On the performance of 1-Bit ADC in Massive MIMO communication systems
Massive multiple-Input multiple-output (MIMO) with low-resolution analog-to-digital converters is a rational solution to deal with hardware costs and accomplish optimal energy efficiency. In particular, utilizing 1-bit ADCs is one of the best choices for massive MIMO systems. This paper investigates the performance of the 1-bit ADC in the wireless coded communication systems where the robust channel coding, protograph low-density parity-check code (LDPC), is employed. The investigation reveals that the performance of the conventional 1-bit ADC with the truncation limit of 3-sigma is severely destroyed by the quantization distortion even when the number of antennas increases to 100. In particular, the optimized 1-bit ADC can achieved the iterative decoding threshold gain of 2 dB over the conventional 3-sigma 1-bit ADC at the coding rate of 1/2 and the gain is more significant at higher coding rates. The optimized 1-bit ADC, though having substantial performance gain over the conventional one, is also affected by the quantization distortion at high coding rates and low MIMO configurations. Importantly, the investigation results suggest that the protograph LDPC codes should be re-designed to combat the negative effect of the quantization distortion of the 1-bit ADC
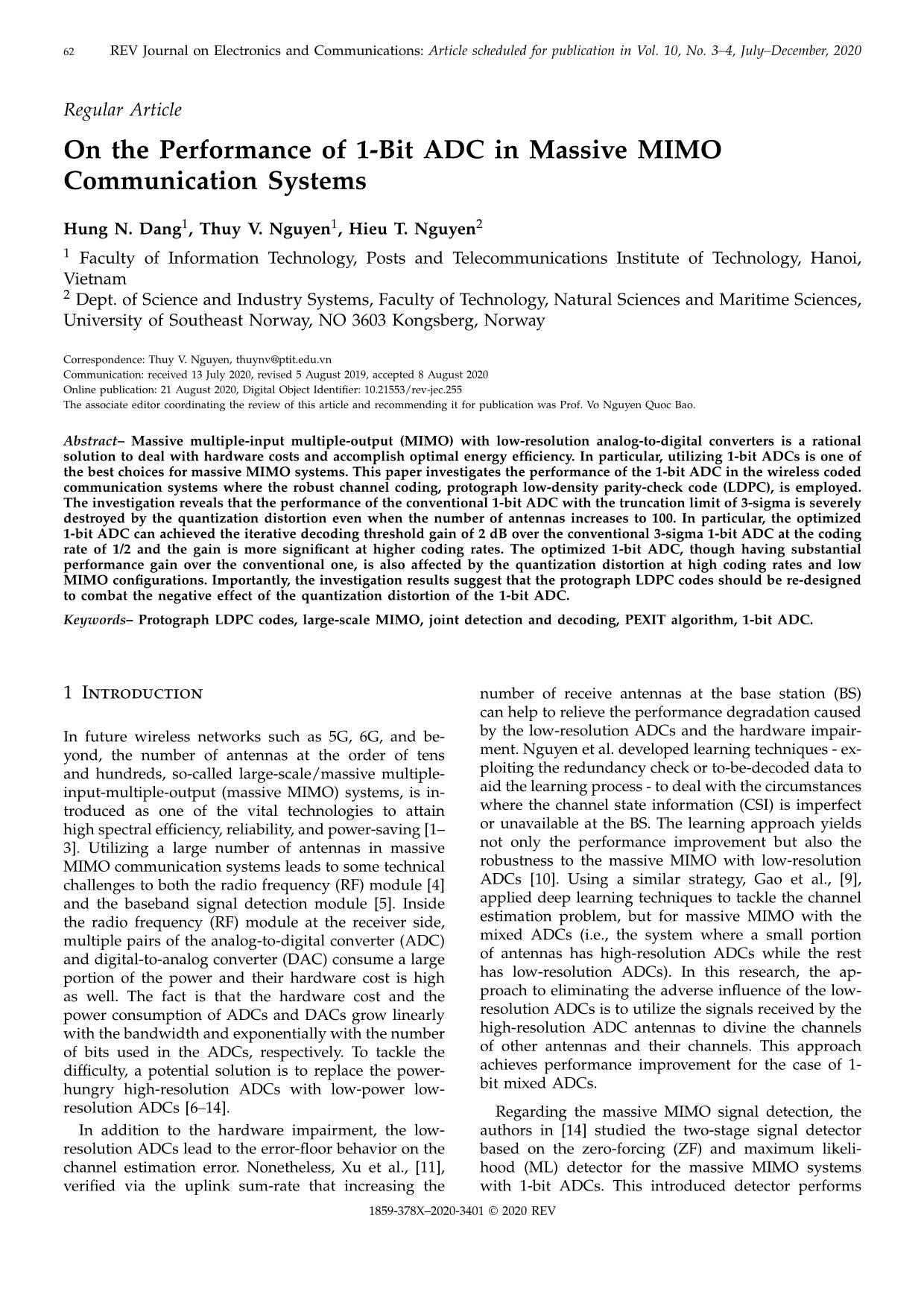
Trang 1
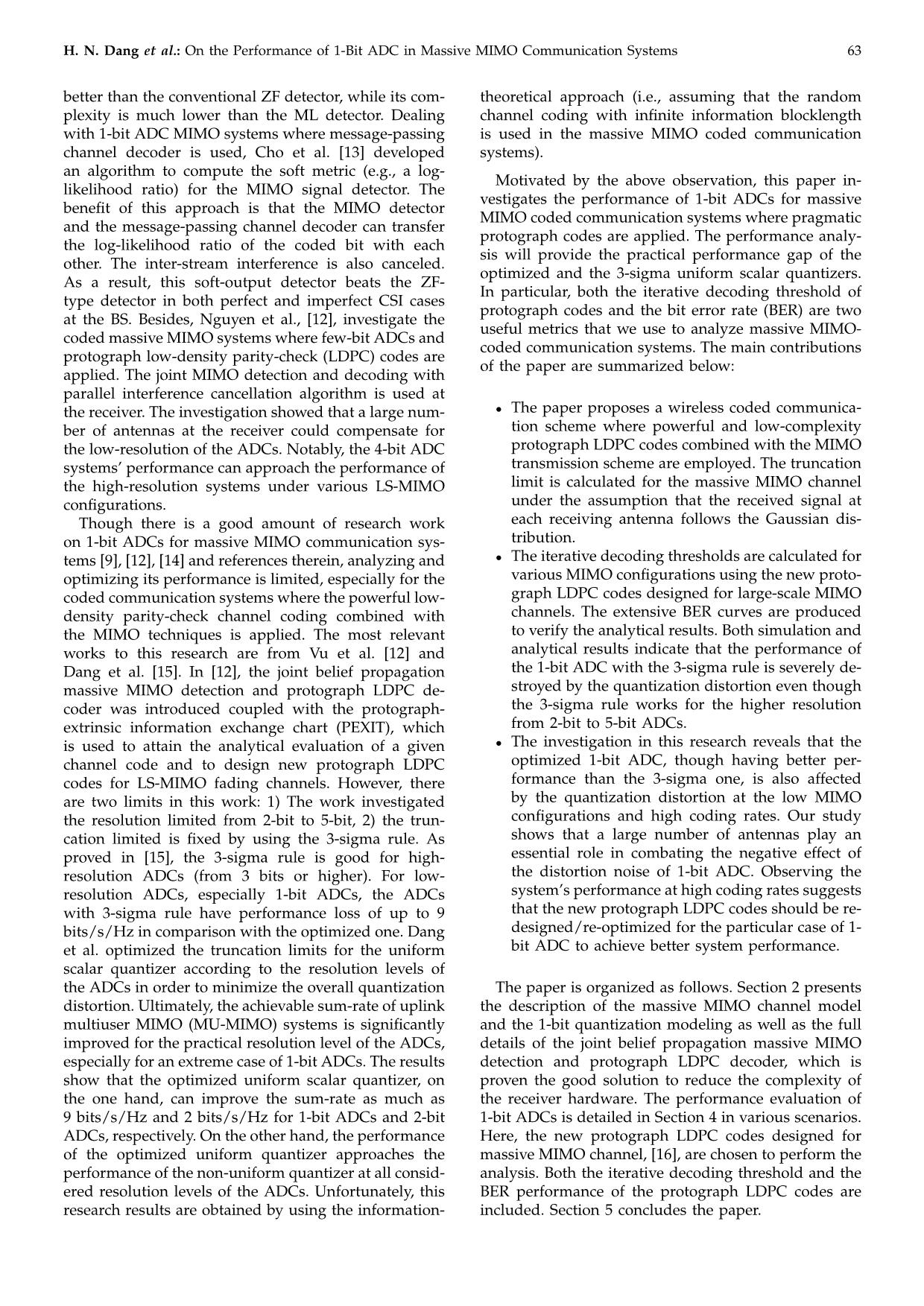
Trang 2
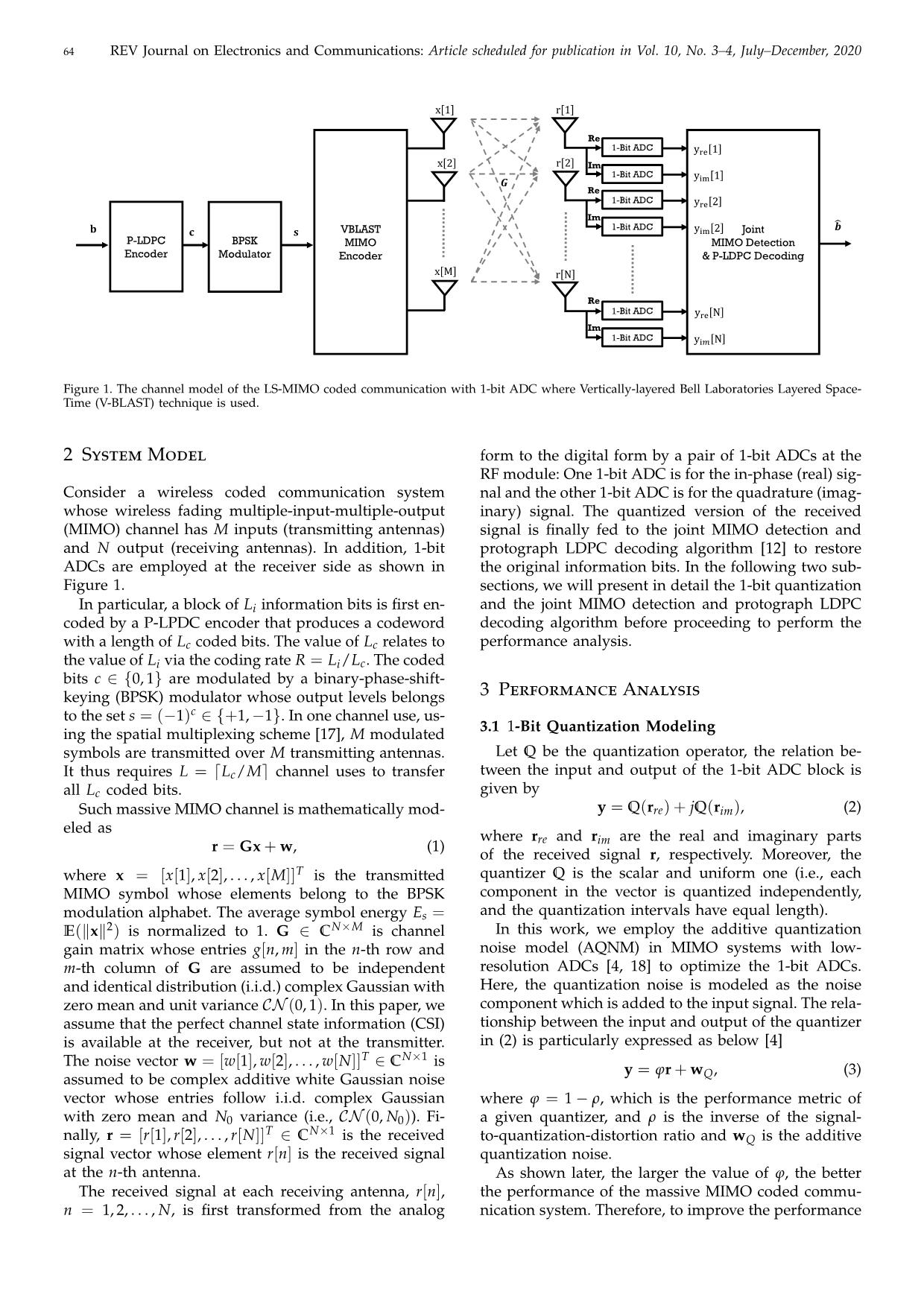
Trang 3
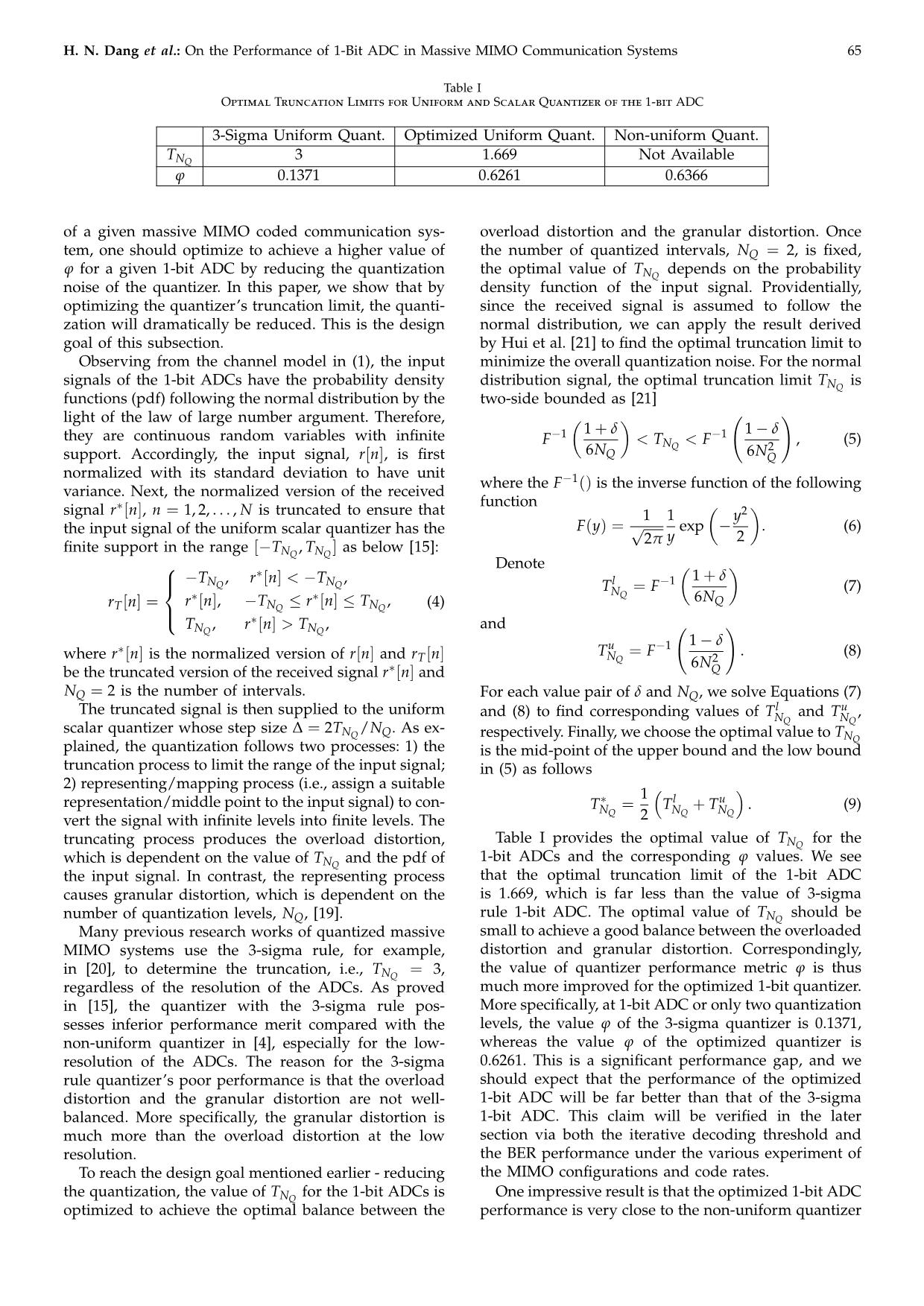
Trang 4
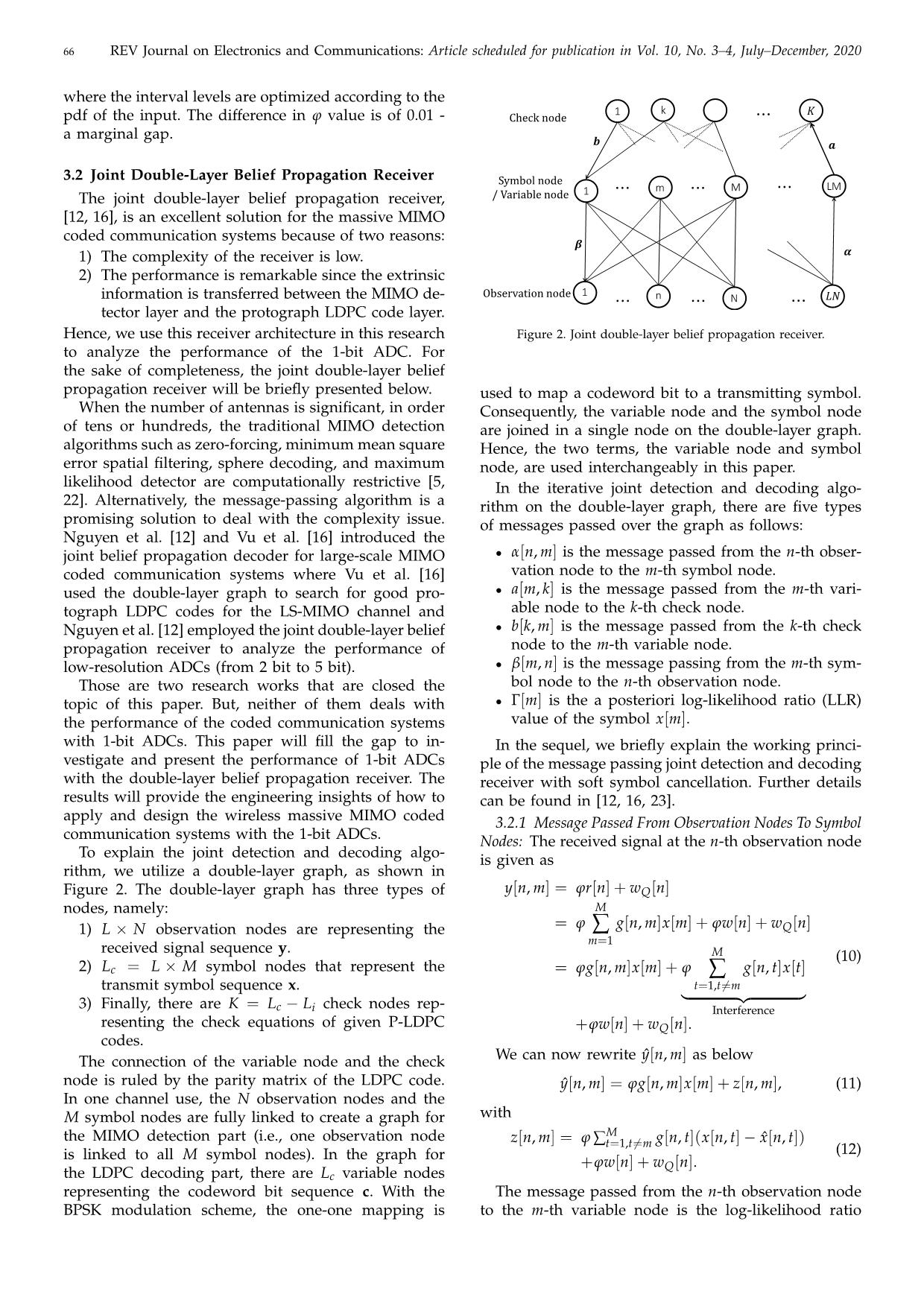
Trang 5
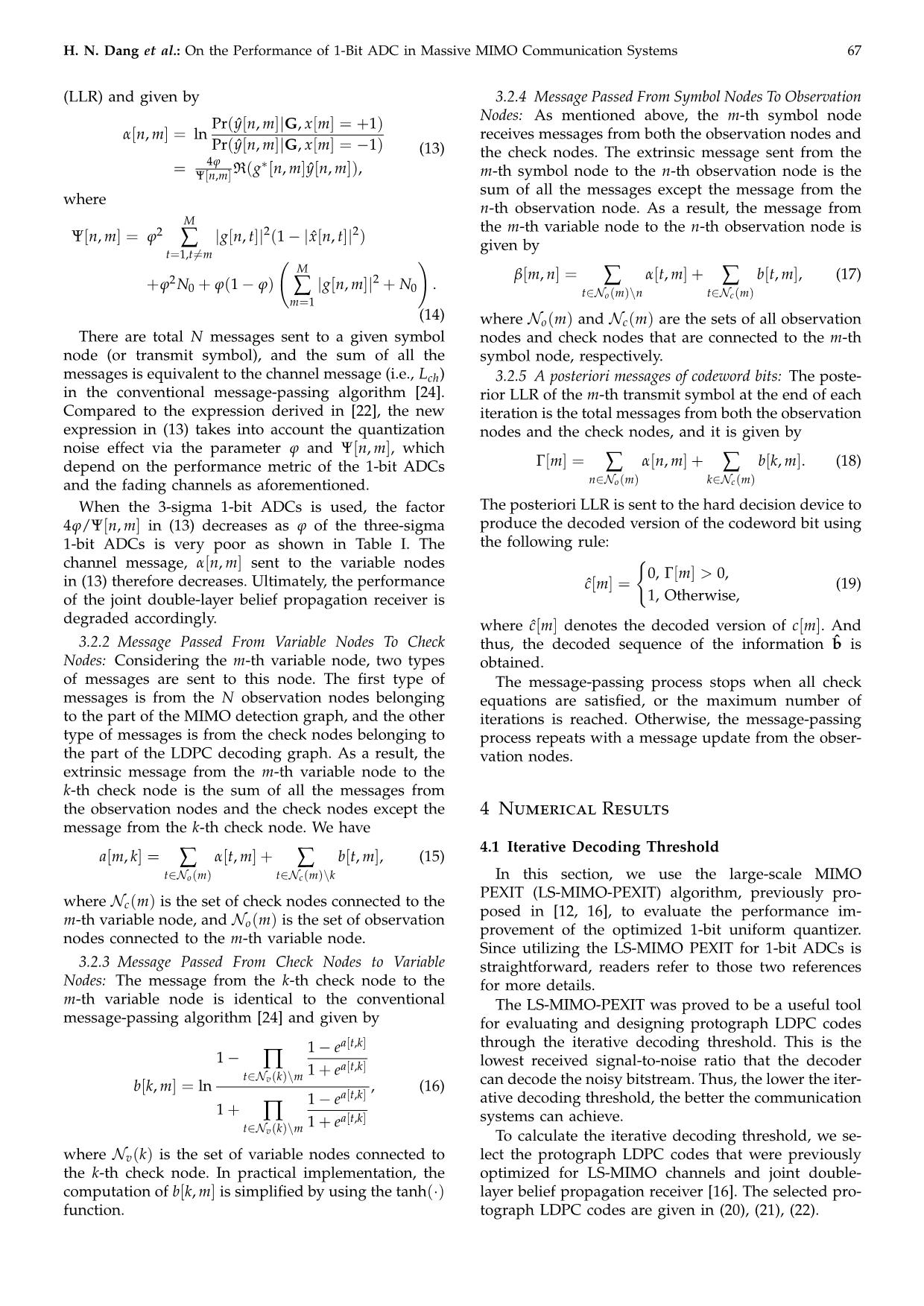
Trang 6
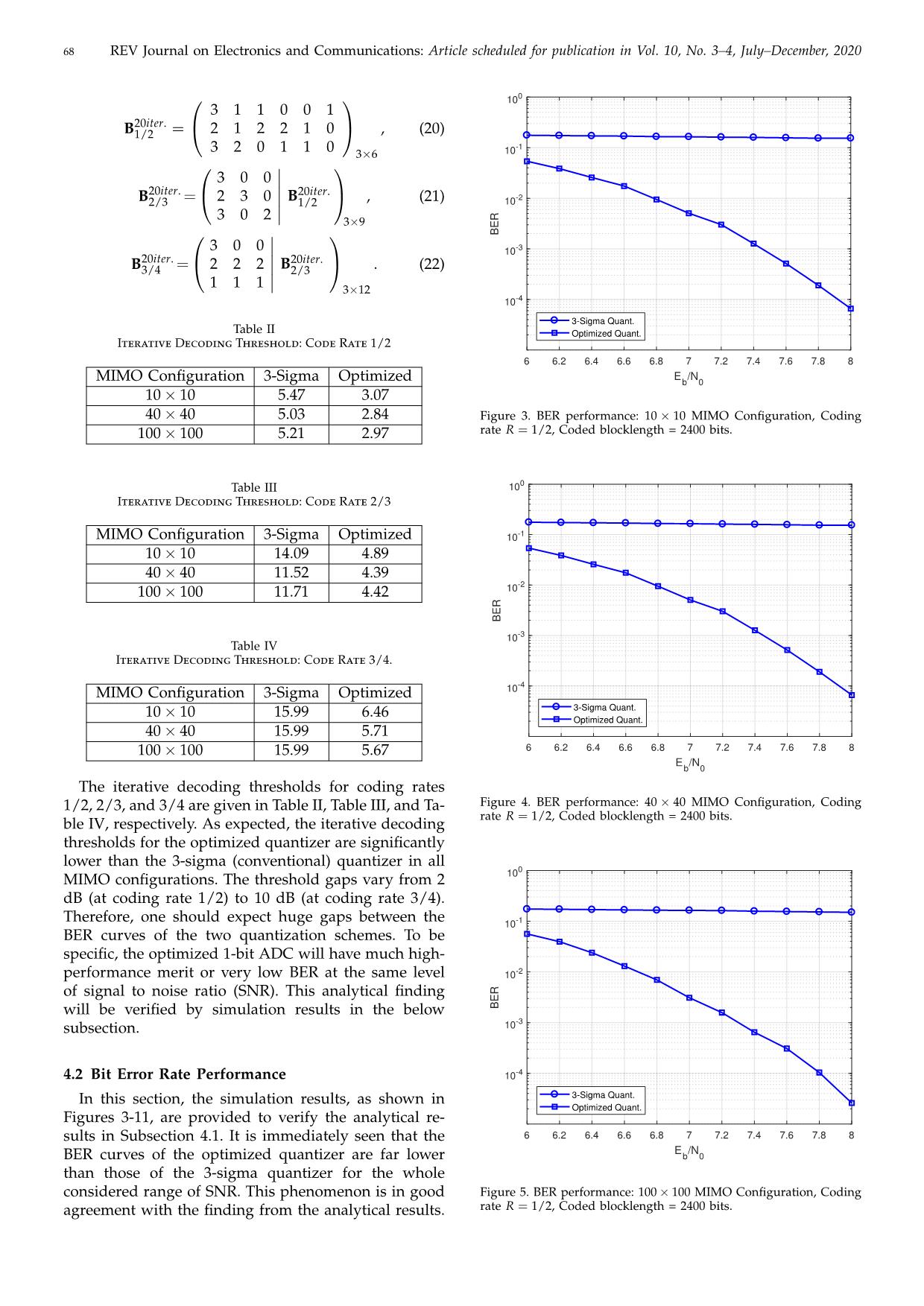
Trang 7
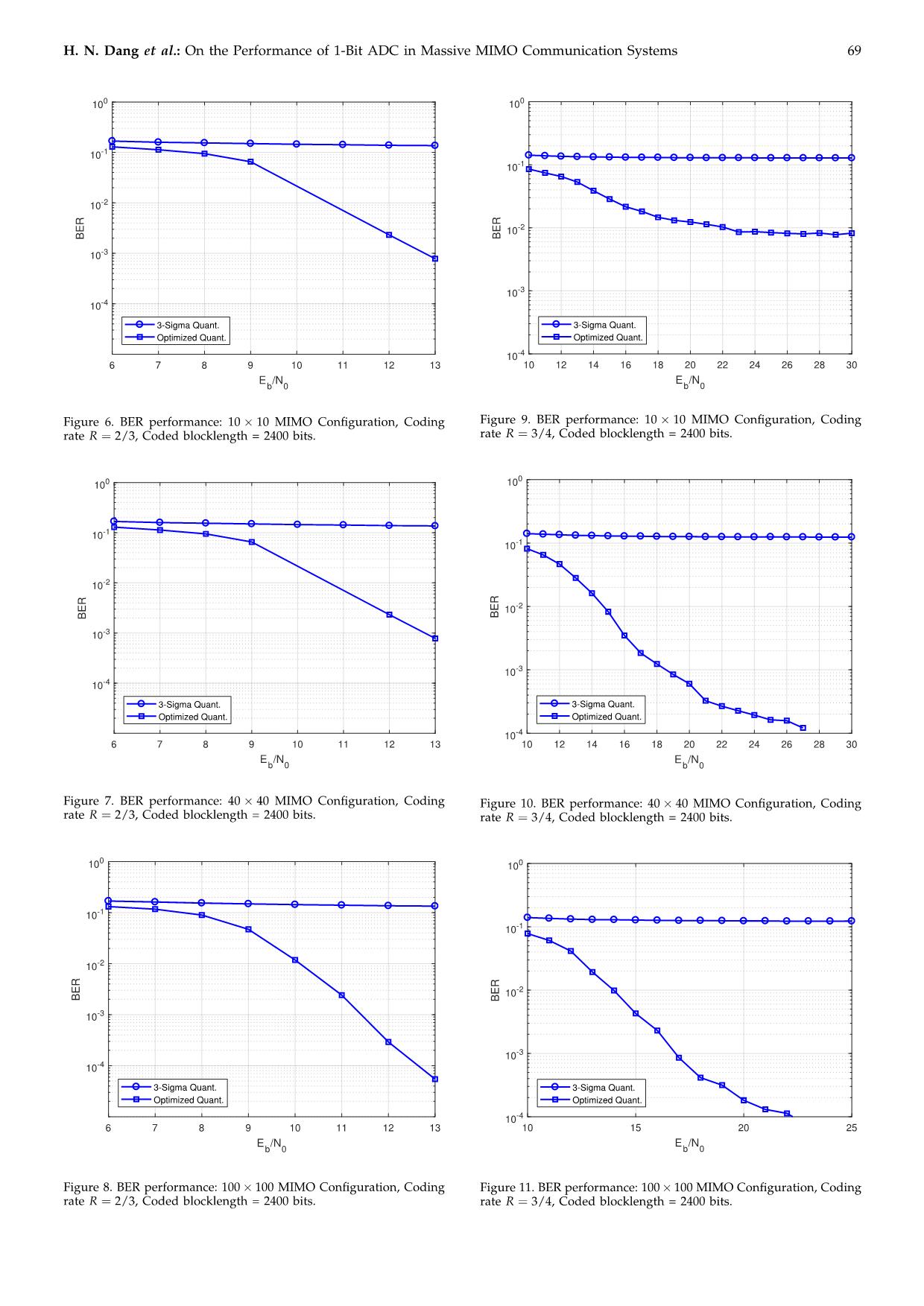
Trang 8
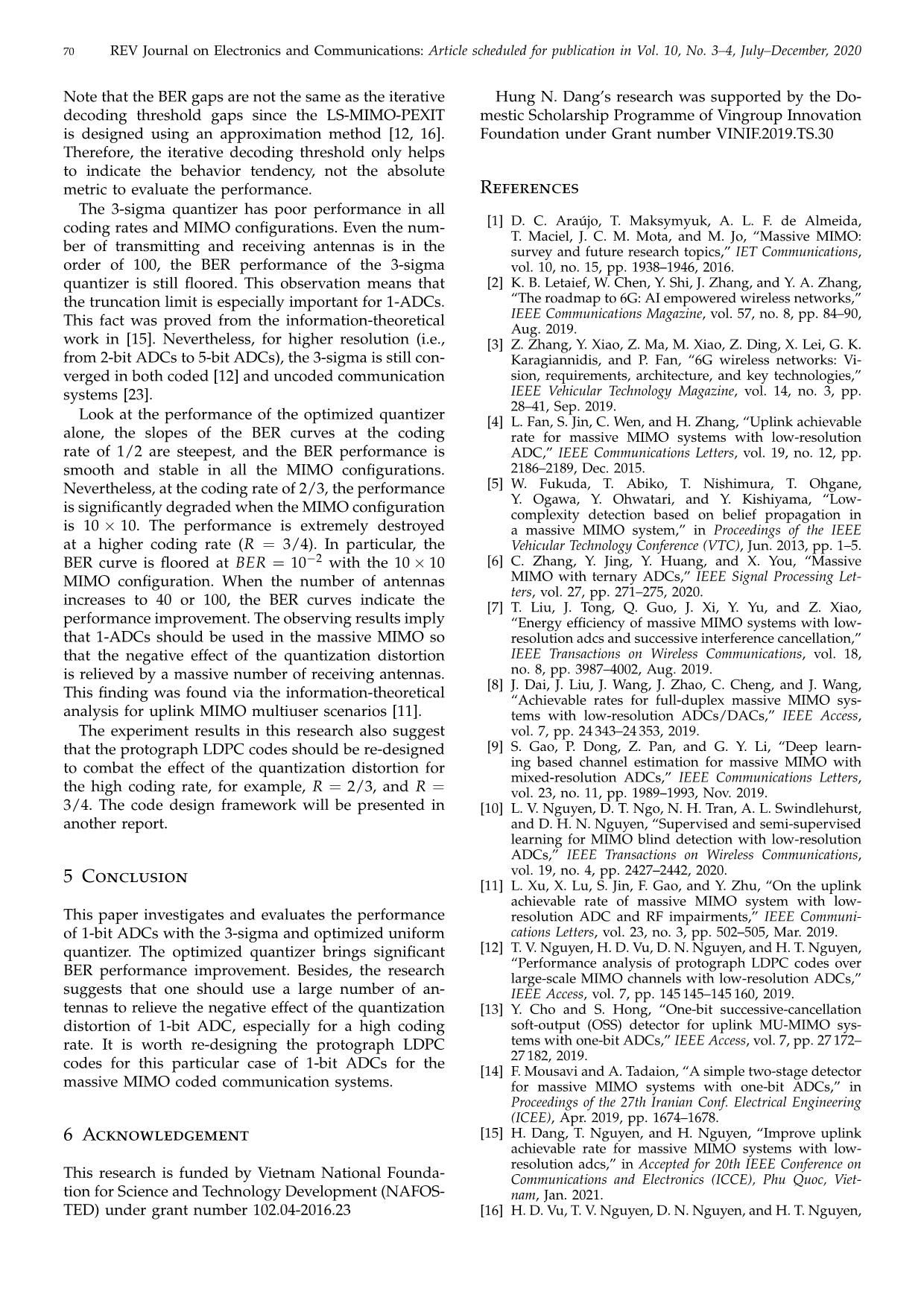
Trang 9
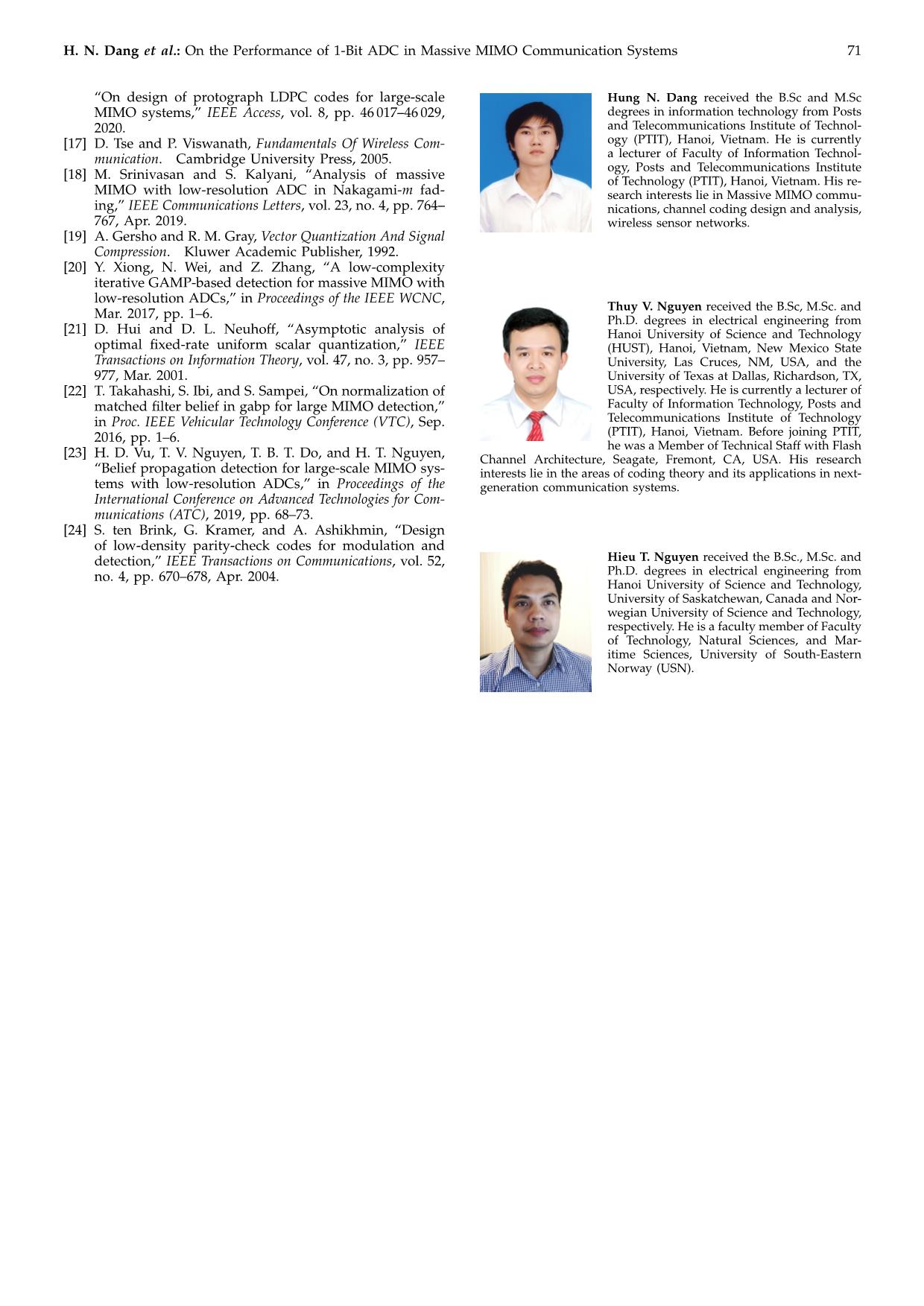
Trang 10
Tóm tắt nội dung tài liệu: On the performance of 1-Bit ADC in Massive MIMO communication systems
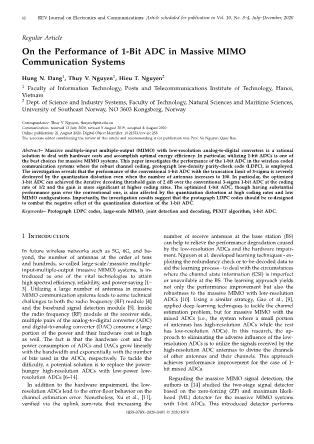
ected to the c posed in [12, 16], to evaluate the performance im- m-th variable node, and N (m) is the set of observation o provement of the optimized 1-bit uniform quantizer. nodes connected to the m-th variable node. Since utilizing the LS-MIMO PEXIT for 1-bit ADCs is 3.2.3 Message Passed From Check Nodes to Variable straightforward, readers refer to those two references Nodes: The message from the k-th check node to the for more details. m-th variable node is identical to the conventional The LS-MIMO-PEXIT was proved to be a useful tool message-passing algorithm [24] and given by for evaluating and designing protograph LDPC codes 1 − ea[t,k] through the iterative decoding threshold. This is the 1 − ∏ a[t,k] lowest received signal-to-noise ratio that the decoder t∈N (k)\m 1 + e b[k, m] = ln v , (16) can decode the noisy bitstream. Thus, the lower the iter- 1 − ea[t,k] ative decoding threshold, the better the communication 1 + ∏ a[t,k] systems can achieve. t∈N (k)\m 1 + e v To calculate the iterative decoding threshold, we se- where Nv(k) is the set of variable nodes connected to lect the protograph LDPC codes that were previously the k-th check node. In practical implementation, the optimized for LS-MIMO channels and joint double- computation of b[k, m] is simplified by using the tanh(·) layer belief propagation receiver [16]. The selected pro- function. tograph LDPC codes are given in (20), (21), (22). 68 REV Journal on Electronics and Communications: Article scheduled for publication in Vol. 10, No. 3–4, July–December, 2020 100 3 1 1 0 0 1 20iter. B1/2 = 2 1 2 2 1 0 , (20) -1 3 2 0 1 1 0 3×6 10 3 0 0 20iter. 20iter. B2/3 = 2 3 0 B1/2 , (21) 10-2 3 0 2 3×9 BER 3 0 0 10-3 20iter. 20iter. B3/4 = 2 2 2 B2/3 . (22) 1 1 1 3×12 10-4 3-Sigma Quant. Table II Optimized Quant. Iterative Decoding Threshold:Code Rate 1/2 6 6.2 6.4 6.6 6.8 7 7.2 7.4 7.6 7.8 8 E /N MIMO Configuration 3-Sigma Optimized b 0 10 × 10 5.47 3.07 40 × 40 5.03 2.84 Figure 3. BER performance: 10 × 10 MIMO Configuration, Coding 100 × 100 5.21 2.97 rate R = 1/2, Coded blocklength = 2400 bits. Table III 100 Iterative Decoding Threshold:Code Rate 2/3 MIMO Configuration 3-Sigma Optimized 10-1 10 × 10 14.09 4.89 40 × 40 11.52 4.39 -2 100 × 100 11.71 4.42 10 BER 10-3 Table IV Iterative Decoding Threshold:Code Rate 3/4. -4 MIMO Configuration 3-Sigma Optimized 10 × 3-Sigma Quant. 10 10 15.99 6.46 Optimized Quant. 40 × 40 15.99 5.71 100 × 100 15.99 5.67 6 6.2 6.4 6.6 6.8 7 7.2 7.4 7.6 7.8 8 E /N b 0 The iterative decoding thresholds for coding rates 1/2, 2/3, and 3/4 are given in Table II, Table III, and Ta- Figure 4. BER performance: 40 × 40 MIMO Configuration, Coding rate R = 1/2, Coded blocklength = 2400 bits. ble IV, respectively. As expected, the iterative decoding thresholds for the optimized quantizer are significantly lower than the 3-sigma (conventional) quantizer in all 0 MIMO configurations. The threshold gaps vary from 2 10 dB (at coding rate 1/2) to 10 dB (at coding rate 3/4). Therefore, one should expect huge gaps between the 10-1 BER curves of the two quantization schemes. To be specific, the optimized 1-bit ADC will have much high- performance merit or very low BER at the same level 10-2 of signal to noise ratio (SNR). This analytical finding will be verified by simulation results in the below BER -3 subsection. 10 4.2 Bit Error Rate Performance 10-4 In this section, the simulation results, as shown in 3-Sigma Quant. Optimized Quant. Figures 3-11, are provided to verify the analytical re- sults in Subsection 4.1. It is immediately seen that the 6 6.2 6.4 6.6 6.8 7 7.2 7.4 7.6 7.8 8 E /N BER curves of the optimized quantizer are far lower b 0 than those of the 3-sigma quantizer for the whole considered range of SNR. This phenomenon is in good Figure 5. BER performance: 100 × 100 MIMO Configuration, Coding agreement with the finding from the analytical results. rate R = 1/2, Coded blocklength = 2400 bits. H. N. Dang et al.: On the Performance of 1-Bit ADC in Massive MIMO Communication Systems 69 100 100 10-1 10-1 10-2 10-2 BER BER 10-3 10-3 10-4 3-Sigma Quant. 3-Sigma Quant. Optimized Quant. Optimized Quant. 10-4 6 7 8 9 10 11 12 13 10 12 14 16 18 20 22 24 26 28 30 E /N E /N b 0 b 0 Figure 6. BER performance: 10 × 10 MIMO Configuration, Coding Figure 9. BER performance: 10 × 10 MIMO Configuration, Coding rate R = 2/3, Coded blocklength = 2400 bits. rate R = 3/4, Coded blocklength = 2400 bits. 0 100 10 10-1 10-1 10-2 10-2 BER BER 10-3 10-3 10-4 3-Sigma Quant. 3-Sigma Quant. Optimized Quant. Optimized Quant. 10-4 6 7 8 9 10 11 12 13 10 12 14 16 18 20 22 24 26 28 30 E /N E /N b 0 b 0 Figure 7. BER performance: 40 × 40 MIMO Configuration, Coding Figure 10. BER performance: 40 × 40 MIMO Configuration, Coding rate R = 2/3, Coded blocklength = 2400 bits. rate R = 3/4, Coded blocklength = 2400 bits. 0 10 100 10-1 10-1 10-2 10-2 BER BER 10-3 10-3 10-4 3-Sigma Quant. 3-Sigma Quant. Optimized Quant. Optimized Quant. 10-4 6 7 8 9 10 11 12 13 10 15 20 25 E /N E /N b 0 b 0 Figure 8. BER performance: 100 × 100 MIMO Configuration, Coding Figure 11. BER performance: 100 × 100 MIMO Configuration, Coding rate R = 2/3, Coded blocklength = 2400 bits. rate R = 3/4, Coded blocklength = 2400 bits. 70 REV Journal on Electronics and Communications: Article scheduled for publication in Vol. 10, No. 3–4, July–December, 2020 Note that the BER gaps are not the same as the iterative Hung N. Dang’s research was supported by the Do- decoding threshold gaps since the LS-MIMO-PEXIT mestic Scholarship Programme of Vingroup Innovation is designed using an approximation method [12, 16]. Foundation under Grant number VINIF.2019.TS.30 Therefore, the iterative decoding threshold only helps to indicate the behavior tendency, not the absolute metric to evaluate the performance. References The 3-sigma quantizer has poor performance in all coding rates and MIMO configurations. Even the num- [1] D. C. Araújo, T. Maksymyuk, A. L. F. de Almeida, T. Maciel, J. C. M. Mota, and M. Jo, “Massive MIMO: ber of transmitting and receiving antennas is in the survey and future research topics,” IET Communications, order of 100, the BER performance of the 3-sigma vol. 10, no. 15, pp. 1938–1946, 2016. quantizer is still floored. This observation means that [2] K. B. Letaief, W. Chen, Y. Shi, J. Zhang, and Y. A. Zhang, the truncation limit is especially important for 1-ADCs. “The roadmap to 6G: AI empowered wireless networks,” This fact was proved from the information-theoretical IEEE Communications Magazine, vol. 57, no. 8, pp. 84–90, Aug. 2019. work in [15]. Nevertheless, for higher resolution (i.e., [3] Z. Zhang, Y. Xiao, Z. Ma, M. Xiao, Z. Ding, X. Lei, G. K. from 2-bit ADCs to 5-bit ADCs), the 3-sigma is still con- Karagiannidis, and P. Fan, “6G wireless networks: Vi- verged in both coded [12] and uncoded communication sion, requirements, architecture, and key technologies,” systems [23]. IEEE Vehicular Technology Magazine, vol. 14, no. 3, pp. 28–41, Sep. 2019. Look at the performance of the optimized quantizer [4] L. Fan, S. Jin, C. Wen, and H. Zhang, “Uplink achievable alone, the slopes of the BER curves at the coding rate for massive MIMO systems with low-resolution rate of 1/2 are steepest, and the BER performance is ADC,” IEEE Communications Letters, vol. 19, no. 12, pp. smooth and stable in all the MIMO configurations. 2186–2189, Dec. 2015. Nevertheless, at the coding rate of 2/3, the performance [5] W. Fukuda, T. Abiko, T. Nishimura, T. Ohgane, Y. Ogawa, Y. Ohwatari, and Y. Kishiyama, “Low- is significantly degraded when the MIMO configuration complexity detection based on belief propagation in is 10 × 10. The performance is extremely destroyed a massive MIMO system,” in Proceedings of the IEEE at a higher coding rate (R = 3/4). In particular, the Vehicular Technology Conference (VTC), Jun. 2013, pp. 1–5. BER curve is floored at BER = 10−2 with the 10 × 10 [6] C. Zhang, Y. Jing, Y. Huang, and X. You, “Massive MIMO configuration. When the number of antennas MIMO with ternary ADCs,” IEEE Signal Processing Let- ters, vol. 27, pp. 271–275, 2020. increases to 40 or 100, the BER curves indicate the [7] T. Liu, J. Tong, Q. Guo, J. Xi, Y. Yu, and Z. Xiao, performance improvement. The observing results imply “Energy efficiency of massive MIMO systems with low- that 1-ADCs should be used in the massive MIMO so resolution adcs and successive interference cancellation,” that the negative effect of the quantization distortion IEEE Transactions on Wireless Communications, vol. 18, is relieved by a massive number of receiving antennas. no. 8, pp. 3987–4002, Aug. 2019. [8] J. Dai, J. Liu, J. Wang, J. Zhao, C. Cheng, and J. Wang, This finding was found via the information-theoretical “Achievable rates for full-duplex massive MIMO sys- analysis for uplink MIMO multiuser scenarios [11]. tems with low-resolution ADCs/DACs,” IEEE Access, The experiment results in this research also suggest vol. 7, pp. 24 343–24 353, 2019. that the protograph LDPC codes should be re-designed [9] S. Gao, P. Dong, Z. Pan, and G. Y. Li, “Deep learn- to combat the effect of the quantization distortion for ing based channel estimation for massive MIMO with mixed-resolution ADCs,” IEEE Communications Letters, the high coding rate, for example, R = 2/3, and R = vol. 23, no. 11, pp. 1989–1993, Nov. 2019. 3/4. The code design framework will be presented in [10] L. V. Nguyen, D. T. Ngo, N. H. Tran, A. L. Swindlehurst, another report. and D. H. N. Nguyen, “Supervised and semi-supervised learning for MIMO blind detection with low-resolution ADCs,” IEEE Transactions on Wireless Communications, vol. 19, no. 4, pp. 2427–2442, 2020. 5 Conclusion [11] L. Xu, X. Lu, S. Jin, F. Gao, and Y. Zhu, “On the uplink achievable rate of massive MIMO system with low- This paper investigates and evaluates the performance resolution ADC and RF impairments,” IEEE Communi- of 1-bit ADCs with the 3-sigma and optimized uniform cations Letters, vol. 23, no. 3, pp. 502–505, Mar. 2019. quantizer. The optimized quantizer brings significant [12] T. V. Nguyen, H. D. Vu, D. N. Nguyen, and H. T. Nguyen, BER performance improvement. Besides, the research “Performance analysis of protograph LDPC codes over large-scale MIMO channels with low-resolution ADCs,” suggests that one should use a large number of an- IEEE Access, vol. 7, pp. 145 145–145 160, 2019. tennas to relieve the negative effect of the quantization [13] Y. Cho and S. Hong, “One-bit successive-cancellation distortion of 1-bit ADC, especially for a high coding soft-output (OSS) detector for uplink MU-MIMO sys- rate. It is worth re-designing the protograph LDPC tems with one-bit ADCs,” IEEE Access, vol. 7, pp. 27 172– codes for this particular case of 1-bit ADCs for the 27 182, 2019. [14] F. Mousavi and A. Tadaion, “A simple two-stage detector massive MIMO coded communication systems. for massive MIMO systems with one-bit ADCs,” in Proceedings of the 27th Iranian Conf. Electrical Engineering (ICEE), Apr. 2019, pp. 1674–1678. 6 Acknowledgement [15] H. Dang, T. Nguyen, and H. Nguyen, “Improve uplink achievable rate for massive MIMO systems with low- resolution adcs,” in Accepted for 20th IEEE Conference on This research is funded by Vietnam National Founda- Communications and Electronics (ICCE), Phu Quoc, Viet- tion for Science and Technology Development (NAFOS- nam, Jan. 2021. TED) under grant number 102.04-2016.23 [16] H. D. Vu, T. V. Nguyen, D. N. Nguyen, and H. T. Nguyen, H. N. Dang et al.: On the Performance of 1-Bit ADC in Massive MIMO Communication Systems 71 “On design of protograph LDPC codes for large-scale Hung N. Dang received the B.Sc and M.Sc MIMO systems,” IEEE Access, vol. 8, pp. 46 017–46 029, degrees in information technology from Posts 2020. and Telecommunications Institute of Technol- [17] D. Tse and P. Viswanath, Fundamentals Of Wireless Com- ogy (PTIT), Hanoi, Vietnam. He is currently munication. Cambridge University Press, 2005. a lecturer of Faculty of Information Technol- ogy, Posts and Telecommunications Institute [18] M. Srinivasan and S. Kalyani, “Analysis of massive of Technology (PTIT), Hanoi, Vietnam. His re- MIMO with low-resolution ADC in Nakagami-m fad- search interests lie in Massive MIMO commu- ing,” IEEE Communications Letters, vol. 23, no. 4, pp. 764– nications, channel coding design and analysis, 767, Apr. 2019. wireless sensor networks. [19] A. Gersho and R. M. Gray, Vector Quantization And Signal Compression. Kluwer Academic Publisher, 1992. [20] Y. Xiong, N. Wei, and Z. Zhang, “A low-complexity iterative GAMP-based detection for massive MIMO with low-resolution ADCs,” in Proceedings of the IEEE WCNC, Thuy V. Nguyen received the B.Sc, M.Sc. and Mar. 2017, pp. 1–6. Ph.D. degrees in electrical engineering from [21] D. Hui and D. L. Neuhoff, “Asymptotic analysis of Hanoi University of Science and Technology optimal fixed-rate uniform scalar quantization,” IEEE (HUST), Hanoi, Vietnam, New Mexico State Transactions on Information Theory, vol. 47, no. 3, pp. 957– University, Las Cruces, NM, USA, and the 977, Mar. 2001. University of Texas at Dallas, Richardson, TX, [22] T. Takahashi, S. Ibi, and S. Sampei, “On normalization of USA, respectively. He is currently a lecturer of matched filter belief in gabp for large MIMO detection,” Faculty of Information Technology, Posts and in Proc. IEEE Vehicular Technology Conference (VTC), Sep. Telecommunications Institute of Technology 2016, pp. 1–6. (PTIT), Hanoi, Vietnam. Before joining PTIT, he was a Member of Technical Staff with Flash [23] H. D. Vu, T. V. Nguyen, T. B. T. Do, and H. T. Nguyen, Channel Architecture, Seagate, Fremont, CA, USA. His research “Belief propagation detection for large-scale MIMO sys- interests lie in the areas of coding theory and its applications in next- tems with low-resolution ADCs,” in Proceedings of the generation communication systems. International Conference on Advanced Technologies for Com- munications (ATC), 2019, pp. 68–73. [24] S. ten Brink, G. Kramer, and A. Ashikhmin, “Design of low-density parity-check codes for modulation and detection,” IEEE Transactions on Communications, vol. 52, Hieu T. Nguyen received the B.Sc., M.Sc. and no. 4, pp. 670–678, Apr. 2004. Ph.D. degrees in electrical engineering from Hanoi University of Science and Technology, University of Saskatchewan, Canada and Nor- wegian University of Science and Technology, respectively. He is a faculty member of Faculty of Technology, Natural Sciences, and Mar- itime Sciences, University of South-Eastern Norway (USN).
File đính kèm:
on_the_performance_of_1_bit_adc_in_massive_mimo_communicatio.pdf