New results on robust state bounding estimation for discrete time markovian jumb stochastic control systems
The paper deals with the robust state bounding estimation problem of
stochastic control systems with discrete time Markovian jump. By
using Lyapunov functional method and probability theory, we
propose new sufficient conditions to guarantee robust state
boundedness for the stochastic control systems. The conditions are
derived in terms of linear matrix inequalities, which is simple and
convenient for testing and application. Unfortunately, difficulties arise
when one attempts to derive the sufficient conditions and to extract
the controller parameters for these systems. In fact, we have to cope
with stochastic process and disturbance. Indeed, the Lyapunov
functional method is a powerful tool to stability analysis of
differential systems. However, this method is not effectively applied
for stochastic systems because we do not know how to construct
suitable Lyapunov functions and use them in these systems. To
overcome the difficulties, we first introduced basic concepts of
probability theory. Next, a new sufficient conditions of robust state
boundedness for unforced stochastic systems was established. Finally,
the result was applied to design controllers to guarantee robust state
boundedness for stochastic control systems.
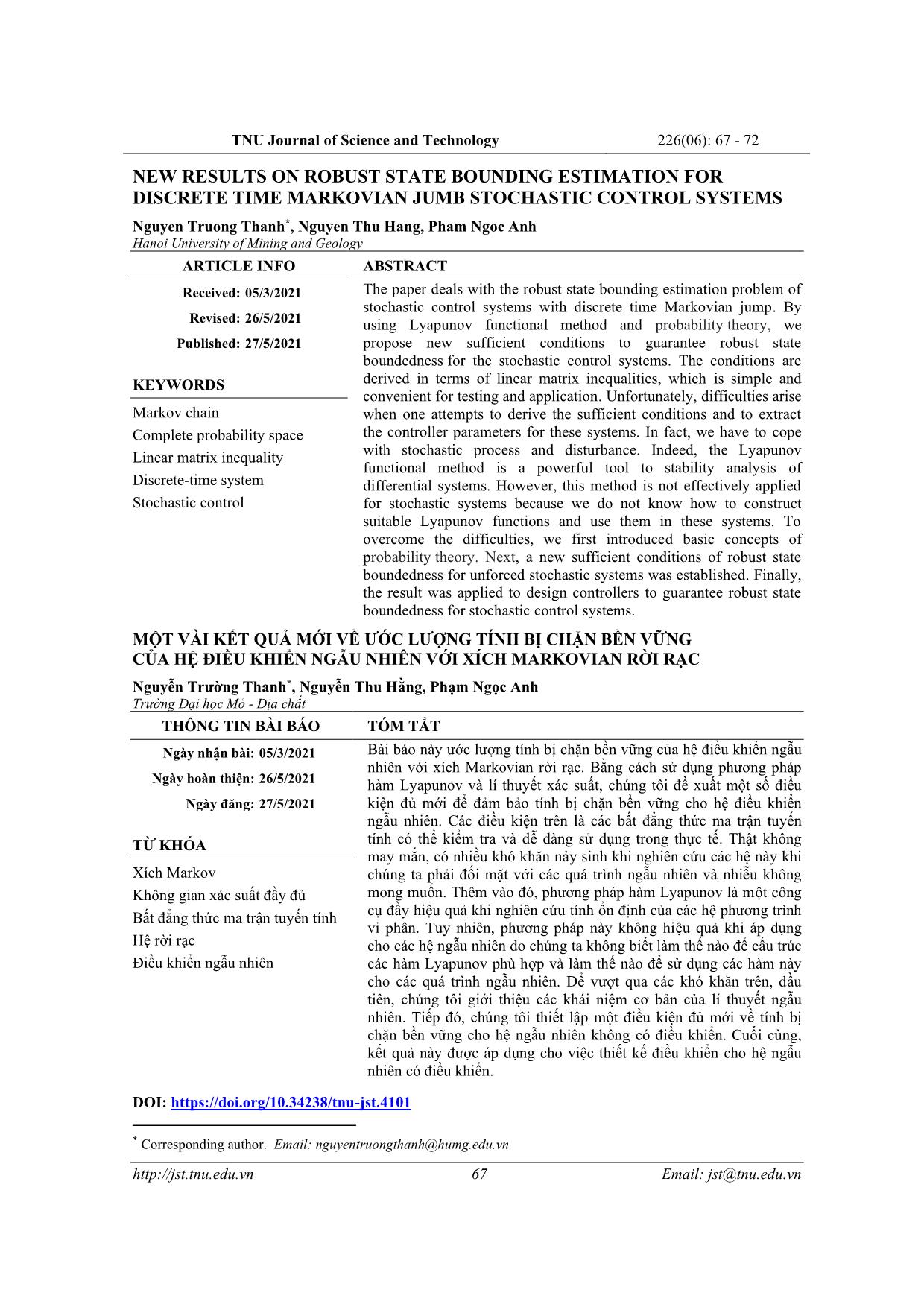
Trang 1
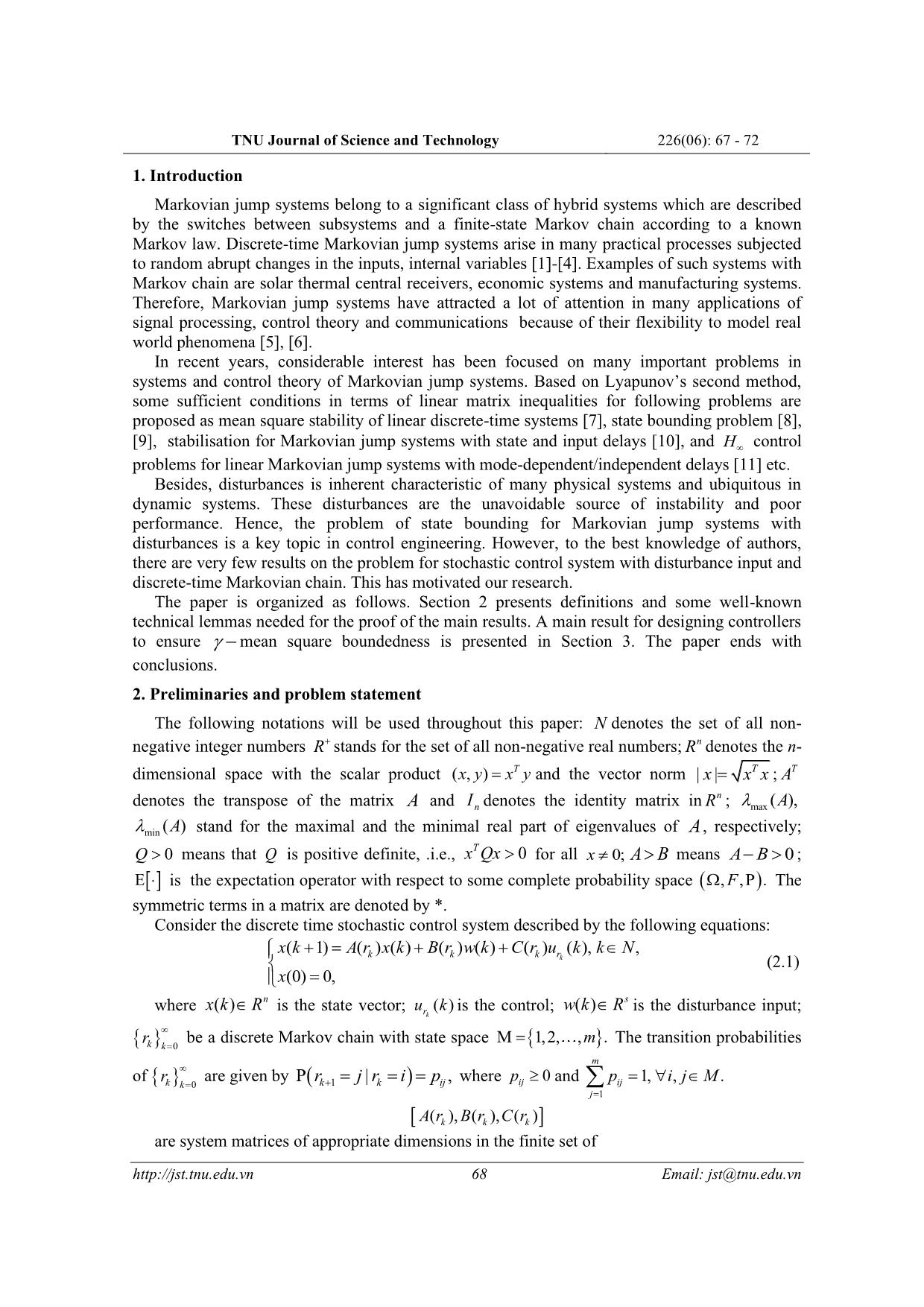
Trang 2
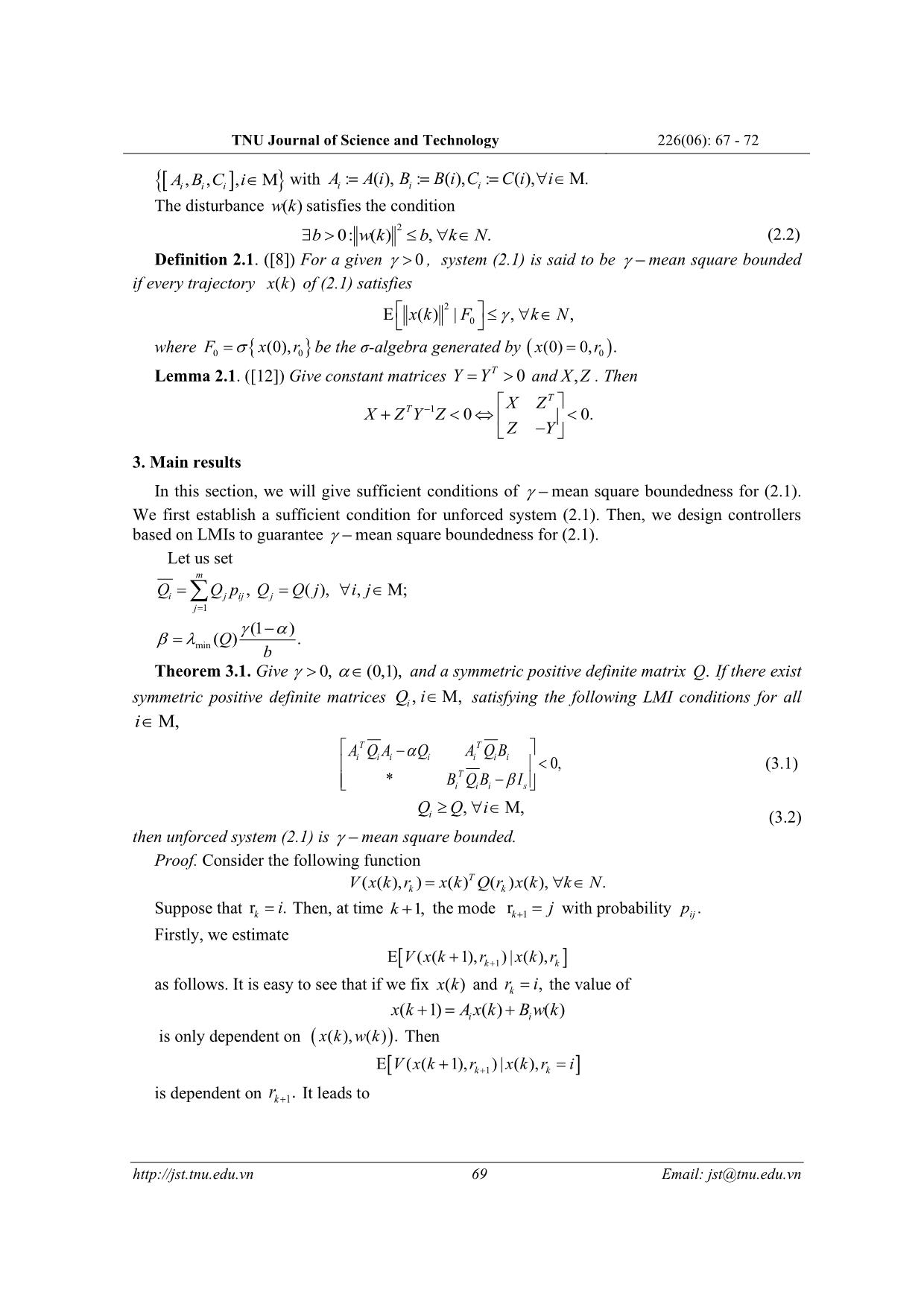
Trang 3
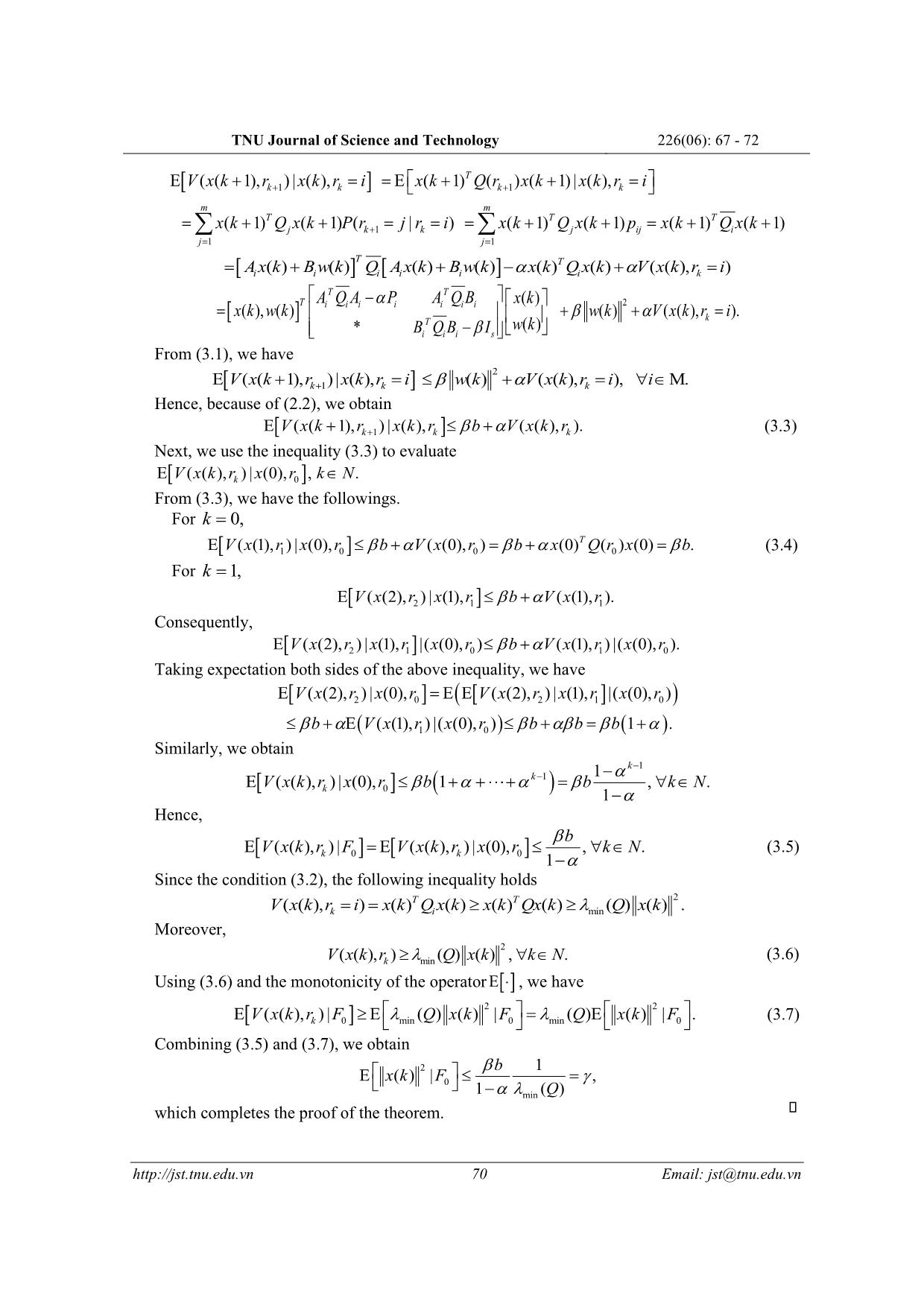
Trang 4
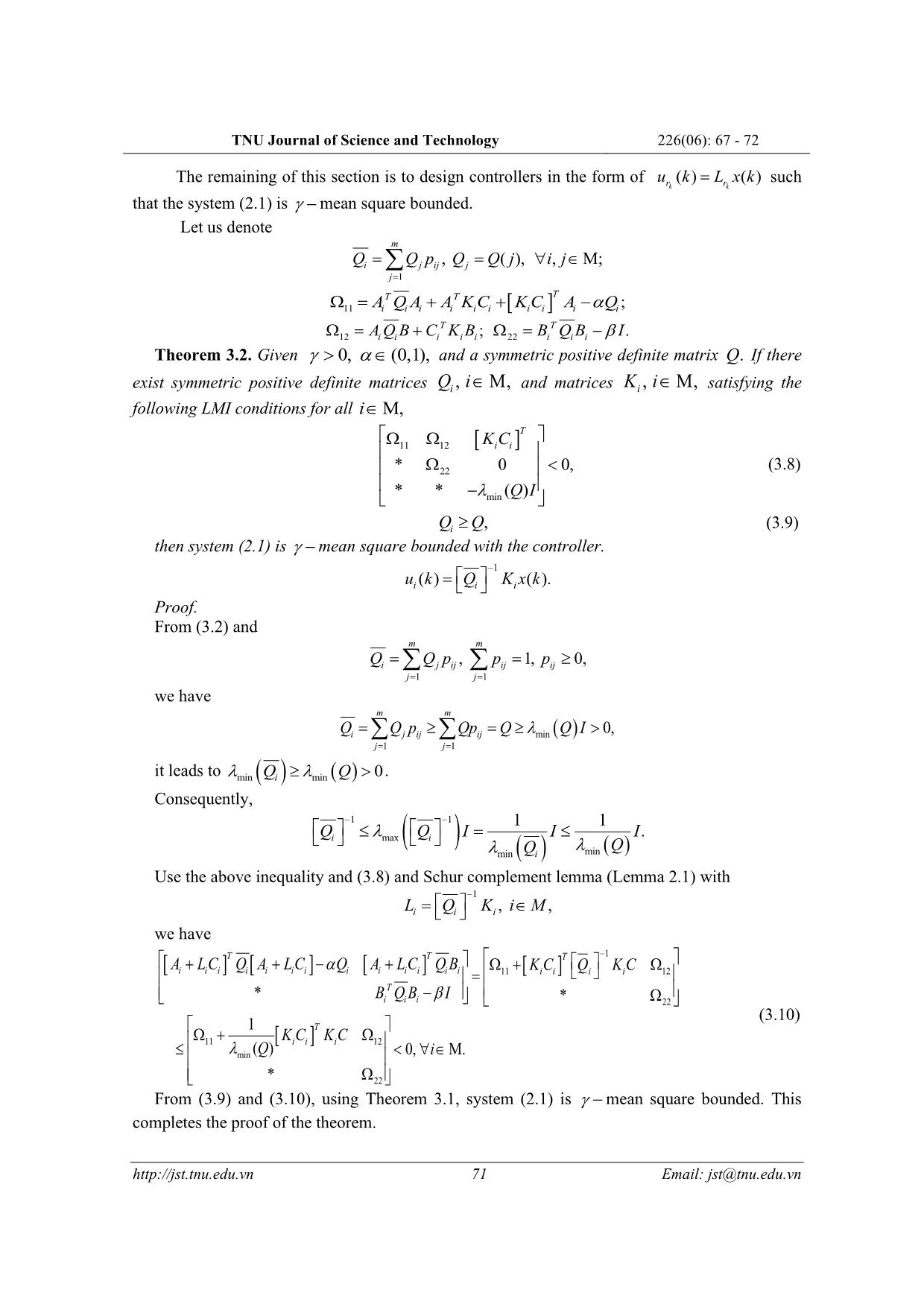
Trang 5
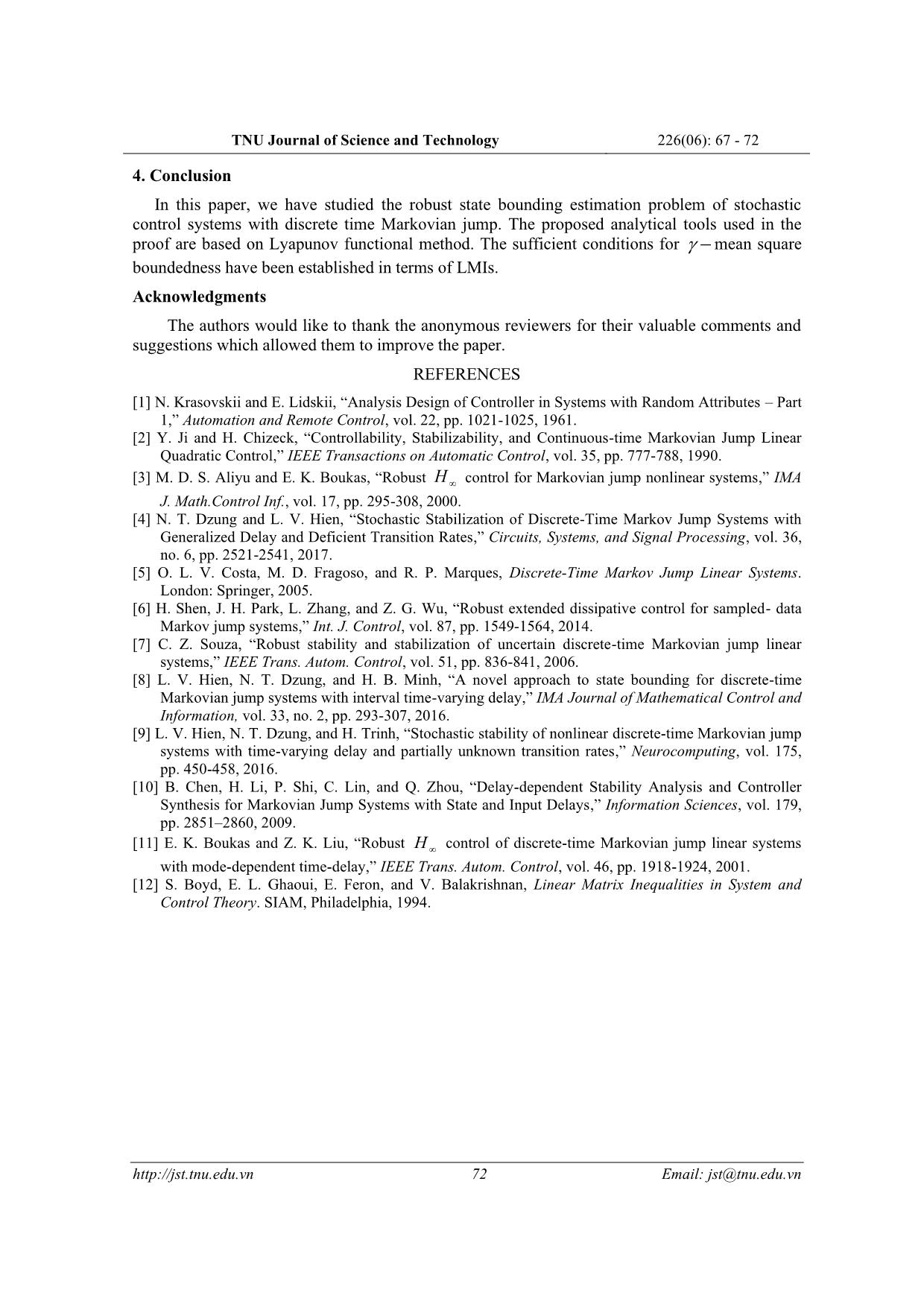
Trang 6
Tóm tắt nội dung tài liệu: New results on robust state bounding estimation for discrete time markovian jumb stochastic control systems
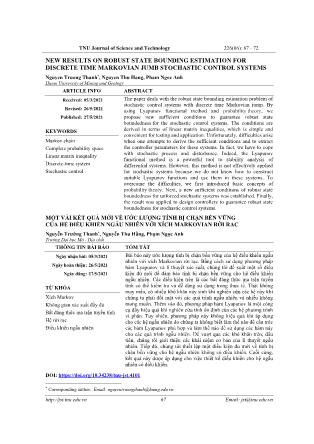
bounding estimation problem of stochastic control systems with discrete time Markovian jump. By Revised: 26/5/2021 using Lyapunov functional method and probability theory, we Published: 27/5/2021 propose new sufficient conditions to guarantee robust state boundedness for the stochastic control systems. The conditions are KEYWORDS derived in terms of linear matrix inequalities, which is simple and convenient for testing and application. Unfortunately, difficulties arise Markov chain when one attempts to derive the sufficient conditions and to extract Complete probability space the controller parameters for these systems. In fact, we have to cope Linear matrix inequality with stochastic process and disturbance. Indeed, the Lyapunov functional method is a powerful tool to stability analysis of Discrete-time system differential systems. However, this method is not effectively applied Stochastic control for stochastic systems because we do not know how to construct suitable Lyapunov functions and use them in these systems. To overcome the difficulties, we first introduced basic concepts of probability theory. Next, a new sufficient conditions of robust state boundedness for unforced stochastic systems was established. Finally, the result was applied to design controllers to guarantee robust state boundedness for stochastic control systems. MỘT VÀI KẾT QUẢ MỚI VỀ ƯỚC LƯỢNG TÍNH BỊ CHẶN BỀN VỮNG CỦA HỆ ĐIỀU KHIỂN NGẪU NHIÊN VỚI XÍCH MARKOVIAN RỜI RẠC Nguyễn Trường Thanh*, Nguyễn Thu Hằng, Phạm Ngọc Anh Trường Đại học Mỏ - Địa chất THÔNG TIN BÀI BÁO TÓM TẮT Ngày nhận bài: 05/3/2021 Bài báo này ước lượng tính bị chặn bền vững của hệ điều khiển ngẫu nhiên với xích Markovian rời rạc. Bằng cách sử dụng phương pháp Ngày hoàn thiện: 26/5/2021 hàm Lyapunov và lí thuyết xác suất, chúng tôi đề xuất một số điều Ngày đăng: 27/5/2021 kiện đủ mới để đảm bảo tính bị chặn bền vững cho hệ điều khiển ngẫu nhiên. Các điều kiện trên là các bất đẳng thức ma trận tuyến TỪ KHÓA tính có thể kiểm tra và dễ dàng sử dụng trong thực tế. Thật không may mắn, có nhiều khó khăn nảy sinh khi nghiên cứu các hệ này khi Xích Markov chúng ta phải đối mặt với các quá trình ngẫu nhiên và nhiễu không Không gian xác suất đầy đủ mong muốn. Thêm vào đó, phương pháp hàm Lyapunov là một công Bất đẳng thức ma trận tuyến tính cụ đầy hiệu quả khi nghiên cứu tính ổn định của các hệ phương trình vi phân. Tuy nhiên, phương pháp này không hiệu quả khi áp dụng Hệ rời rạc cho các hệ ngẫu nhiên do chúng ta không biết làm thế nào để cấu trúc Điều khiển ngẫu nhiên các hàm Lyapunov phù hợp và làm thế nào để sử dụng các hàm này cho các quá trình ngẫu nhiên. Để vượt qua các khó khăn trên, đầu tiên, chúng tôi giới thiệu các khái niệm cơ bản của lí thuyết ngẫu nhiên. Tiếp đó, chúng tôi thiết lập một điều kiện đủ mới về tính bị chặn bền vững cho hệ ngẫu nhiên không có điều khiển. Cuối cùng, kết quả này được áp dụng cho việc thiết kế điều khiển cho hệ ngẫu nhiên có điều khiển. DOI: https://doi.org/10.34238/tnu-jst.4101 * Corresponding author. Email: nguyentruongthanh@humg.edu.vn 67 Email: jst@tnu.edu.vn TNU Journal of Science and Technology 226(06): 67 - 72 1. Introduction Markovian jump systems belong to a significant class of hybrid systems which are described by the switches between subsystems and a finite-state Markov chain according to a known Markov law. Discrete-time Markovian jump systems arise in many practical processes subjected to random abrupt changes in the inputs, internal variables [1]-[4]. Examples of such systems with Markov chain are solar thermal central receivers, economic systems and manufacturing systems. Therefore, Markovian jump systems have attracted a lot of attention in many applications of signal processing, control theory and communications because of their flexibility to model real world phenomena [5], [6]. In recent years, considerable interest has been focused on many important problems in systems and control theory of Markovian jump systems. Based on Lyapunov’s second method, some sufficient conditions in terms of linear matrix inequalities for following problems are proposed as mean square stability of linear discrete-time systems [7], state bounding problem [8], [9], stabilisation for Markovian jump systems with state and input delays [10], and H control problems for linear Markovian jump systems with mode-dependent/independent delays [11] etc. Besides, disturbances is inherent characteristic of many physical systems and ubiquitous in dynamic systems. These disturbances are the unavoidable source of instability and poor performance. Hence, the problem of state bounding for Markovian jump systems with disturbances is a key topic in control engineering. However, to the best knowledge of authors, there are very few results on the problem for stochastic control system with disturbance input and discrete-time Markovian chain. This has motivated our research. The paper is organized as follows. Section 2 presents definitions and some well-known technical lemmas needed for the proof of the main results. A main result for designing controllers to ensure − mean square boundedness is presented in Section 3. The paper ends with conclusions. 2. Preliminaries and problem statement The following notations will be used throughout this paper: N denotes the set of all non- negative integer numbers R+ stands for the set of all non-negative real numbers; Rn denotes the n- dimensional space with the scalar product (,)x y= xT y and the vector norm ||x= xT x ; AT denotes the transpose of the matrix A and I denotes the identity matrix in Rn ; (A ), n max ()A stand for the maximal and the minimal real part of eigenvalues of A, respectively; min Q 0 means that Q is positive definite, .i.e., xT Qx 0 for all x 0; AB means AB− 0 ; is the expectation operator with respect to some complete probability space (,,P.F ) The symmetric terms in a matrix are denoted by *. Consider the discrete time stochastic control system described by the following equations: xk(+ 1) = Arxk ()()k + Brwk ()() k + CrukkN ()(), k r , k (2.1) x(0)= 0, where x () k R n is the state vector; uk()is the control; w() k Rs is the disturbance input; rk r be a discrete Markov chain with state space M= 1,2, ,m . The transition probabilities k k=0 m of r are given by Pr= j | r = i = p , where p 0 and p=1, i , j M . k k=0 ( k+1 k) ij ij ij j=1 A( r ), B ( r ), C ( r ) k k k are system matrices of appropriate dimensions in the finite set of 68 Email: jst@tnu.edu.vn TNU Journal of Science and Technology 226(06): 67 - 72 Ai,,,M B i C i i with Ai:= A (), i B i : = B (), i C i : = C (), i i M. The disturbance wk()satisfies the condition b 0: w ( k )2 b , k N . (2.2) Definition 2.1. ([8]) For a given 0 , system (2.1) is said to be − mean square bounded if every trajectory xk() of (2.1) satisfies E x ( k )2 | F , k N , 0 where F00= x(0), r be the σ-algebra generated by ( xr(0)= 0,0 ) . T Lemma 2.1. ([12]) Give constant matrices YY= 0 and XZ, . Then T T −1 XZ XZYZ+ 0 0. ZY− 3. Main results In this section, we will give sufficient conditions of − mean square boundedness for (2.1). We first establish a sufficient condition for unforced system (2.1). Then, we design controllers based on LMIs to guarantee − mean square boundedness for (2.1). Let us set m Qi= Q j p ij, Q j = Q ( j ), i , j M; j=1 (1− ) = ().Q min b Theorem 3.1. Give 0, (0,1), and a symmetric positive definite matrix Q. If there exist symmetric positive definite matrices Qii , M, satisfying the following LMI conditions for all i M, AQAQAQBTT− i i i i i i i 0, (3.1) T * BQBIi i i− s Q Q, i M, i (3.2) then unforced system (2.1) is − mean square bounded. Proof. Consider the following function Vxkr((),)= xkQrxk ()T ()(), k N . kk Suppose that r.= i Then, at time k +1, the mode r = j with probability p . k k+1 ij Firstly, we estimate +V( x ( k 1), rkk+1 ) | x ( k ), r as follows. It is easy to see that if we fix xk() and rik = , the value of x( k+ 1) = Aii x ( k ) + B w ( k ) is only dependent on ( x( k ), w ( k )) . Then V( x ( k + 1), r ) | x ( k ), r = i kk+1 is dependent on rk +1. It leads to 69 Email: jst@tnu.edu.vn TNU Journal of Science and Technology 226(06): 67 - 72 T Vxk(( + 1), rk++11 )|(), xkri k = = xk ( + 1) Qrxk ( k )( + 1)|(), xkri k = mm TTT =+xk(1) Qxkj (1)( +===+ Pr k+1 jri | k ) xk (1) Qxk j (1) +=+ p ij xk (1) Qxk i (1) + jj==11 T T =Axki() + Bwk i () QAxk i i () + Bwk i () − xkQxk () i () + Vxkr ((), k = i ) TT T AQAPAQB− xk() 2 =xkwk(),() i i i i i i i + wkVxkri () + ((),). = T wk() k * BQBIi i i− s From (3.1), we have 2 Vxk(( + 1), rk+1 )|(), xkri k = wk () + Vxkri ((), k = ), i M. Hence, because of (2.2), we obtain Vxk( ( + 1), rk+1 ) | xkr ( ), k b + Vxkr ( ( ), k ). (3.3) Next, we use the inequality (3.3) to evaluate V( x ( k ), rk ) | x (0), r0 , k N . From (3.3), we have the followings. For k = 0, Vxrxr( (1), ) | (0), bVxr + ( (0), ) = bxQrx + (0)T ( ) (0) = b . (3.4) 1 0 0 0 For k =1, V( x (2), r2 ) | x (1), r 1 b + V ( x (1), r 1 ). Consequently, Vxrxrxr( (2),2 ) | (1), 1 |( (0), 0 ) bVxrxr + ( (1), 1 ) |( (0), 0 ). Taking expectation both sides of the above inequality, we have Vxrxr( (2),2 ) | (0), 0 = ( Vxrxrxr ( (2), 2 ) | (1), 1 |( (0), 0 )) b + ( V( x (1), r10 ) |( x (0), r )) b + b = b( 1 + ) . Similarly, we obtain k−1 k−1 1− V( x ( k ), rk ) | x (0), r0 b( 1 + + + ) = b , k N . 1− Hence, b VxkrF( ( ), ) | = Vxkrxr ( ( ), ) | (0), , kN . (3.5) kk001− Since the condition (3.2), the following inequality holds TT 2 Vxkr((),ki= i ) = xkQxk () () xkQxk () () min ()(). Qxk Moreover, V((),) x k r ()(), Q x k2 k N . (3.6) k min Using (3.6) and the monotonicity of the operator , we have VxkrF((),)| ()()| QxkF22 = () Q xkF ()|. (3.7) k 0 min 0 min 0 Combining (3.5) and (3.7), we obtain 2 b 1 x( k ) | F = , 0 1− min (Q ) which completes the proof of the theorem. 70 Email: jst@tnu.edu.vn TNU Journal of Science and Technology 226(06): 67 - 72 The remaining of this section is to design controllers in the form of u()() k= L x k such rrkk that the system (2.1) is − mean square bounded. Let us denote m Qi= Q j p ij, Q j = Q ( j ), i , j M; j=1 =AQAAKCKCAQTT + +T − ; 11 i i i i i i i i i i =AQBCKB + T ; =BQBIT − . 12 i i i i i 22 i i i Theorem 3.2. Given 0, (0,1), and a symmetric positive definite matrix Q. If there exist symmetric positive definite matrices Qii , M, and matrices Kii , M, satisfying the following LMI conditions for all i M, T 11 12 KCii * 0 0, (3.8) 22 **()− QI min QQ , (3.9) i then system (2.1) is − mean square bounded with the controller. −1 u( k )= Q K x ( k ). i i i Proof. From (3.2) and mm Qi= Q j p ij, p ij = 1, p ij 0, jj==11 we have mm Qi= Q j p ij Qp ij = Q min ( Q) I 0, jj==11 it leads to min(QQi ) min ( ) 0. Consequently, −−1111 QQIII = . ii max ( ) Q min (Qi ) min ( ) Use the above inequality and (3.8) and Schur complement lemma (Lemma 2.1) with −1 L= Q K,, i M i i i we have TT T −1 ALCQALCQALCQBiii+ iiii + − i iii + ii +KCQKC = 11i i i i 12 * BQBIT − i i i * 22 (3.10) 1 T +KCKC 11 ()Q i i i 12 0, i M. min * 22 From (3.9) and (3.10), using Theorem 3.1, system (2.1) is − mean square bounded. This completes the proof of the theorem. 71 Email: jst@tnu.edu.vn TNU Journal of Science and Technology 226(06): 67 - 72 4. Conclusion In this paper, we have studied the robust state bounding estimation problem of stochastic control systems with discrete time Markovian jump. The proposed analytical tools used in the proof are based on Lyapunov functional method. The sufficient conditions for − mean square boundedness have been established in terms of LMIs. Acknowledgments The authors would like to thank the anonymous reviewers for their valuable comments and suggestions which allowed them to improve the paper. REFERENCES [1] N. Krasovskii and E. Lidskii, “Analysis Design of Controller in Systems with Random Attributes – Part 1,” Automation and Remote Control, vol. 22, pp. 1021-1025, 1961. [2] Y. Ji and H. Chizeck, “Controllability, Stabilizability, and Continuous-time Markovian Jump Linear Quadratic Control,” IEEE Transactions on Automatic Control, vol. 35, pp. 777-788, 1990. [3] M. D. S. Aliyu and E. K. Boukas, “Robust H control for Markovian jump nonlinear systems,” IMA J. Math.Control Inf., vol. 17, pp. 295-308, 2000. [4] N. T. Dzung and L. V. Hien, “Stochastic Stabilization of Discrete-Time Markov Jump Systems with Generalized Delay and Deficient Transition Rates,” Circuits, Systems, and Signal Processing, vol. 36, no. 6, pp. 2521-2541, 2017. [5] O. L. V. Costa, M. D. Fragoso, and R. P. Marques, Discrete-Time Markov Jump Linear Systems. London: Springer, 2005. [6] H. Shen, J. H. Park, L. Zhang, and Z. G. Wu, “Robust extended dissipative control for sampled- data Markov jump systems,” Int. J. Control, vol. 87, pp. 1549-1564, 2014. [7] C. Z. Souza, “Robust stability and stabilization of uncertain discrete-time Markovian jump linear systems,” IEEE Trans. Autom. Control, vol. 51, pp. 836-841, 2006. [8] L. V. Hien, N. T. Dzung, and H. B. Minh, “A novel approach to state bounding for discrete-time Markovian jump systems with interval time-varying delay,” IMA Journal of Mathematical Control and Information, vol. 33, no. 2, pp. 293-307, 2016. [9] L. V. Hien, N. T. Dzung, and H. Trinh, “Stochastic stability of nonlinear discrete-time Markovian jump systems with time-varying delay and partially unknown transition rates,” Neurocomputing, vol. 175, pp. 450-458, 2016. [10] B. Chen, H. Li, P. Shi, C. Lin, and Q. Zhou, “Delay-dependent Stability Analysis and Controller Synthesis for Markovian Jump Systems with State and Input Delays,” Information Sciences, vol. 179, pp. 2851–2860, 2009. [11] E. K. Boukas and Z. K. Liu, “Robust H control of discrete-time Markovian jump linear systems with mode-dependent time-delay,” IEEE Trans. Autom. Control, vol. 46, pp. 1918-1924, 2001. [12] S. Boyd, E. L. Ghaoui, E. Feron, and V. Balakrishnan, Linear Matrix Inequalities in System and Control Theory. SIAM, Philadelphia, 1994. 72 Email: jst@tnu.edu.vn
File đính kèm:
new_results_on_robust_state_bounding_estimation_for_discrete.pdf