An overview of facial attribute learning
Facial attributes are useful for developing applications such as face recognition, search, and
surveillance. They are therefore important for various facial analysis. Many facial attribute learning
algorithms have been developed to automatically detect those key attributes over the years. In this
paper, we have surveyed some typical facial attribute learning methods. Five major categories of the
state-of-the-art methods are identified: (1) Traditional learning, (2) Deep Single Task Learning, (3)
Deep Multitask Learning, (4) Imbalanced Data Solver, and (5) Facial Attribute Ontology. They
included from traditional learning algorithm to deep learning, along with methods that assist in
solving semantic gaps based on ontology and solving data imbalances. For each algorithm of
category, basic theories as well as their strengths, weaknesses, and differences are discussed. We also
compared their performance on the standard datasets. Finally, based on characteristics and
contribution of methods, we present conclusion and future works to solve facial attributes learning.
The survey can help researchers gain a quick overview to build future human face applications as
well as further studies.
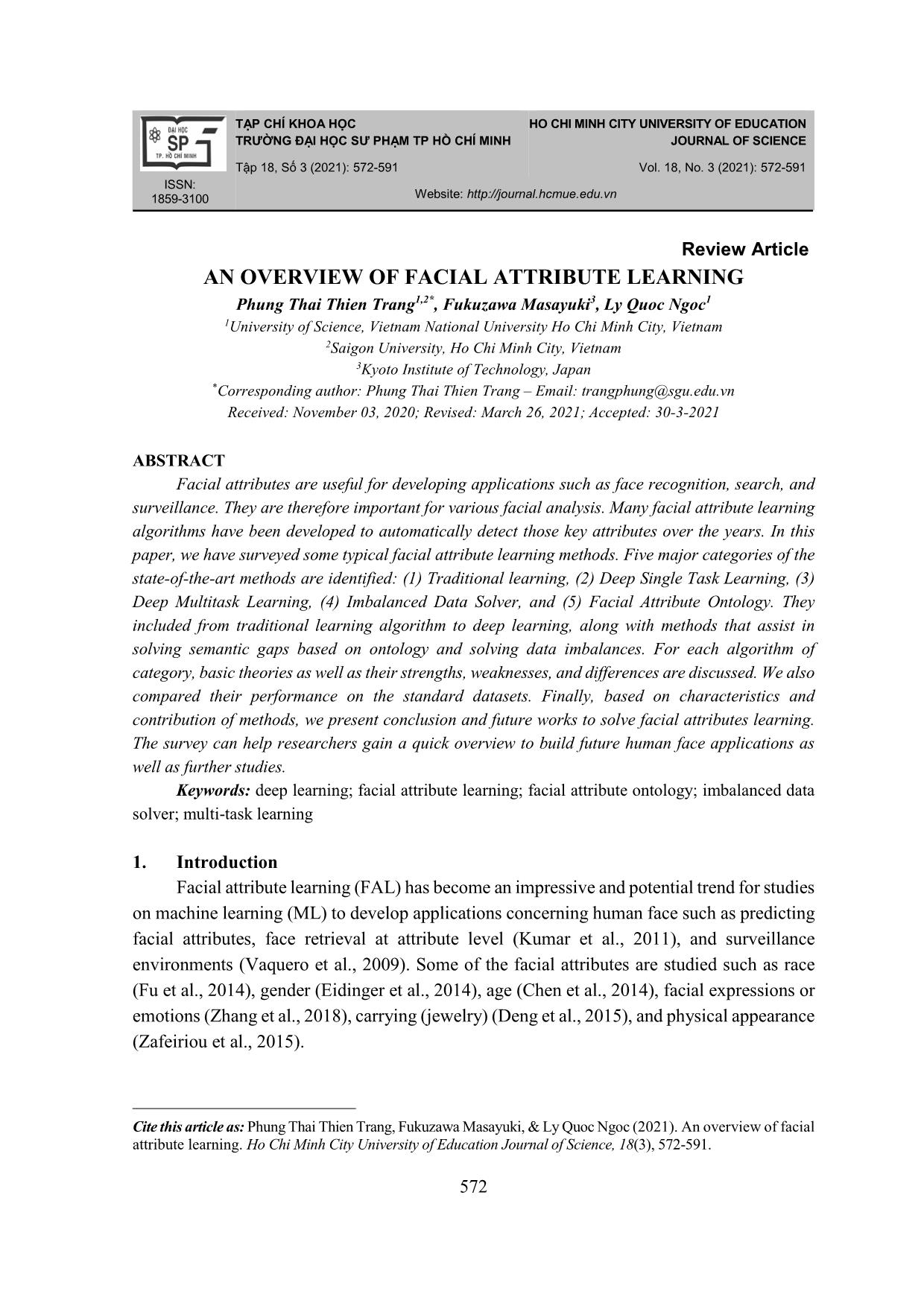
Trang 1
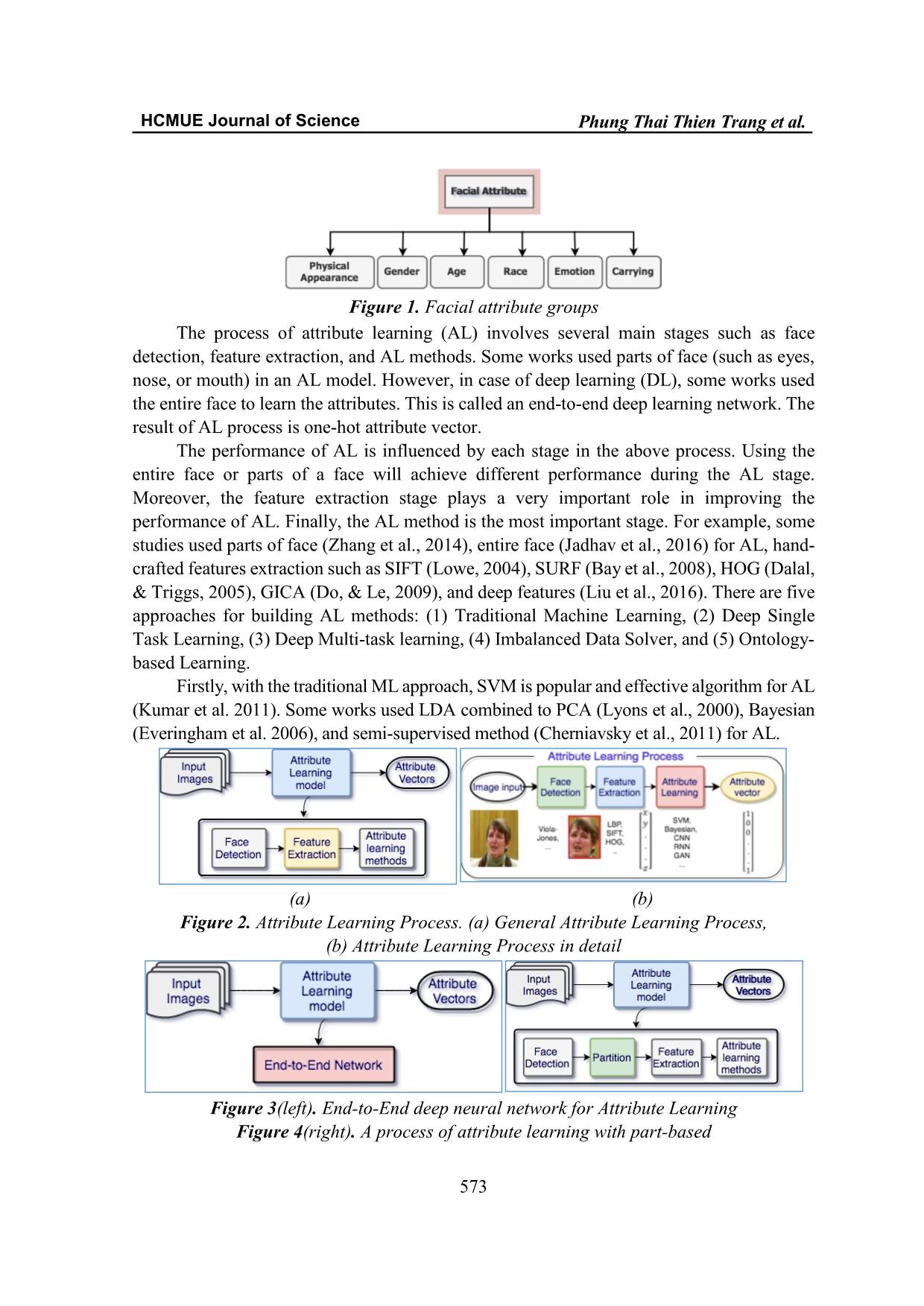
Trang 2
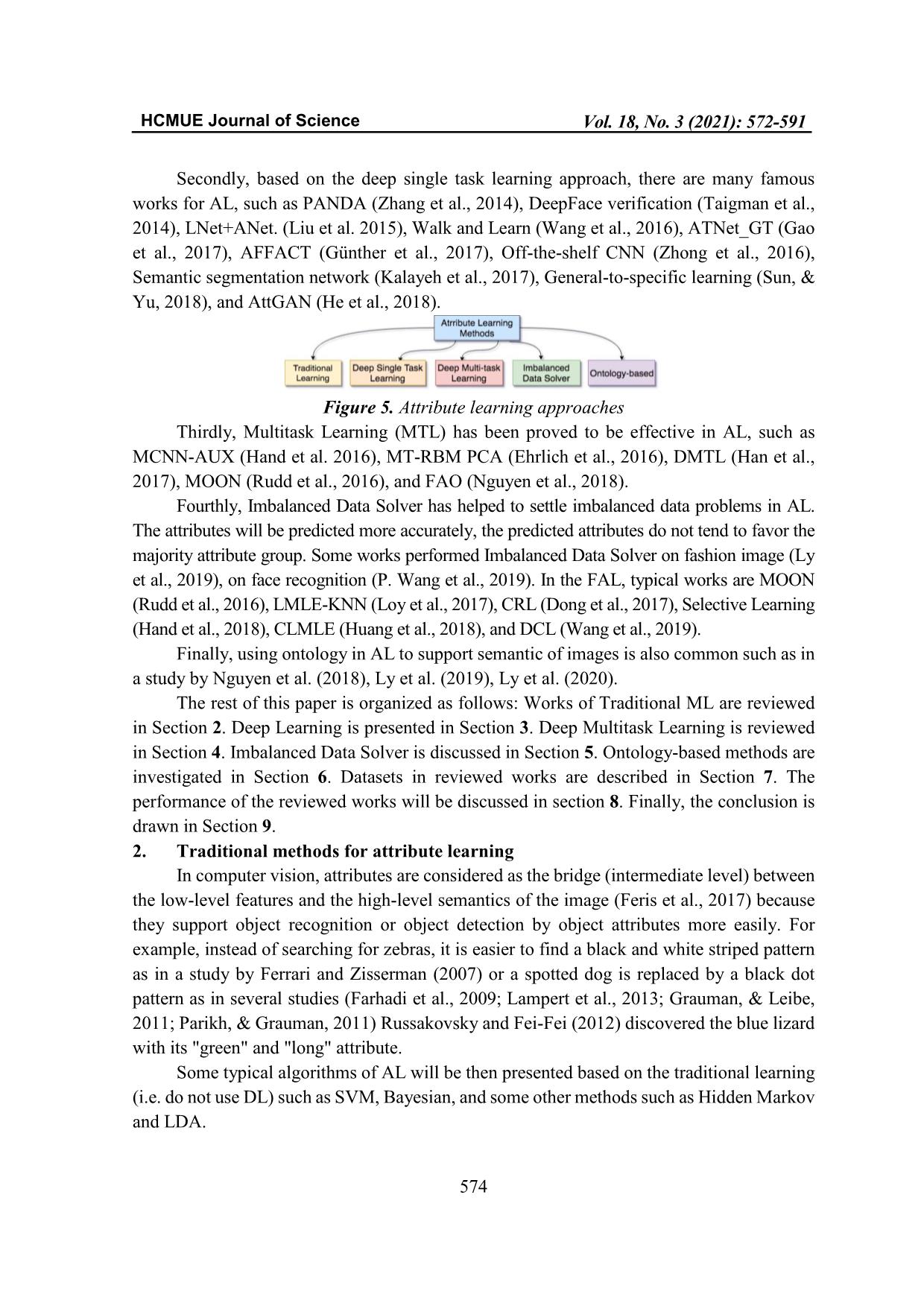
Trang 3
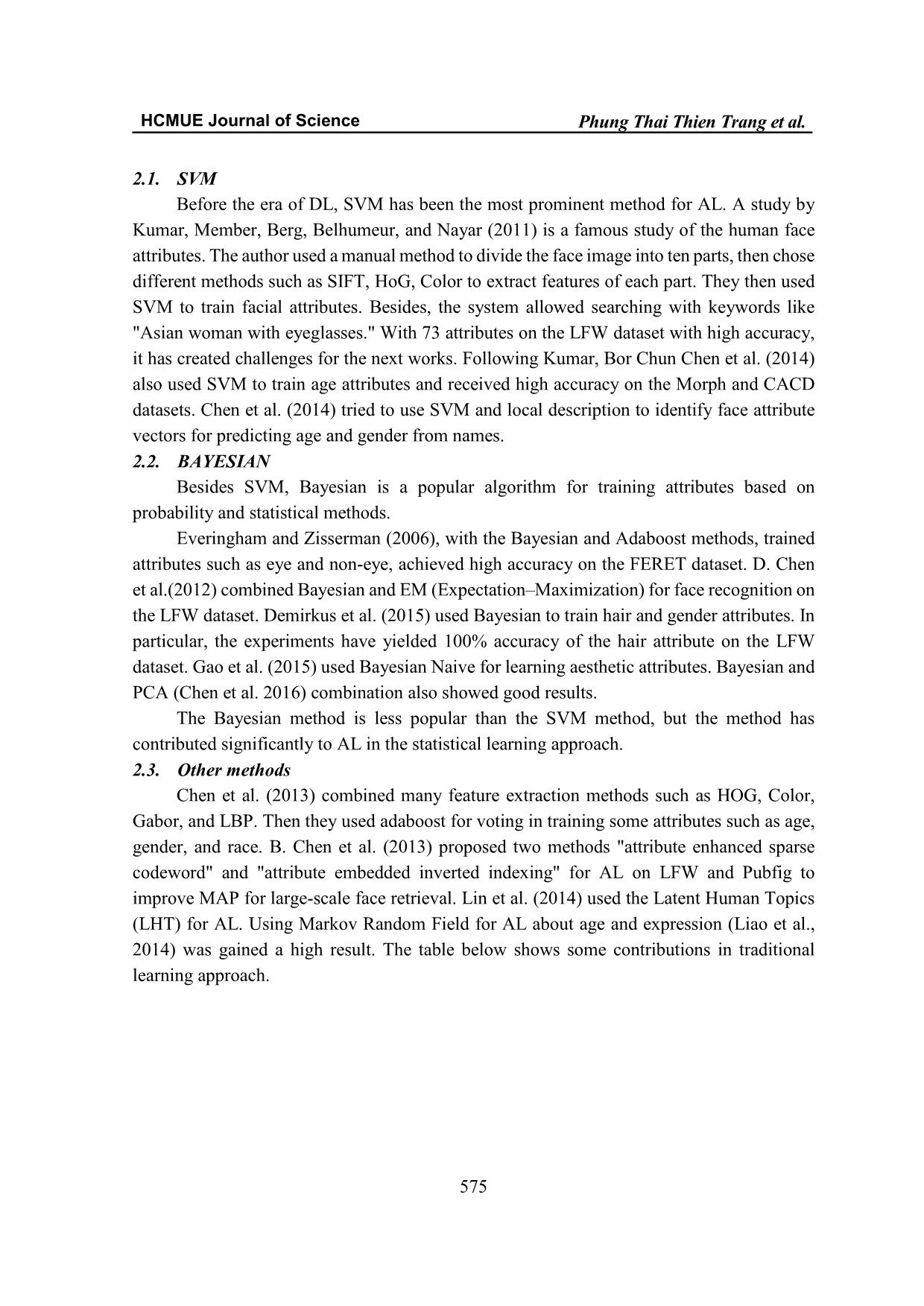
Trang 4
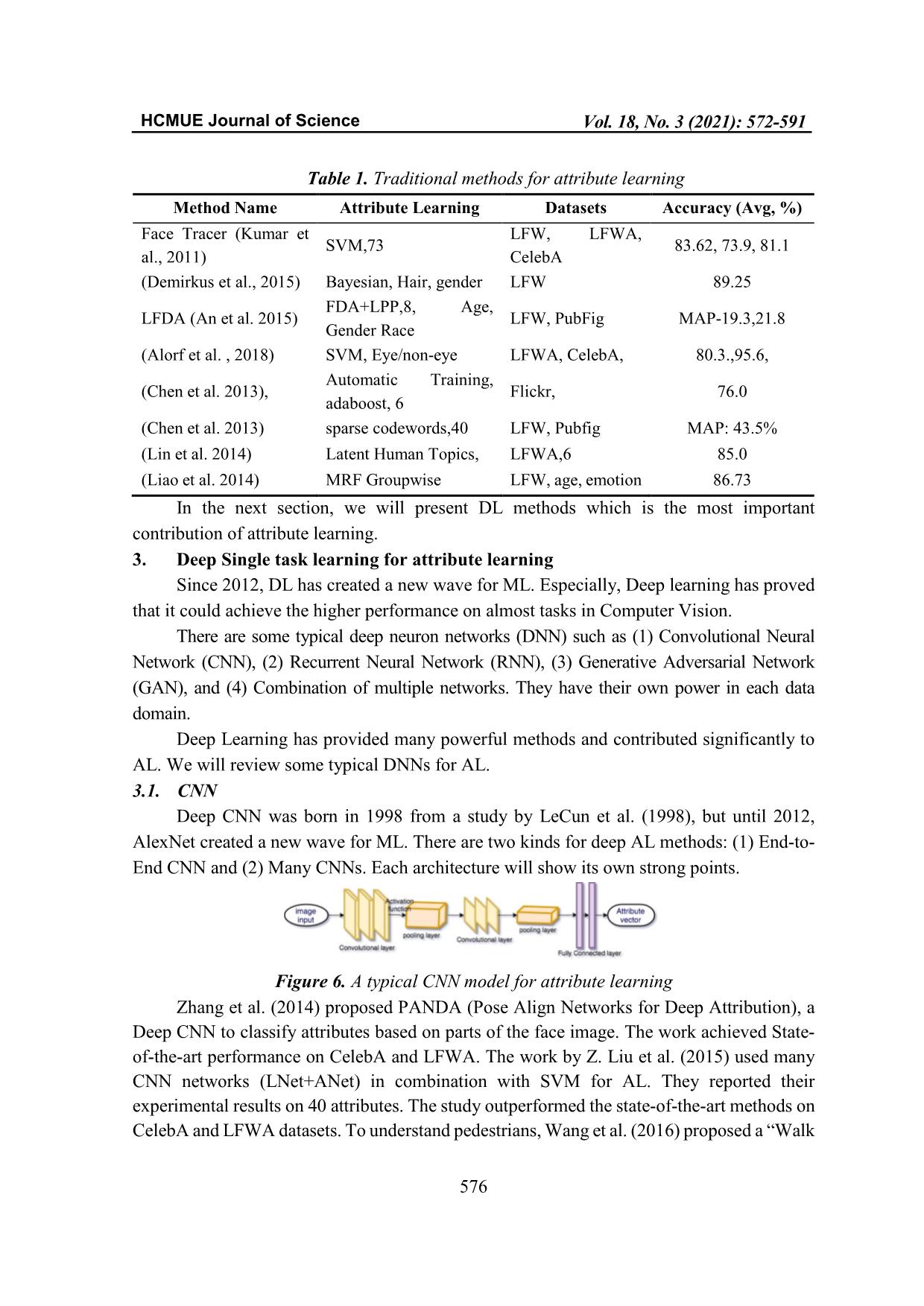
Trang 5
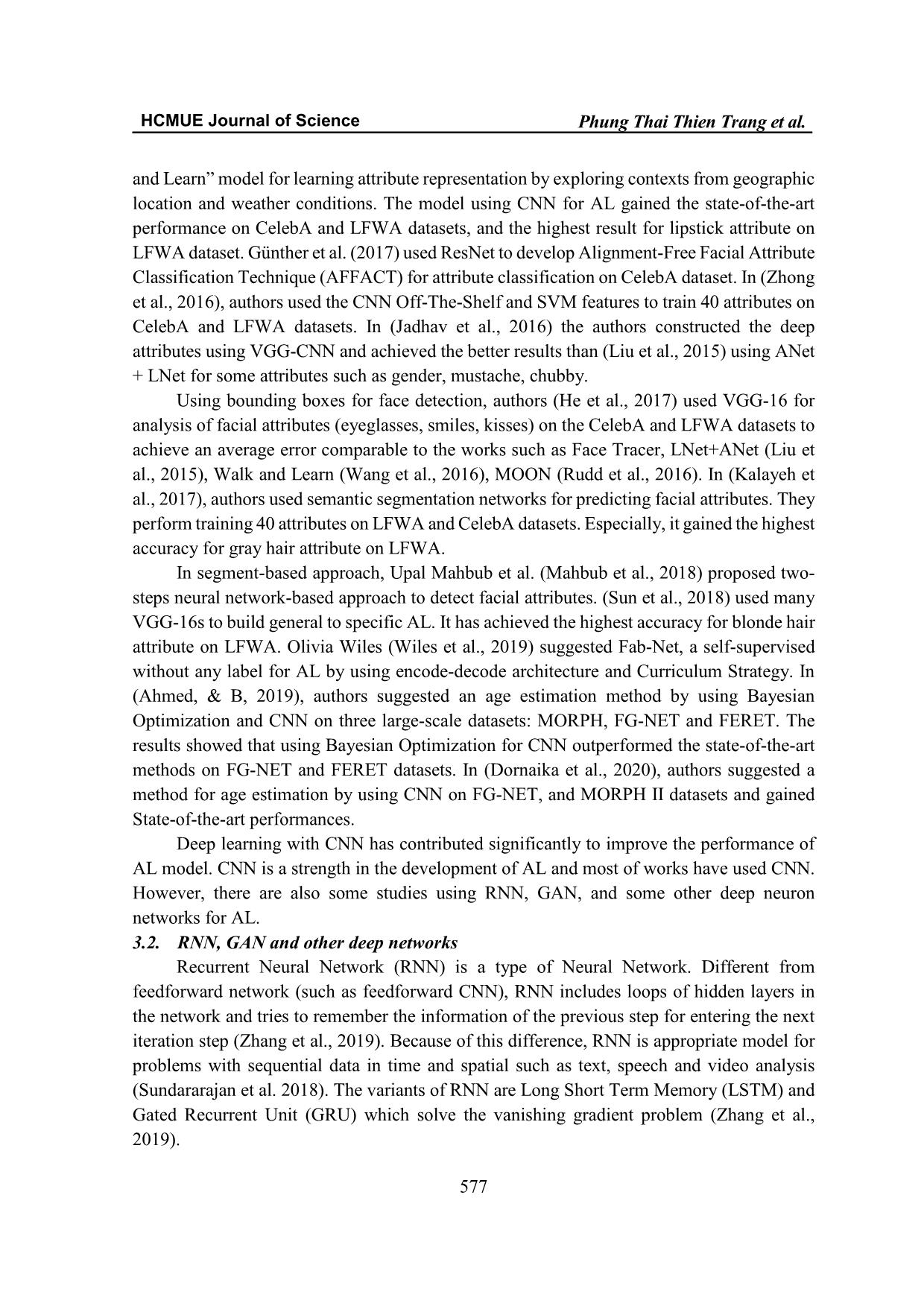
Trang 6
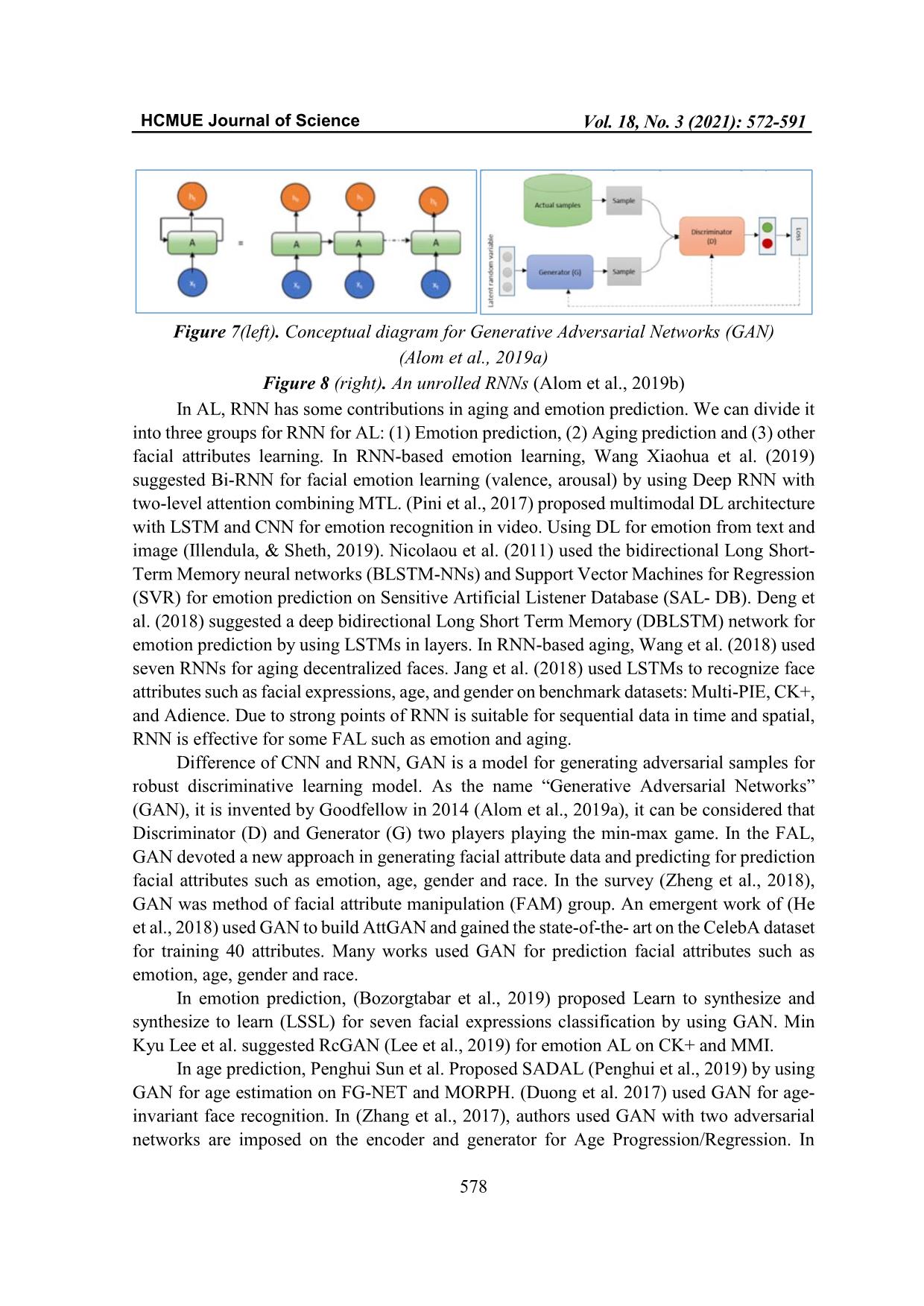
Trang 7
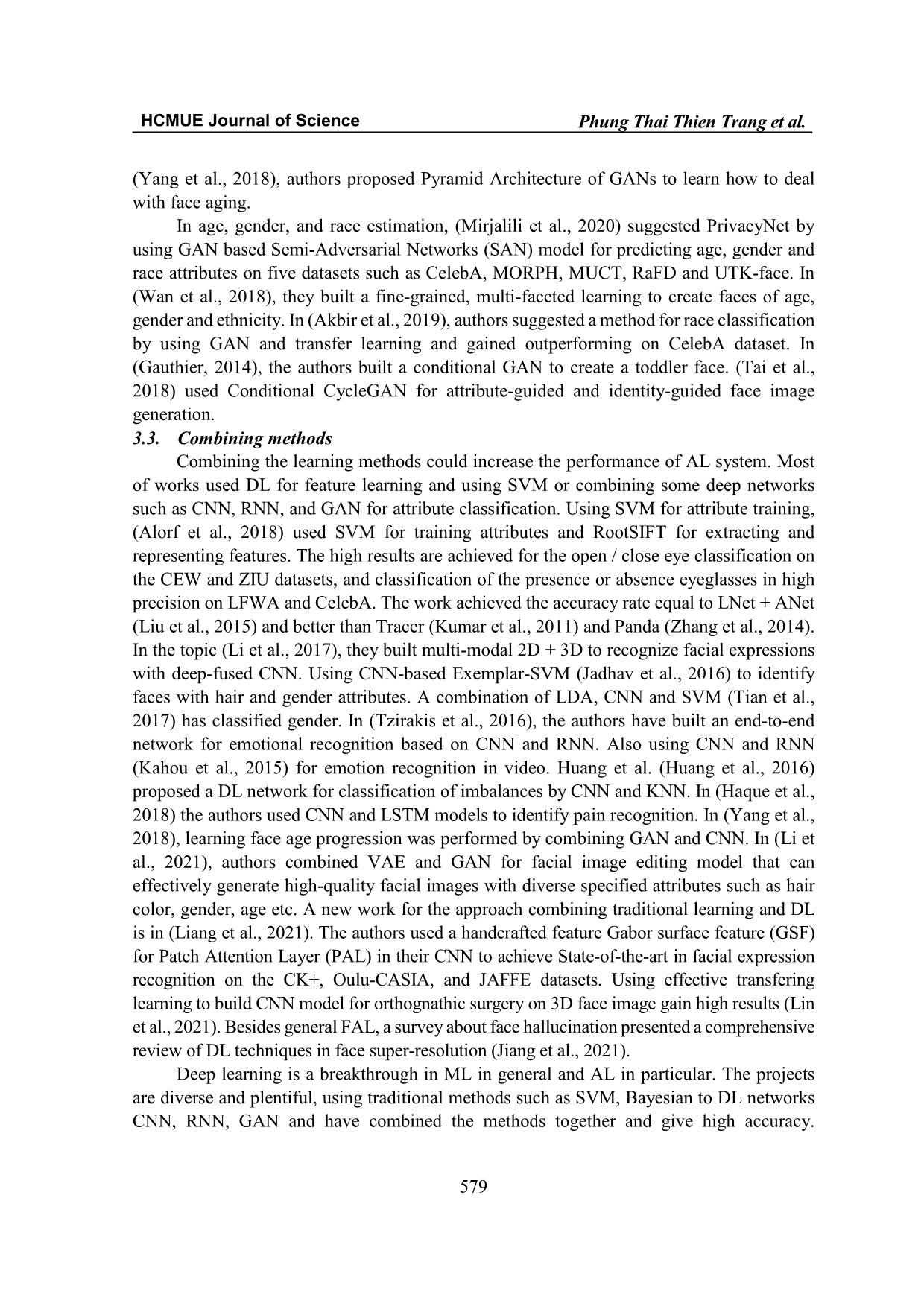
Trang 8
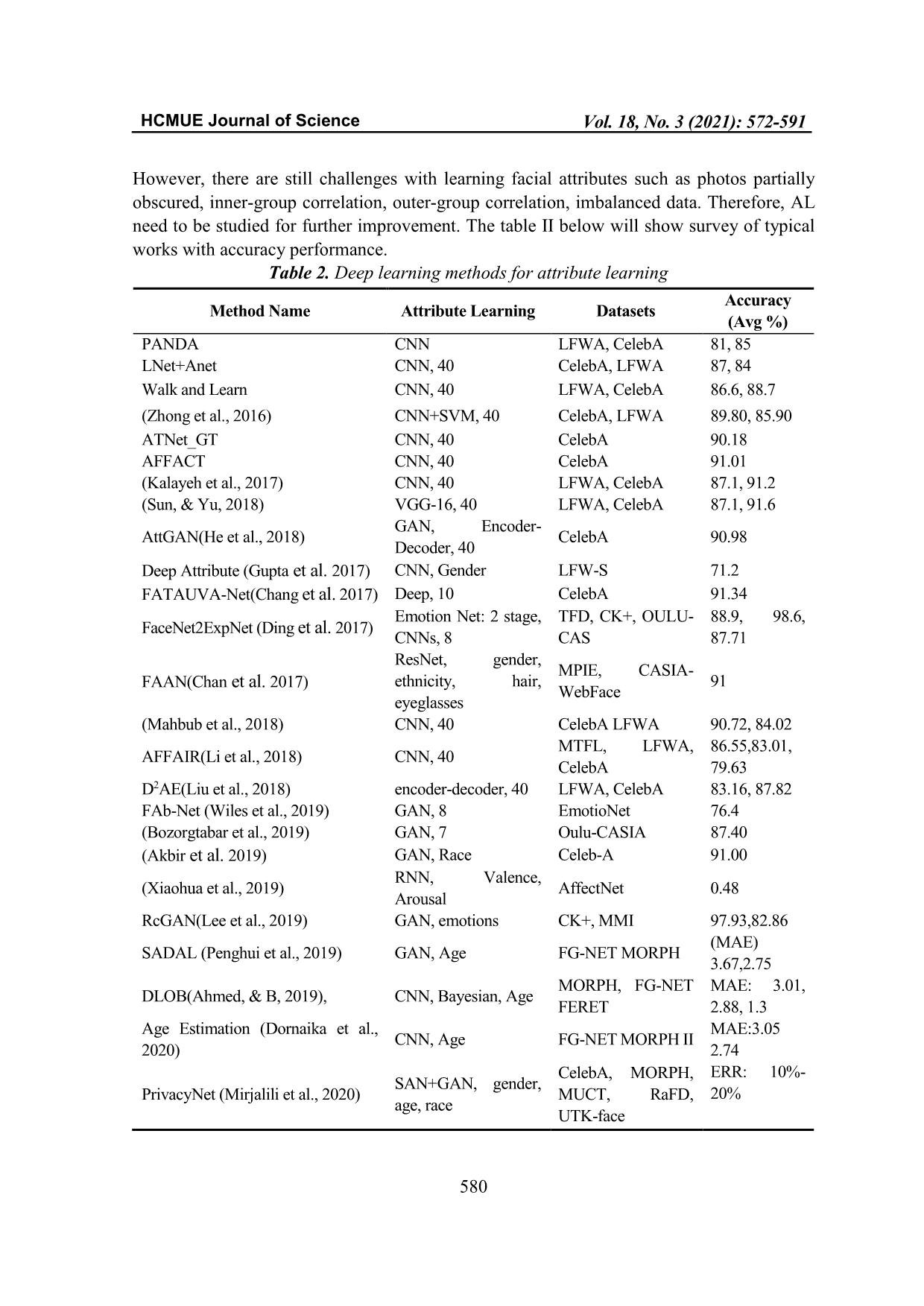
Trang 9
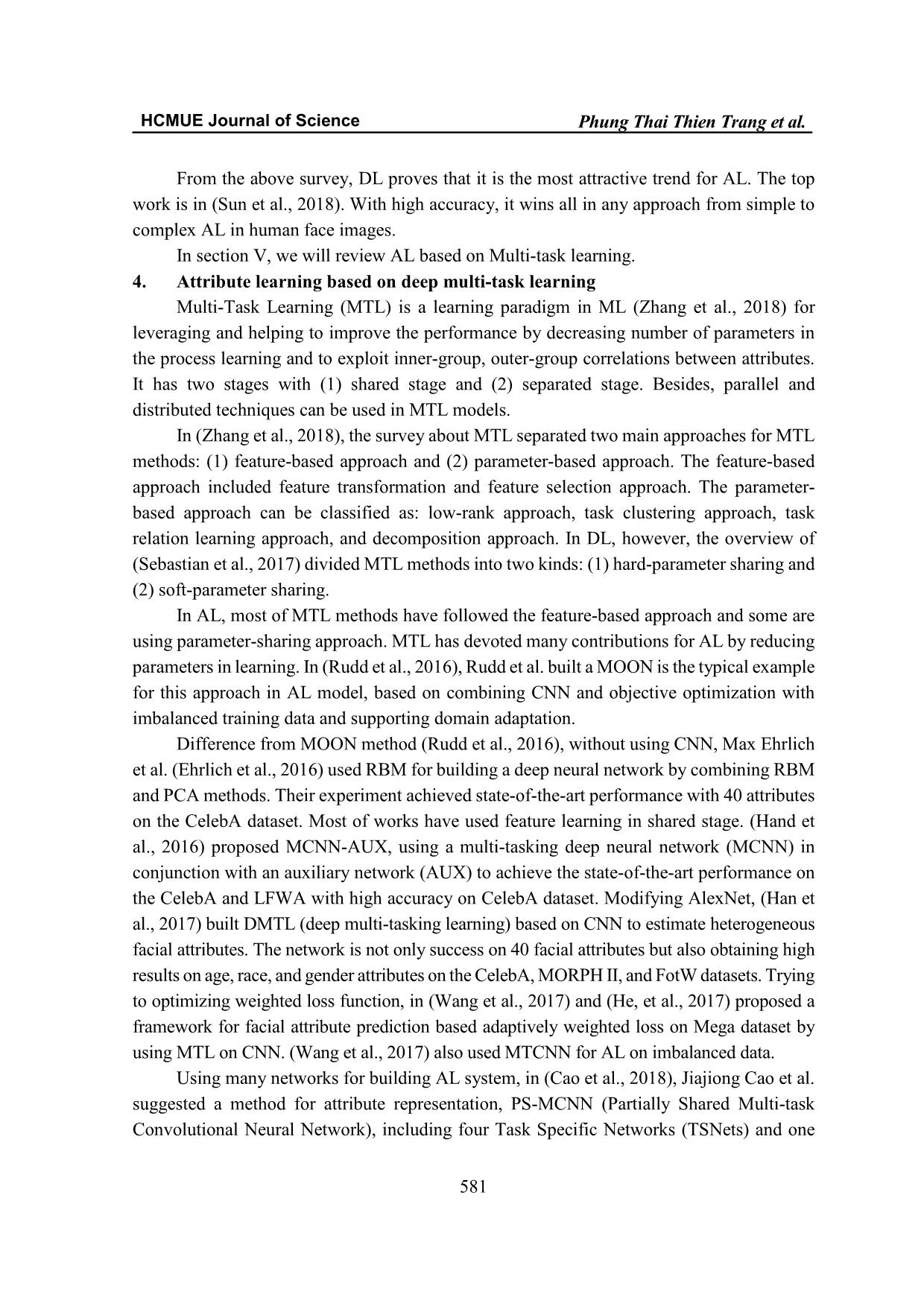
Trang 10
Tải về để xem bản đầy đủ
Tóm tắt nội dung tài liệu: An overview of facial attribute learning
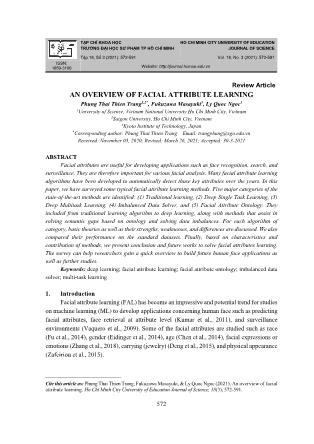
i.org/10.1109/ICIT.2019.8755180 Gao, Z., & Wang, S. (2015). Multiple Aesthetic Attribute Assessment by Exploiting Relations Among Aesthetic Attributes, 575-578. Gauthier, J. (2014). Conditional generative adversarial nets for convolutional face generation. Class Project for Stanford CS231N: Convolutional Neural Networks for Visual Recognition, Winter Semester 2014. Gruber, T. R. (1993). Toward principles for the design of ontologies used for knowledge sharing. International Journal of Human - Computer Studies, 43(5-6), 907-928. Günther, M., Rozsa, A., & Boult, T. E. (2017). AFFACT - Alignment Free Facial Attribute Classification Technique. Fg, 90-99. Gupta, N., Gupta, A., Joshi, V., Subramaniam, L. V., & Mehta, S. (2017). Deep Attribute Driven Image Similarity Learning Using Limited Data. Proceedings - 2017 IEEE International Symposium on Multimedia, ISM 2017, 2017-Janua, 146-153. Han, H., Jain, A. K., Shan, S., & Chen, X. (2017). Heterogeneous Face Attribute Estimation: A Deep Multi-Task Learning Approach. Proc. 12th IEEE Int. Conf. Autom. Face Gesture Recognit., 8828(c), 1-14. https://doi.org/10.1109/TPAMI.2017.2738004 Hand, E. M., Castillo, C., & Chellappa, R. (2018). Doing the best we can with what we have: Multi- label balancing with selective learning for attribute prediction. 32nd AAAI Conference on Artificial Intelligence, AAAI 2018, 6878-6885. Hand, E. M., & Chellappa, R. (2016). Attributes for Improved Attributes: A Multi-Task Network for Attribute Classification, 8057–8058. Retrieved from Haque, M. A., Bautista, R. B., Noroozi, F., Kulkarni, K., Laursen, C. B., Irani, R., Moeslund, T. B. (2018). Deep Multimodal Pain Recognition : A Database and Comparison of Spatio- Temporal Visual Modalities. IEEE International Conference on Automatic Face & Gesture Recognition (FG 2018), 250-257. https://doi.org/10.1109/FG.2018.00044 He, H., & Garcia, E. A. (2009). Learning from imbalanced data. Ieee Transactions On Knowledge And Data Engineering, 21(9), 1263-1284. He, K., Fu, Y., & Xue, X. (2017). A Jointly Learned Deep Architecture for Facial Attribute Analysis and Face Detection in the Wild. Retrieved from He, K., Wang, Z., Fu, Y., Feng, R., Jiang, Y. G., & Xue, X. (2017). Adaptively weighted multi-task deep network for person atribute classification. 2017 ACM Multimed. Conf., 1636-1644. He, Z., Zuo, W., Member, S., Kan, M., Shan, S., Member, S., & Chen, X. (2018). AttGAN : Facial Attribute Editing by Only Changing What You Want, 1-16. Hsieh, H.-L., Hsu, W., & Chen, Y.-Y. (2017). Multi-task learning for face identification and attribute estimation. IEEE International Conference on Acoustics, Speech and Signal Processing (ICASSP), 2981-2985. Hsieh, H.-L., Hsu, W., & Chen, Y.-Y. (2017). Multi-task learning for face identification and attribute estimation, 1, 2981-2985. Huang, C., Li, Y., Loy, C. C., & Tang, X. (2016). Learning Deep Representation for Imbalanced Classification. IEEE Conference on Computer Vision and Pattern Recognition (CVPR), 6. 588 HCMUE Journal of Science Phung Thai Thien Trang et al. Huang, C., Li, Y., Loy, C. C., & Tang, X. (2018). Deep Imbalanced Learning for Face Recognition and Attribute Prediction, 1-14. Retrieved from Hudelot, C. (2008). Towards a Cognitive Vision Platform for Semantic Image Interpretation; Application to the Recognition of Biological Organisms, 280. Hupont, I., & Fernández, C. (2019). DemogPairs: Quantifying the impact of demographic imbalance in deep face recognition. Proc. - 14th IEEE Int. Conf. FG 2019. Illendula, A., & Sheth, A. (2019). Multimodal emotion classification. The Web Conference 2019 - Companion of the World Wide Web Conference, WWW 2019, 2, 439-449. Jadhav, A., Namboodiri, V. P., & Venkatesh, K. S. (2016). Deep Attributes for One-Shot Face Recognition. ECCV Workshops, (3), 516-523. https://doi.org/10.1007/978-3-319-49409-8_44 Jiang, J., Wang, C., Liu, X., & Ma, J. (2021). Deep Learning-based Face Super-resolution: A Survey. Retrieved from Johnson, J. M., & Khoshgoftaar, T. M. (2019). Survey on deep learning with class imbalance. Journal of Big Data, 6(1). https://doi.org/10.1186/s40537-019-0192-5 Kahou, S. E., Michalski, V., Konda, K., Memisevic, R., & Pal, C. (2015). Recurrent neural networks for emotion recognition in video. ICMI 2015 - Proceedings of the 2015 ACM International Conference on Multimodal Interaction, 467-474. Kalayeh, M. M., Gong, B., & Shah, M. (2017). Improving Facial Attribute Prediction using Semantic Segmentation, 6942-6950. https://doi.org/10.1109/CVPR.2017.450 Kumar, N., Member, S., Berg, A. C., Belhumeur, P. N., & Nayar, S. K. (2011). Describable Visual Attributes for Face Verification and Image Search, 1-17. Lee, M. K., Choi, D. Y., & Song, B. C. (2019). Facial expression recognition via relation-based conditional generative adversarial network. ICMI 2019 - Proceedings of the 2019 International Conference on Multimodal Interaction, 35-39. LeCun, Y., Bottou, L., Bengio, Y., Haffner, P. (1998). Gradient-Based Learning Applied to Document Recognition. Proceedings of the IEEE, 86, 2278-2324. Li, D., Zhang, M., Zhang, L., Chen, W., & Feng, G. (2021). A novel attribute-based generation architecture for facial image editing. Multimedia Tools and Applications, 80(4), 4881-4902. Li, H., Sun, J., & Xu, Z. (2017). Multimodal 2D + 3D Facial Expression Recognition with Deep Fusion Convolutional Neural Network, 9210(c), 1-16. Li, J., Zhao, F., Feng, J., Roy, S., Yan, S., & Sim, T. (2018). Landmark free face attribute prediction. IEEE Transactions on Image Processing, 27(9), 4651-4662. Li, Y., Wang, Q., Nie, L., & Cheng, H. (2017). Face Attributes Recognition via Deep Multi-Task Cascade. Proc. 2017 Int. Conf. Data Mining, Commun. Inf. Technol. - DMCIT ’17, 5-9. Liang, X., Xu, L., Liu, J., Liu, Z., Cheng, G., Xu, J., & Liu, L. (2021). Patch attention layer of embedding handcrafted features in CNN for facial expression recognition. Sensors Liao, S., Shen, D., & Chung, A. C. S. (2014). A Markov Random Field Groupwise Registration Framework for Face Recognition, 36(4). Lin, C.-H., Chen, Y.-Y., Chen, B.-C., Hou, Y.-L., & Hsu, W. (2014). Facial Attribute Space Compression by Latent Human Topic Discovery. Proc. ACM Int. Conf. Multimed. - MM ’14, Lin, H. H., Chiang, W. C., Yang, C. T., Cheng, C. T., Zhang, T., & Lo, L. J. (2021). On construction of transfer learning for facial symmetry assessment before and after orthognathic surgery. Computer Methods and Programs in Biomedicine, 200. Liu, Y., Wei, F., Shao, J., Sheng, L., Yan, J., & Wang, X. (2018). Exploring Disentangled Feature Representation Beyond Face Identification, 2080-2089. Liu, Z., Luo, P., Wang, X., & Tang, X. (2015). Deep learning face attributes in the wild. Proceedings of the IEEE International Conf on Computer Vision, 2015 Inter, 3730-3738. Loy, C. C., Luo, P., & Huang, C. (2017). Deep Learning Face Attributes for Detection and Alignment. https://doi.org/10.1007/978-3-319-50077-5 Ly, N. Q., Do, T. K., & Nguyen, B. X. (2019). Large-scale coarse-to-fine object retrieval ontology 589 HCMUE Journal of Science Vol. 18, No. 3 (2021): 572-591 and deep local multitask learning. Computational Intelligence and Neuroscience, 2019. Ly, N. Q., Cao, H. N.M., Nguyen, T. T (2020). Person Re-Identification System at Semantic Level based on Pedestrian Attributes Ontology. International Journal of Advanced Computer Science and Applications (IJACSA), 11(2), 2020. Mahbub, U., Sarkar, S., & Chellappa, R. (2018). Segment-based Methods for Facial Attribute Detection from Partial Faces, 1-13. Retrieved from Maillot, N. (2005). Ontology Based Object Learning and Recognition. Matthews, B. W. (1975). Comparison of the predicted and observed secondary structure of T4 phage lysozyme. BBA - Protein Structure, 405(2), 442-451. Mezaris, V., Kompatsiaris, I., & Strintzis, M. G. (2004). An ontology approach to object-based image retrieval, II-511-514. https://doi.org/10.1109/icip.2003.1246729 Mirjalili, V., Raschka, S., & Ross, A. (2020). PrivacyNet: Semi-Adversarial Networks for Multi- attribute Face Privacy, 1-3. Retrieved from Nguyen, H. M., Ly, N. Q., & Phung, T. T. T. (2018). Large-Scale Face Image Retrieval System at attribute level based on Facial Attribute Ontology and Deep Neuron Network. Penghui, S., Hao, L., Xin, W., Zhenhua, Y., & Wu, S. (2019). Similarity-aware deep adversarial learning for facial age estimation. Proc. - IEEE Int. Conf. Multimed. Expo, 2019-July. Pini, S., Ahmed, O. Ben, Cornia, M., Baraldi, L., Cucchiara, R., & Huet, B. (2017). Modeling Multimodal Cues in a Deep Learning-based Framework for Emotion Recognition in the Wild. Proceedings of the 19th ACM International Conference on Multimodal Interaction. Rudd, E. M., Günther, M., & Boult, T. E. (2016). MOON: A mixed objective optimization network for the recognition of facial attributes. Lecture Notes in Computer Science (Including Subseries Lecture Notes in Artificial Intelligence and Lecture Notes in Bioinformatics), 9909 Ruder, S. (2017). An Overview of Multi-Task Learning in Deep Neural Networks, (May). Retrieved from Sun, Y., & Yu, J. (2018). General-to-specific learning for facial attribute classification in the wild. J. Vis. Commun. Image Represent., 56, 83-91. https://doi.org/10.1016/j.jvcir.2018.09.003 Sundararajan, K., & Woodard, D. L. (2018). Deep learning for biometrics: A survey. ACM Computing Surveys, 51(3). https://doi.org/10.1145/3190618 Taherkhani, F., Nasrabadi, N. M., & Dawson, J. (2018). A Deep Face Identification Network Enhanced by Facial Attributes Prediction, 666-673. Tian, Q., Arbel, T., & Clark, J. J. (2017). Deep LDA-Pruned Nets for Efficient Facial Gender Classification. https://doi.org/10.1109/CVPRW.2017.78 Tzirakis, P., Trigeorgis, G., Nicolaou, M. A., Schuller, B., & Zafeiriou, S. (2016). End-to-End Multimodal Emotion Recognition using Deep Neural Networks, 14(8), 1-9. Wan, L., Wan, J., Jin, Y., Tan, Z., & Li, S. Z. (2018). Fine-grained multi-attribute adversarial learning for face generation of age, gender and ethnicity. Proceedings - 2018 International Conference on Biometrics, ICB 2018, 98-103. https://doi.org/10.1109/ICB2018.2018.00025 Wang, J., Cheng, Y., & Feris, R. S. (2016). Walk and Learn: Facial Attribute Representation Learning from Egocentric Video and Contextual Data. Wang, P., Su, F., & Zhao, Z. (2017). Joint Multi-Feature Fusion and Attribute Relationships for Facial Attribute Prediction, 3-6. Wang, P., Su, F., Zhao, Z., Guo, Y., Zhao, Y., & Zhuang, B. (2019). Deep class-skewed learning for face recognition. Neurocomputing, 363, 35-45. Wang, S., Yin, S., Hao, L., & Liang, G. (2021). Multi-task face analyses through adversarial learning. Pattern Recognition, 114, 107837. https://doi.org/10.1016/j.patcog.2021.107837 Wang, Y., Gan, W., Yang, J., Wu, W., & Yan, J. (2019). Dynamic Curriculum Learning for Imbalanced Data Classification, (2), 5017-5026. Wang, Z., He, K., & Fu, Y. (2017). Multi-task Deep Neural Network for Joint Face Recognition and Facial Attribute Prediction. ICMR’17, 365-374. 590 HCMUE Journal of Science Phung Thai Thien Trang et al. Wiles, O., Sophia Koepke, A., & Zisserman, A. (2019). Self-supervised learning of a facial attribute embedding from video. British Machine Vision Conference 2018, BMVC 2018. Xiao, T., Tsai, Y.-H., Sohn, K., Chandraker, M., & Yang, M.-H. (2019). Adversarial Learning of Privacy-Preserving and Task-Oriented Representations. Xiaohua, W., Muzi, P., Lijuan, P., Min, H., Chunhua, J., & Fuji, R. (2019). Two-level attention with two-stage multi-task learning for facial emotion recognition. J. Vis. Commun. Image Represent., 62, 217-225. https://doi.org/10.1016/j.jvcir.2019.05.009 Xu, M., Chen, F., Li, L., Shen, C., Lv, P., Zhou, B., & Ji, R. (2018). Bio-Inspired Deep Attribute Learning Towards Facial Aesthetic Prediction. IEEE Transactions on Affective Computing. Yang, H., Huang, D., Wang, Y., & Jain, A. K. (2018). Learning Face Age Progression : A Pyramid Architecture of GANs. CVPR, 31-39. Zhang, N., Paluri, M., Ranzato, M. A., Darrell, T., Bourdev, L., & Berkeley, U. C. (2014). PANDA : Pose Aligned Networks for Deep Attribute Modeling. Zhang, Y., & Yang, Q. (2018). A Survey on Multi-Task Learning, 1-20. Zhang, Z., Song, Y., & Qi, H. (2017). Age Progression / Regression by Conditional Adversarial Autoencoder, 5810-5818. Zheng, X., Guo, Y., Huang, H., Li, Y., & He, R. (2018). A Survey to Deep Facial Attribute Analysis. Retrieved from Zhong, Y., Sullivan, J., & Li, H. (2016). Leveraging mid-level deep representations for predicting face attributes in the wild. Proceedings - ICIP, 2016-Augus, 3239-3243. TỔNG QUAN VỀ PHƯƠNG PHÁP HỌC THUỘC TÍNH MẶT NGƯỜI Phùng Thái Thiên Trang1,2*, Fukuzawa Masayuki3, Lý Quốc Ngọc1,2 1Trường Đại học Khoa học Tự nhiên, Đại học Quốc gia Thành phố Hồ Chí Minh, Việt Nam 2Trường Đại học Sài Gòn, Việt Nam 3Học viện Kỹ thuật Kyoto, Nhật Bản *Tác giả liên hệ: Phùng Thái Thiên Trang – Email: trangphung@sgu.edu.vn Ngày nhận bài: 03-11-2020; ngày nhận bài sửa: 26-3-2021; ngày duyệt đăng: 30-03-2021 TÓM TẮT Thuộc tính mặt người là thông tin hữu ích cho việc xây dựng các ứng dụng như nhận dạng, tìm kiếm và giám sát khuôn mặt người. Do đó, chúng rất quan trọng đối với các nhiệm vụ phân tích khuôn mặt khác nhau. Nhiều thuật toán học thuộc tính khuôn mặt người đã và đang được phát triển để tự động phát hiện các thuộc tính trong nhiều năm qua. Trong bài báo này, chúng tôi khảo sát một số phương pháp điển hình về học thuộc tính khuôn mặt người. Chúng tôi chia ra năm loại chính của các phương pháp: (1) Học truyền thống, (2) Học sâu đơn nhiệm, (3) Học sâu đa nhiệm, (4) Giải quyết vấn đề mất cân bằng dữ liệu và (5) Thuộc tính khuôn mặt dựa vào phả hệ tri thức. Các phương pháp bao gồm từ học truyền thống đến học sâu, cùng với các phương pháp hỗ trợ giải quyết bài toán lỗ hổng ngữ nghĩa dựa trên phả hệ tri thức và giải quyết sự mất cân bằng dữ liệu. Đối với mỗi phương pháp trong mỗi loại, chúng tôi thảo luận về các lí thuyết cơ bản cũng như điểm mạnh, điểm yếu và sự khác biệt của chúng. Chúng tôi cũng so sánh hiệu suất của chúng trên bộ dữ liệu tiêu chuẩn. Cuối cùng, dựa trên đặc điểm và đóng góp của các phương pháp, chúng tôi đưa ra kết luận và hướng nghiên cứu trong tương lai để giải quyết vấn đề học thuộc tính khuôn mặt. bài khảo sát này sẽ giúp các nhà nghiên cứu có góc nhìn tổng quan nhanh để xây dựng các ứng dụng khuôn mặt người trong tương lai cũng như các nghiên cứu mới. Từ khóa: học sâu; học thuộc tính mặt người; học đa nhiệm; sự mất cân bằng dữ liệu; phả hệ tri thức 591
File đính kèm:
an_overview_of_facial_attribute_learning.pdf