will not be limited to diagnosis and treatment based on a few known disease-associated mutations on some genes, but will rely on whole molecular characteristics of patients by integrating their –omics data. In this study, we draw a big picture of personalized medicine research in cancer research of the –omics data era, including –omics databases, challenges of data fusion to solve two major problems in personalized medicine, i.e., personalized diagnosis and treatment. These problems are approached as patient stratification and drug response prediction based on the –omics data by computational methods
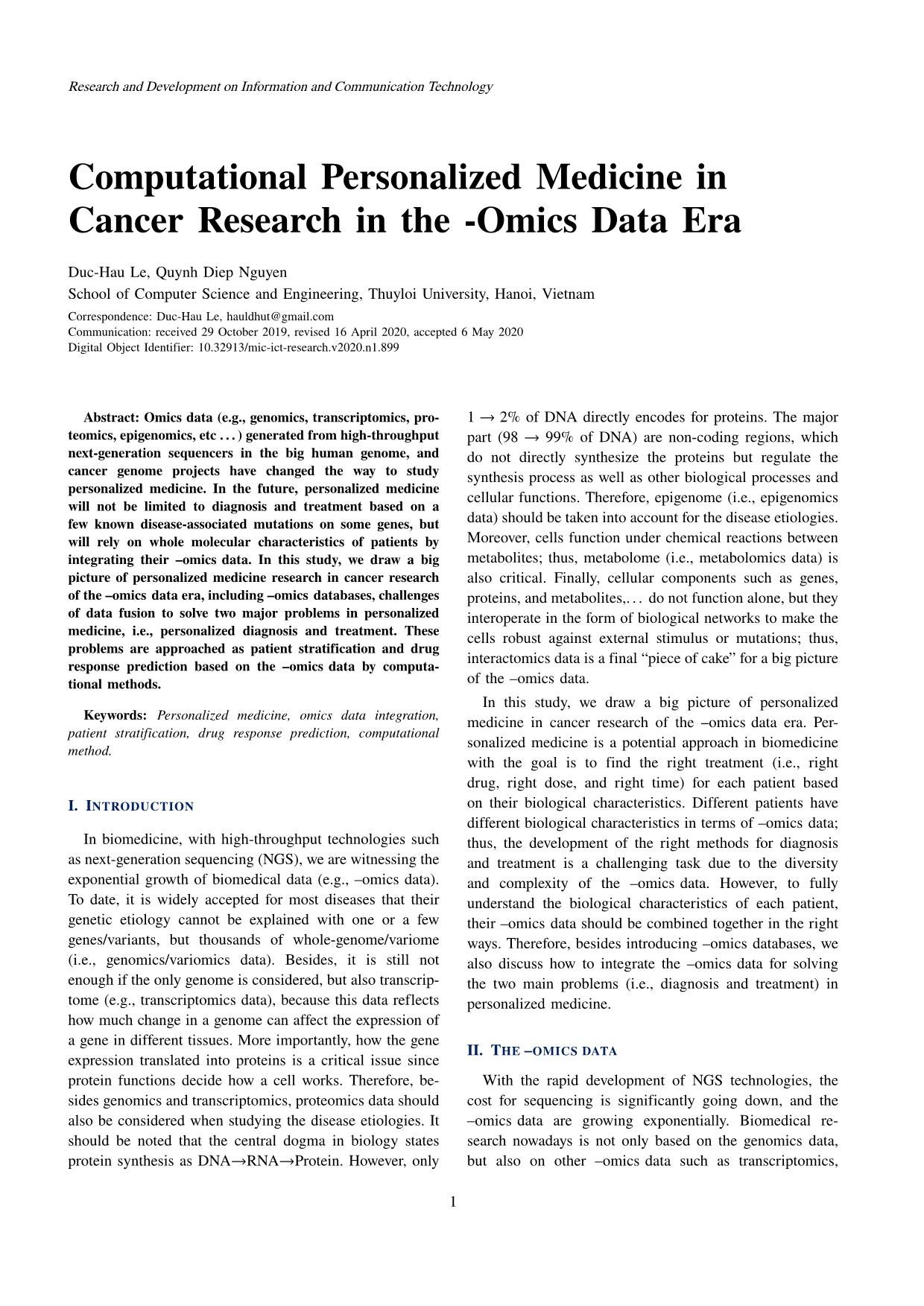
Trang 1
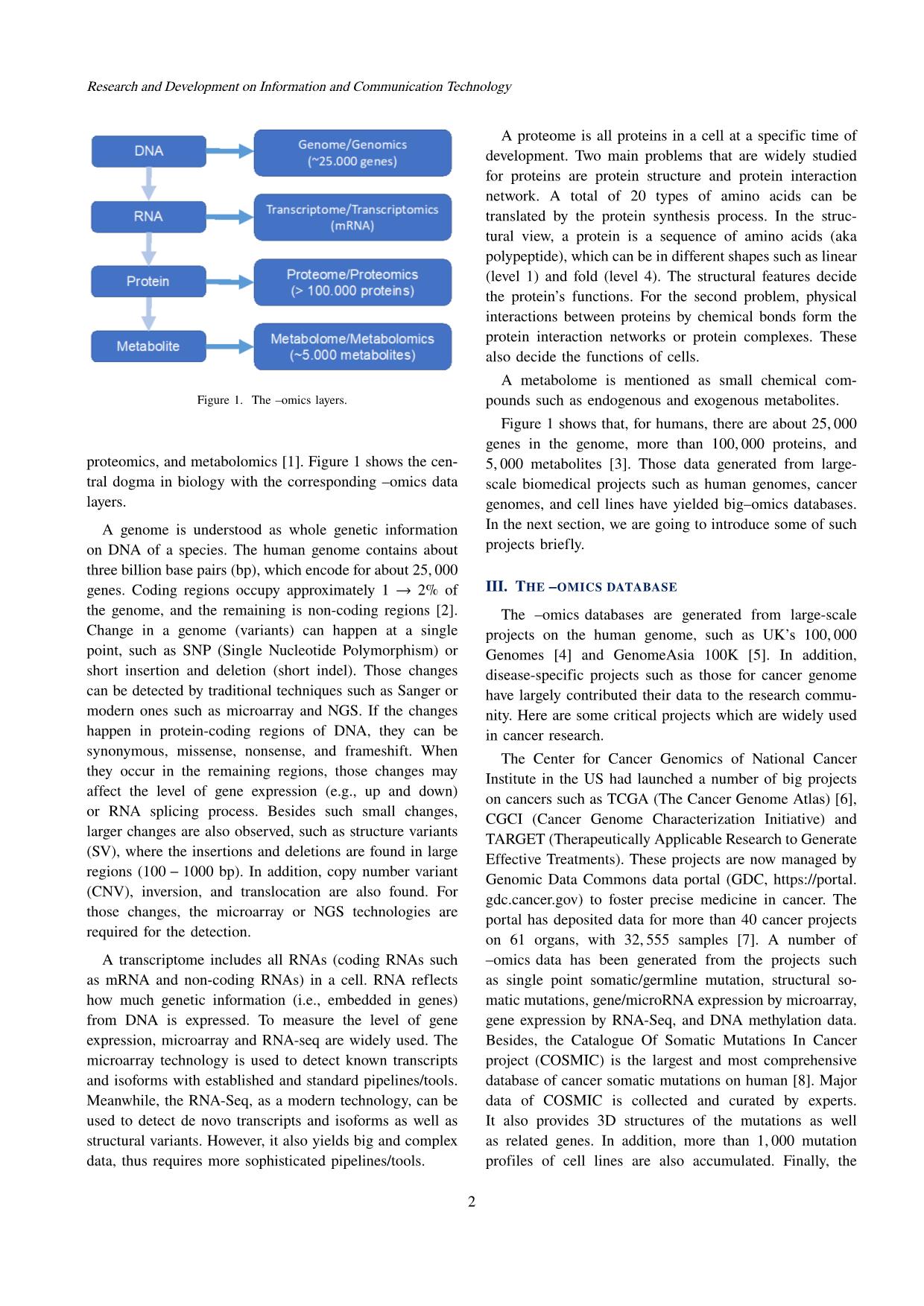
Trang 2
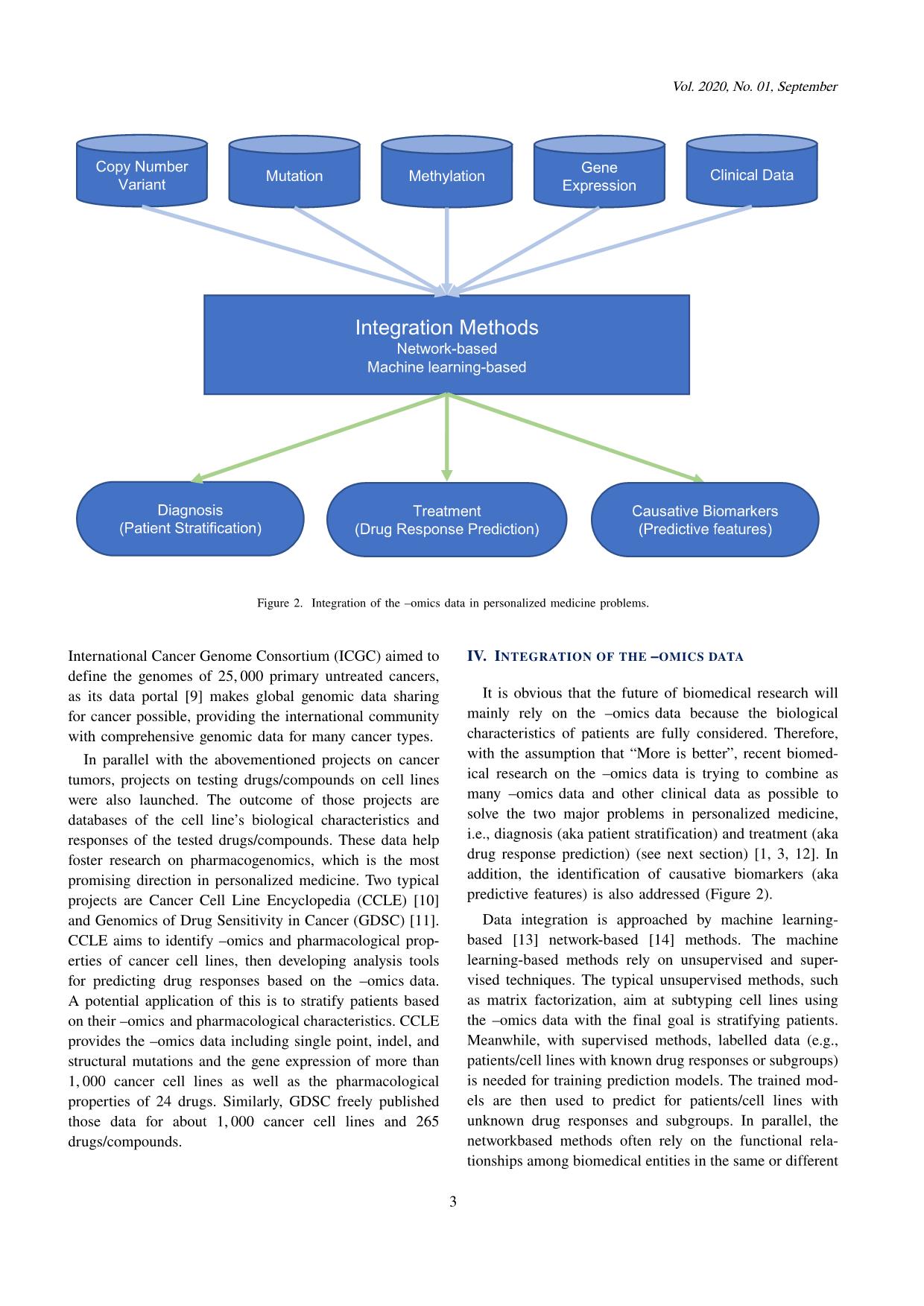
Trang 3
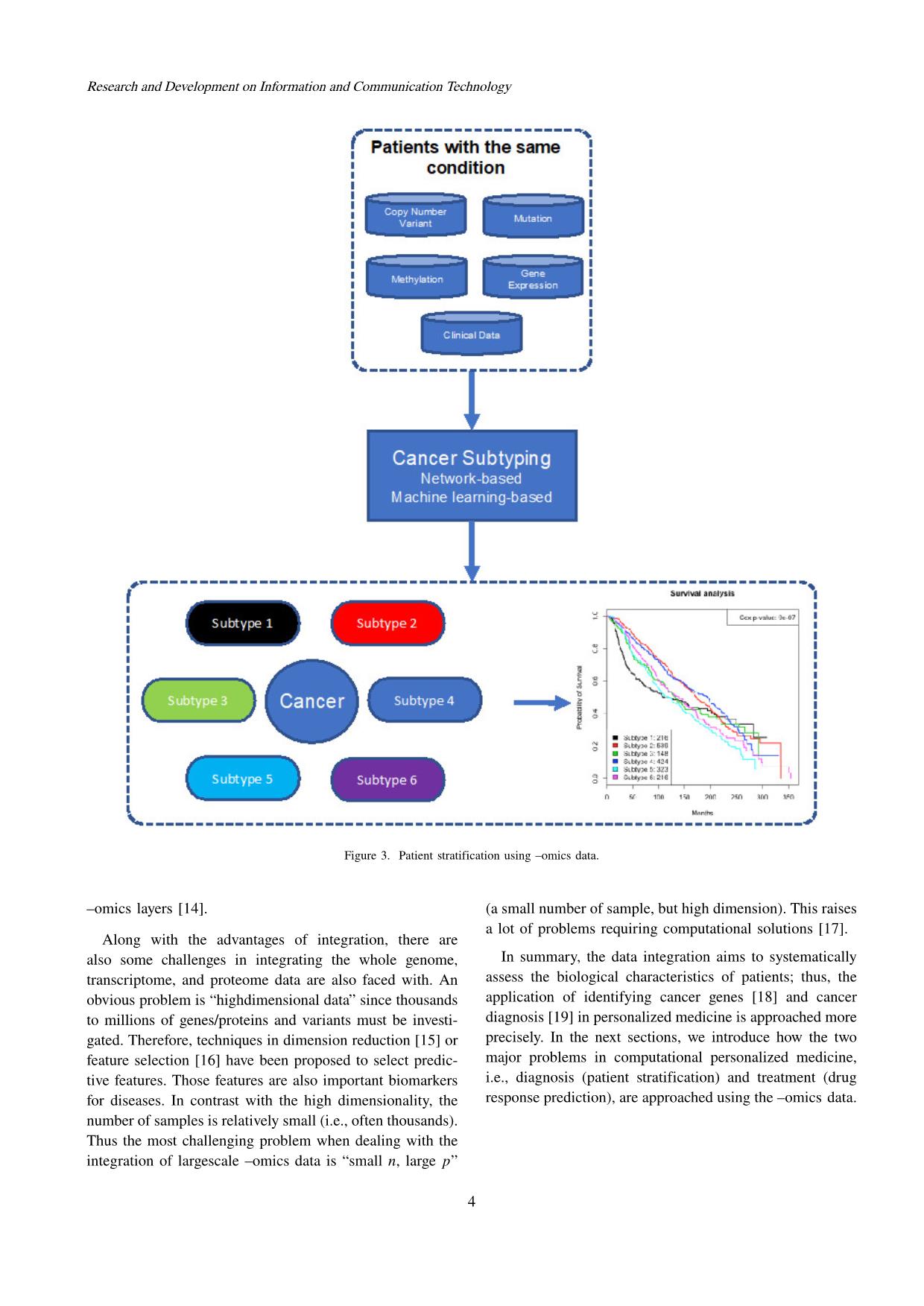
Trang 4
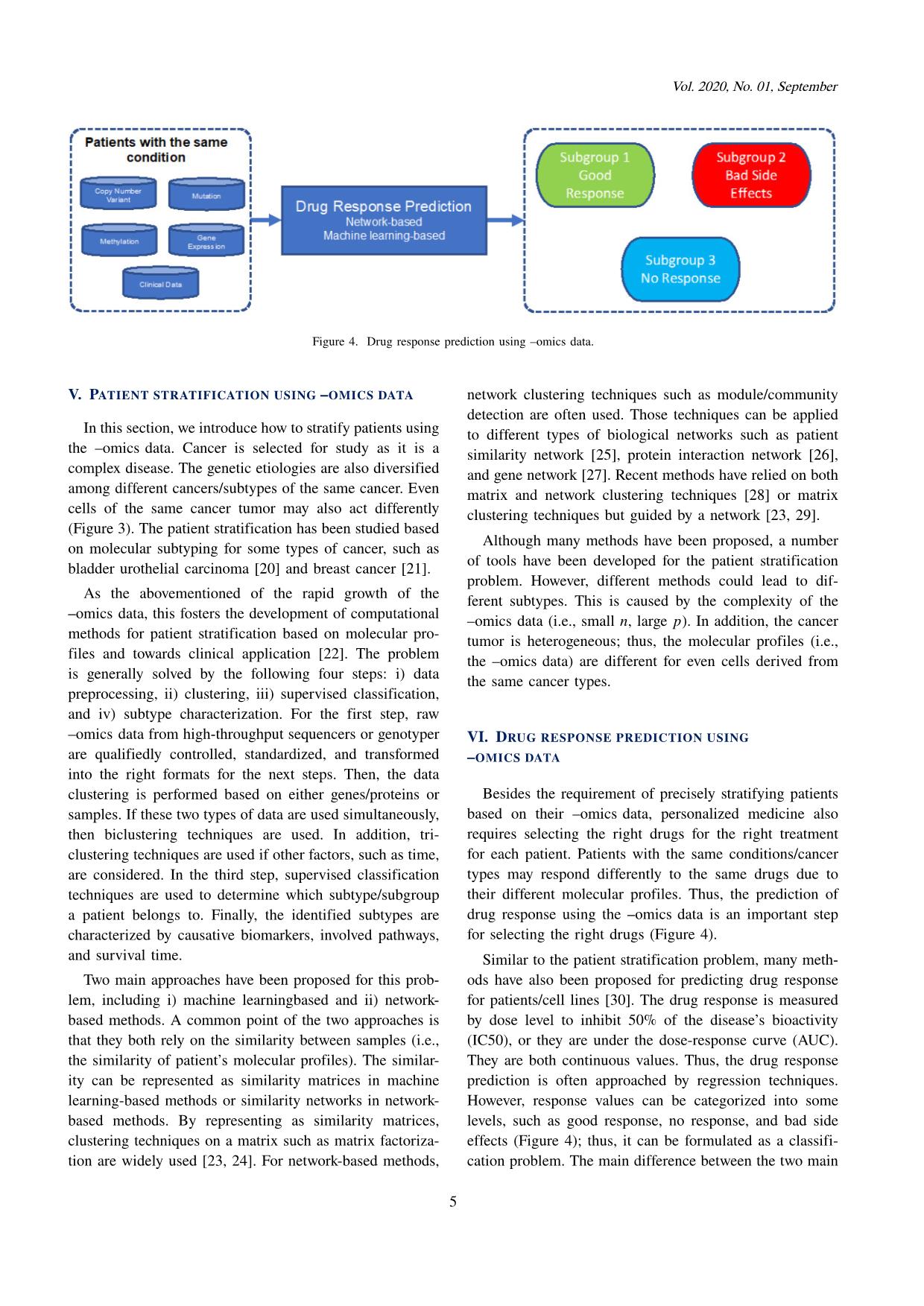
Trang 5
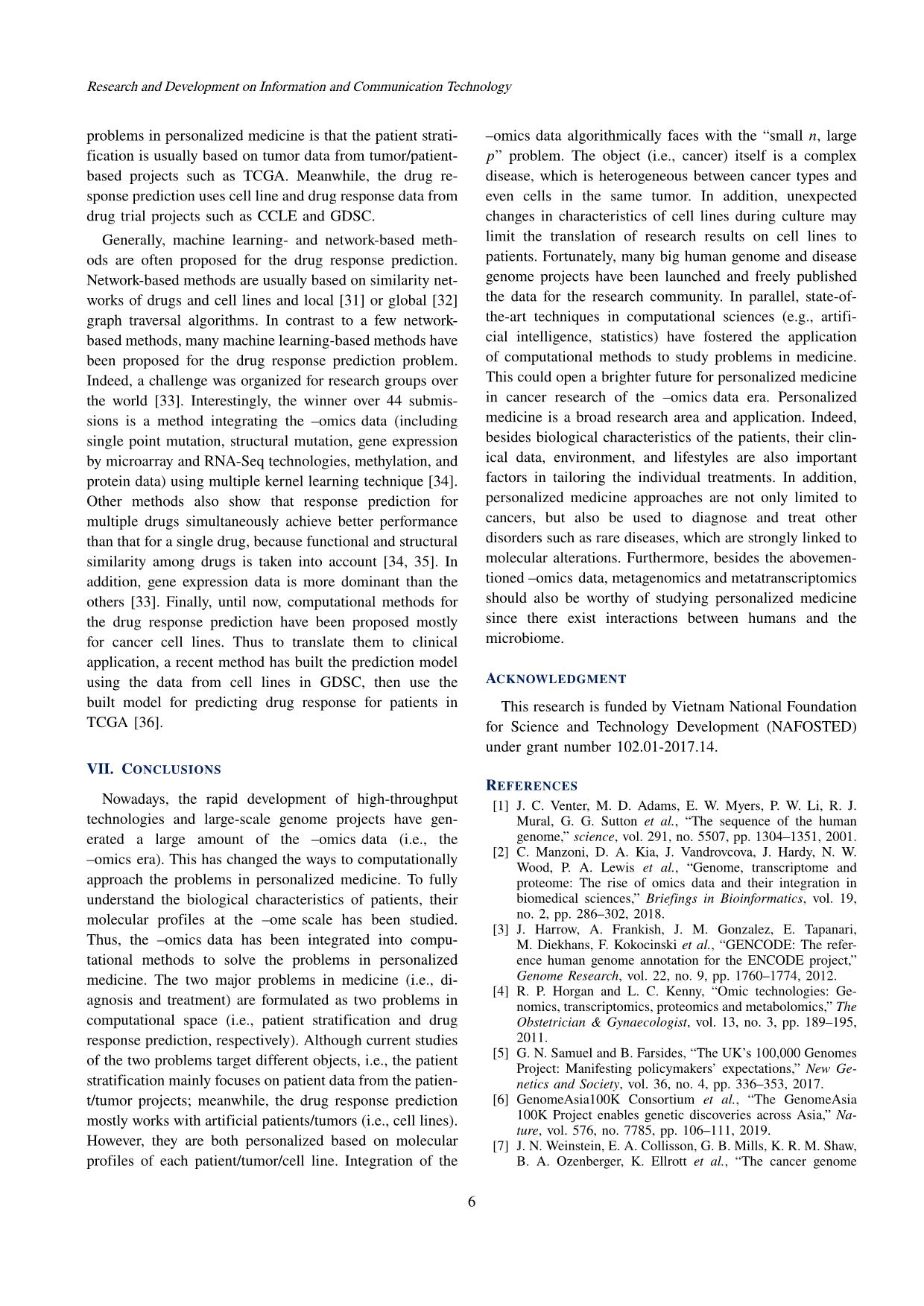
Trang 6
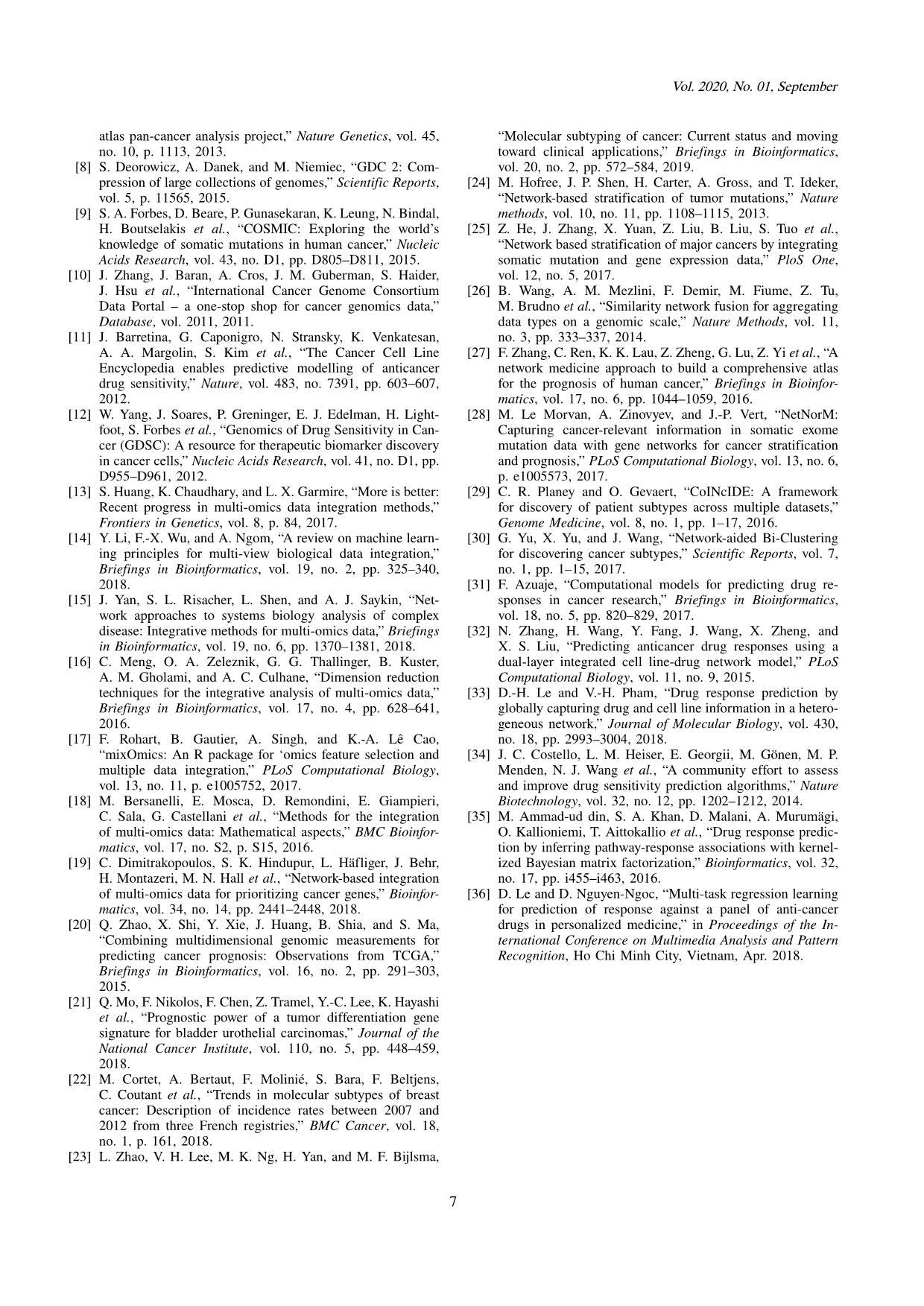
Trang 7
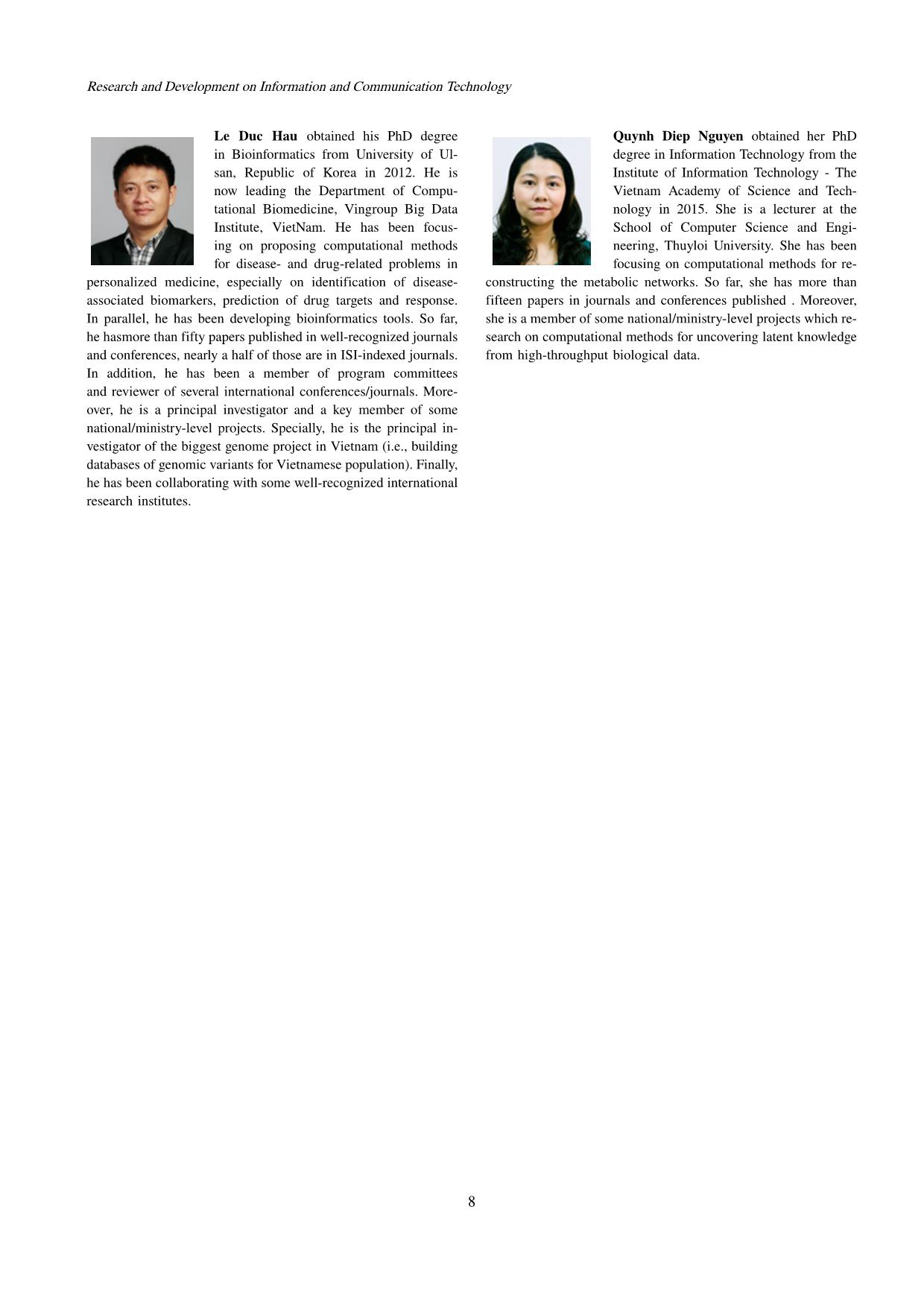
Trang 8
Tóm tắt nội dung tài liệu: Computational personalized medicine in cancer research in the-omics data era
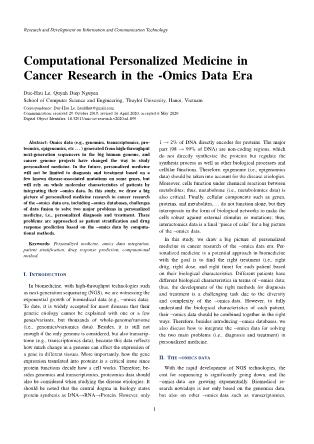
rent molecular profiles. Thus, the prediction of drug response using the –omics data is an important step for selecting the right drugs (Figure 4). Similar to the patient stratification problem, many meth- ods have also been proposed for predicting drug response for patients/cell lines [30]. The drug response is measured by dose level to inhibit 50% of the disease’s bioactivity (IC50), or they are under the dose-response curve (AUC). They are both continuous values. Thus, the drug response prediction is often approached by regression techniques. However, response values can be categorized into some levels, such as good response, no response, and bad side effects (Figure 4); thus, it can be formulated as a classifi- cation problem. The main difference between the two main 5 Research and Development on Information and Communication Technology problems in personalized medicine is that the patient strati- fication is usually based on tumor data from tumor/patient- based projects such as TCGA. Meanwhile, the drug re- sponse prediction uses cell line and drug response data from drug trial projects such as CCLE and GDSC. Generally, machine learning- and network-based meth- ods are often proposed for the drug response prediction. Network-based methods are usually based on similarity net- works of drugs and cell lines and local [31] or global [32] graph traversal algorithms. In contrast to a few network- based methods, many machine learning-based methods have been proposed for the drug response prediction problem. Indeed, a challenge was organized for research groups over the world [33]. Interestingly, the winner over 44 submis- sions is a method integrating the –omics data (including single point mutation, structural mutation, gene expression by microarray and RNA-Seq technologies, methylation, and protein data) using multiple kernel learning technique [34]. Other methods also show that response prediction for multiple drugs simultaneously achieve better performance than that for a single drug, because functional and structural similarity among drugs is taken into account [34, 35]. In addition, gene expression data is more dominant than the others [33]. Finally, until now, computational methods for the drug response prediction have been proposed mostly for cancer cell lines. Thus to translate them to clinical application, a recent method has built the prediction model using the data from cell lines in GDSC, then use the built model for predicting drug response for patients in TCGA [36]. VII. CONCLUSIONS Nowadays, the rapid development of high-throughput technologies and large-scale genome projects have gen- erated a large amount of the –omics data (i.e., the –omics era). This has changed the ways to computationally approach the problems in personalized medicine. To fully understand the biological characteristics of patients, their molecular profiles at the –ome scale has been studied. Thus, the –omics data has been integrated into compu- tational methods to solve the problems in personalized medicine. The two major problems in medicine (i.e., di- agnosis and treatment) are formulated as two problems in computational space (i.e., patient stratification and drug response prediction, respectively). Although current studies of the two problems target different objects, i.e., the patient stratification mainly focuses on patient data from the patien- t/tumor projects; meanwhile, the drug response prediction mostly works with artificial patients/tumors (i.e., cell lines). However, they are both personalized based on molecular profiles of each patient/tumor/cell line. Integration of the –omics data algorithmically faces with the “small 𝑛, large 𝑝” problem. The object (i.e., cancer) itself is a complex disease, which is heterogeneous between cancer types and even cells in the same tumor. In addition, unexpected changes in characteristics of cell lines during culture may limit the translation of research results on cell lines to patients. Fortunately, many big human genome and disease genome projects have been launched and freely published the data for the research community. In parallel, state-of- the-art techniques in computational sciences (e.g., artifi- cial intelligence, statistics) have fostered the application of computational methods to study problems in medicine. This could open a brighter future for personalized medicine in cancer research of the –omics data era. Personalized medicine is a broad research area and application. Indeed, besides biological characteristics of the patients, their clin- ical data, environment, and lifestyles are also important factors in tailoring the individual treatments. In addition, personalized medicine approaches are not only limited to cancers, but also be used to diagnose and treat other disorders such as rare diseases, which are strongly linked to molecular alterations. Furthermore, besides the abovemen- tioned –omics data, metagenomics and metatranscriptomics should also be worthy of studying personalized medicine since there exist interactions between humans and the microbiome. ACKNOWLEDGMENT This research is funded by Vietnam National Foundation for Science and Technology Development (NAFOSTED) under grant number 102.01-2017.14. REFERENCES [1] J. C. Venter, M. D. Adams, E. W. Myers, P. W. Li, R. J. Mural, G. G. Sutton et al., “The sequence of the human genome,” science, vol. 291, no. 5507, pp. 1304–1351, 2001. [2] C. Manzoni, D. A. Kia, J. Vandrovcova, J. Hardy, N. W. Wood, P. A. Lewis et al., “Genome, transcriptome and proteome: The rise of omics data and their integration in biomedical sciences,” Briefings in Bioinformatics, vol. 19, no. 2, pp. 286–302, 2018. [3] J. Harrow, A. Frankish, J. M. Gonzalez, E. Tapanari, M. Diekhans, F. Kokocinski et al., “GENCODE: The refer- ence human genome annotation for the ENCODE project,” Genome Research, vol. 22, no. 9, pp. 1760–1774, 2012. [4] R. P. Horgan and L. C. Kenny, “Omic technologies: Ge- nomics, transcriptomics, proteomics and metabolomics,” The Obstetrician & Gynaecologist, vol. 13, no. 3, pp. 189–195, 2011. [5] G. N. Samuel and B. Farsides, “The UK’s 100,000 Genomes Project: Manifesting policymakers’ expectations,” New Ge- netics and Society, vol. 36, no. 4, pp. 336–353, 2017. [6] GenomeAsia100K Consortium et al., “The GenomeAsia 100K Project enables genetic discoveries across Asia,” Na- ture, vol. 576, no. 7785, pp. 106–111, 2019. [7] J. N. Weinstein, E. A. Collisson, G. B. Mills, K. R. M. Shaw, B. A. Ozenberger, K. Ellrott et al., “The cancer genome 6 Vol. 2020, No. 01, September atlas pan-cancer analysis project,” Nature Genetics, vol. 45, no. 10, p. 1113, 2013. [8] S. Deorowicz, A. Danek, and M. Niemiec, “GDC 2: Com- pression of large collections of genomes,” Scientific Reports, vol. 5, p. 11565, 2015. [9] S. A. Forbes, D. Beare, P. Gunasekaran, K. Leung, N. Bindal, H. Boutselakis et al., “COSMIC: Exploring the world’s knowledge of somatic mutations in human cancer,” Nucleic Acids Research, vol. 43, no. D1, pp. D805–D811, 2015. [10] J. Zhang, J. Baran, A. Cros, J. M. Guberman, S. Haider, J. Hsu et al., “International Cancer Genome Consortium Data Portal – a one-stop shop for cancer genomics data,” Database, vol. 2011, 2011. [11] J. Barretina, G. Caponigro, N. Stransky, K. Venkatesan, A. A. Margolin, S. Kim et al., “The Cancer Cell Line Encyclopedia enables predictive modelling of anticancer drug sensitivity,” Nature, vol. 483, no. 7391, pp. 603–607, 2012. [12] W. Yang, J. Soares, P. Greninger, E. J. Edelman, H. Light- foot, S. Forbes et al., “Genomics of Drug Sensitivity in Can- cer (GDSC): A resource for therapeutic biomarker discovery in cancer cells,” Nucleic Acids Research, vol. 41, no. D1, pp. D955–D961, 2012. [13] S. Huang, K. Chaudhary, and L. X. Garmire, “More is better: Recent progress in multi-omics data integration methods,” Frontiers in Genetics, vol. 8, p. 84, 2017. [14] Y. Li, F.-X. Wu, and A. Ngom, “A review on machine learn- ing principles for multi-view biological data integration,” Briefings in Bioinformatics, vol. 19, no. 2, pp. 325–340, 2018. [15] J. Yan, S. L. Risacher, L. Shen, and A. J. Saykin, “Net- work approaches to systems biology analysis of complex disease: Integrative methods for multi-omics data,” Briefings in Bioinformatics, vol. 19, no. 6, pp. 1370–1381, 2018. [16] C. Meng, O. A. Zeleznik, G. G. Thallinger, B. Kuster, A. M. Gholami, and A. C. Culhane, “Dimension reduction techniques for the integrative analysis of multi-omics data,” Briefings in Bioinformatics, vol. 17, no. 4, pp. 628–641, 2016. [17] F. Rohart, B. Gautier, A. Singh, and K.-A. Lê Cao, “mixOmics: An R package for ‘omics feature selection and multiple data integration,” PLoS Computational Biology, vol. 13, no. 11, p. e1005752, 2017. [18] M. Bersanelli, E. Mosca, D. Remondini, E. Giampieri, C. Sala, G. Castellani et al., “Methods for the integration of multi-omics data: Mathematical aspects,” BMC Bioinfor- matics, vol. 17, no. S2, p. S15, 2016. [19] C. Dimitrakopoulos, S. K. Hindupur, L. Ha¨fliger, J. Behr, H. Montazeri, M. N. Hall et al., “Network-based integration of multi-omics data for prioritizing cancer genes,” Bioinfor- matics, vol. 34, no. 14, pp. 2441–2448, 2018. [20] Q. Zhao, X. Shi, Y. Xie, J. Huang, B. Shia, and S. Ma, “Combining multidimensional genomic measurements for predicting cancer prognosis: Observations from TCGA,” Briefings in Bioinformatics, vol. 16, no. 2, pp. 291–303, 2015. [21] Q. Mo, F. Nikolos, F. Chen, Z. Tramel, Y.-C. Lee, K. Hayashi et al., “Prognostic power of a tumor differentiation gene signature for bladder urothelial carcinomas,” Journal of the National Cancer Institute, vol. 110, no. 5, pp. 448–459, 2018. [22] M. Cortet, A. Bertaut, F. Molinié, S. Bara, F. Beltjens, C. Coutant et al., “Trends in molecular subtypes of breast cancer: Description of incidence rates between 2007 and 2012 from three French registries,” BMC Cancer, vol. 18, no. 1, p. 161, 2018. [23] L. Zhao, V. H. Lee, M. K. Ng, H. Yan, and M. F. Bijlsma, “Molecular subtyping of cancer: Current status and moving toward clinical applications,” Briefings in Bioinformatics, vol. 20, no. 2, pp. 572–584, 2019. [24] M. Hofree, J. P. Shen, H. Carter, A. Gross, and T. Ideker, “Network-based stratification of tumor mutations,” Nature methods, vol. 10, no. 11, pp. 1108–1115, 2013. [25] Z. He, J. Zhang, X. Yuan, Z. Liu, B. Liu, S. Tuo et al., “Network based stratification of major cancers by integrating somatic mutation and gene expression data,” PloS One, vol. 12, no. 5, 2017. [26] B. Wang, A. M. Mezlini, F. Demir, M. Fiume, Z. Tu, M. Brudno et al., “Similarity network fusion for aggregating data types on a genomic scale,” Nature Methods, vol. 11, no. 3, pp. 333–337, 2014. [27] F. Zhang, C. Ren, K. K. Lau, Z. Zheng, G. Lu, Z. Yi et al., “A network medicine approach to build a comprehensive atlas for the prognosis of human cancer,” Briefings in Bioinfor- matics, vol. 17, no. 6, pp. 1044–1059, 2016. [28] M. Le Morvan, A. Zinovyev, and J.-P. Vert, “NetNorM: Capturing cancer-relevant information in somatic exome mutation data with gene networks for cancer stratification and prognosis,” PLoS Computational Biology, vol. 13, no. 6, p. e1005573, 2017. [29] C. R. Planey and O. Gevaert, “CoINcIDE: A framework for discovery of patient subtypes across multiple datasets,” Genome Medicine, vol. 8, no. 1, pp. 1–17, 2016. [30] G. Yu, X. Yu, and J. Wang, “Network-aided Bi-Clustering for discovering cancer subtypes,” Scientific Reports, vol. 7, no. 1, pp. 1–15, 2017. [31] F. Azuaje, “Computational models for predicting drug re- sponses in cancer research,” Briefings in Bioinformatics, vol. 18, no. 5, pp. 820–829, 2017. [32] N. Zhang, H. Wang, Y. Fang, J. Wang, X. Zheng, and X. S. Liu, “Predicting anticancer drug responses using a dual-layer integrated cell line-drug network model,” PLoS Computational Biology, vol. 11, no. 9, 2015. [33] D.-H. Le and V.-H. Pham, “Drug response prediction by globally capturing drug and cell line information in a hetero- geneous network,” Journal of Molecular Biology, vol. 430, no. 18, pp. 2993–3004, 2018. [34] J. C. Costello, L. M. Heiser, E. Georgii, M. Go¨nen, M. P. Menden, N. J. Wang et al., “A community effort to assess and improve drug sensitivity prediction algorithms,” Nature Biotechnology, vol. 32, no. 12, pp. 1202–1212, 2014. [35] M. Ammad-ud din, S. A. Khan, D. Malani, A. Muruma¨gi, O. Kallioniemi, T. Aittokallio et al., “Drug response predic- tion by inferring pathway-response associations with kernel- ized Bayesian matrix factorization,” Bioinformatics, vol. 32, no. 17, pp. i455–i463, 2016. [36] D. Le and D. Nguyen-Ngoc, “Multi-task regression learning for prediction of response against a panel of anti-cancer drugs in personalized medicine,” in Proceedings of the In- ternational Conference on Multimedia Analysis and Pattern Recognition, Ho Chi Minh City, Vietnam, Apr. 2018. 7 Research and Development on Information and Communication Technology Le Duc Hau obtained his PhD degree in Bioinformatics from University of Ul- san, Republic of Korea in 2012. He is now leading the Department of Compu- tational Biomedicine, Vingroup Big Data Institute, VietNam. He has been focus- ing on proposing computational methods for disease- and drug-related problems in personalized medicine, especially on identification of disease- associated biomarkers, prediction of drug targets and response. In parallel, he has been developing bioinformatics tools. So far, he hasmore than fifty papers published in well-recognized journals and conferences, nearly a half of those are in ISI-indexed journals. In addition, he has been a member of program committees and reviewer of several international conferences/journals. More- over, he is a principal investigator and a key member of some national/ministry-level projects. Specially, he is the principal in- vestigator of the biggest genome project in Vietnam (i.e., building databases of genomic variants for Vietnamese population). Finally, he has been collaborating with some well-recognized international research institutes. Quynh Diep Nguyen obtained her PhD degree in Information Technology from the Institute of Information Technology - The Vietnam Academy of Science and Tech- nology in 2015. She is a lecturer at the School of Computer Science and Engi- neering, Thuyloi University. She has been focusing on computational methods for re- constructing the metabolic networks. So far, she has more than fifteen papers in journals and conferences published . Moreover, she is a member of some national/ministry-level projects which re- search on computational methods for uncovering latent knowledge from high-throughput biological data. 8
File đính kèm:
computational_personalized_medicine_in_cancer_research_in_th.pdf