From microgrids to smartgrids
To facilitate the integration of renewable energy resources (RES) into the
grid, a concept of smart grid and microgrid is used. The smart grid uses digital
technology to improve reliability, flexibility, and efficiency (both economic and
energy) of the electric system. This paper presents a development of stochastic tool
to assess impacts of RES integration. Intelligent strategies to control voltage and
frequency in a microgrid are also proposed. Energy management strategies for a
gridconnected or isolated microgrid are developed by using dynamic programming
or multiagent system. Then strategies for optimal charging of electric vehicles and
for smart energy management in buildings are shown. Finally, the interoperability of
microgrids is presented. Proposed solutions are evaluated by tests or demonstrations
at the CEAINES.
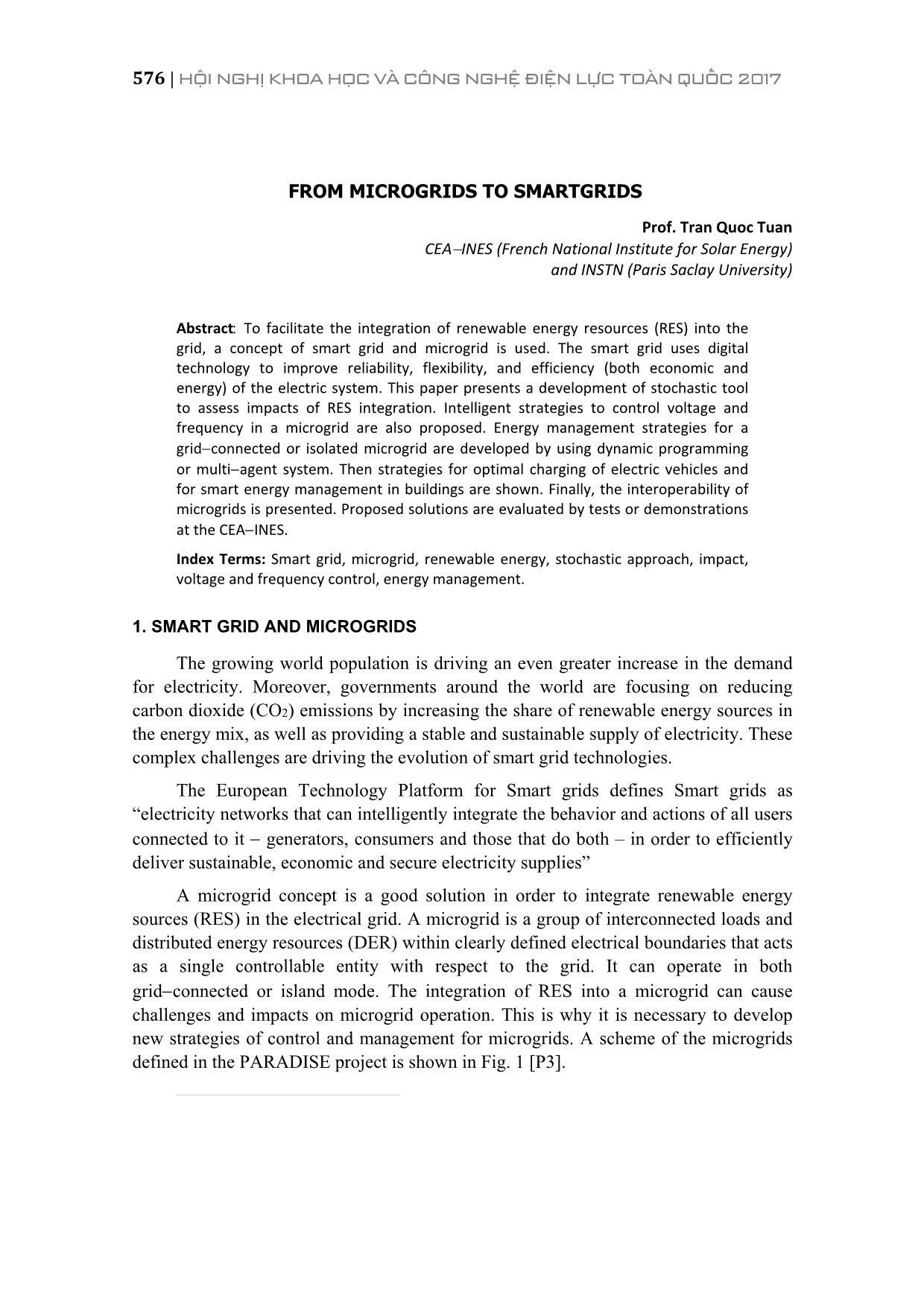
Trang 1
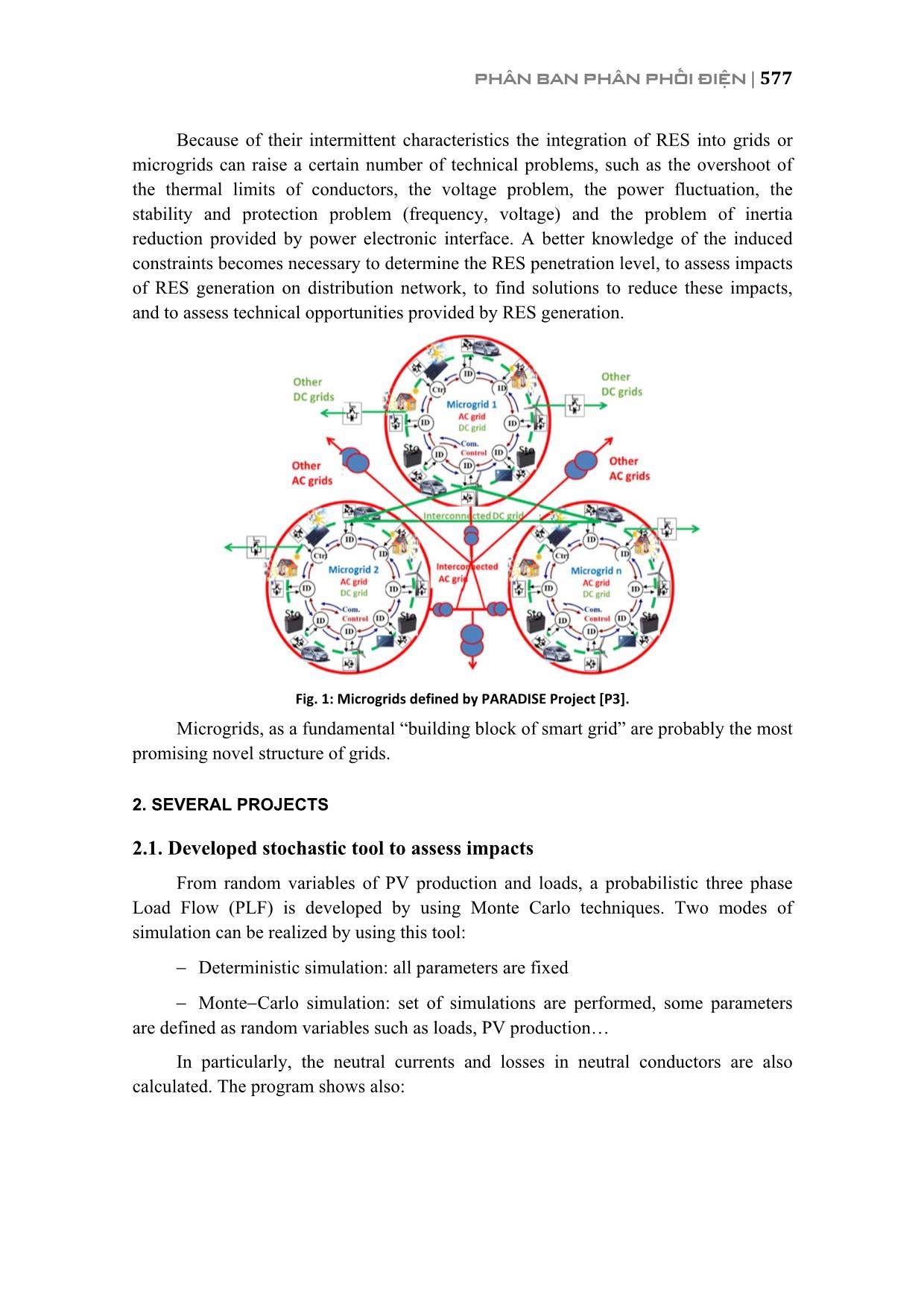
Trang 2
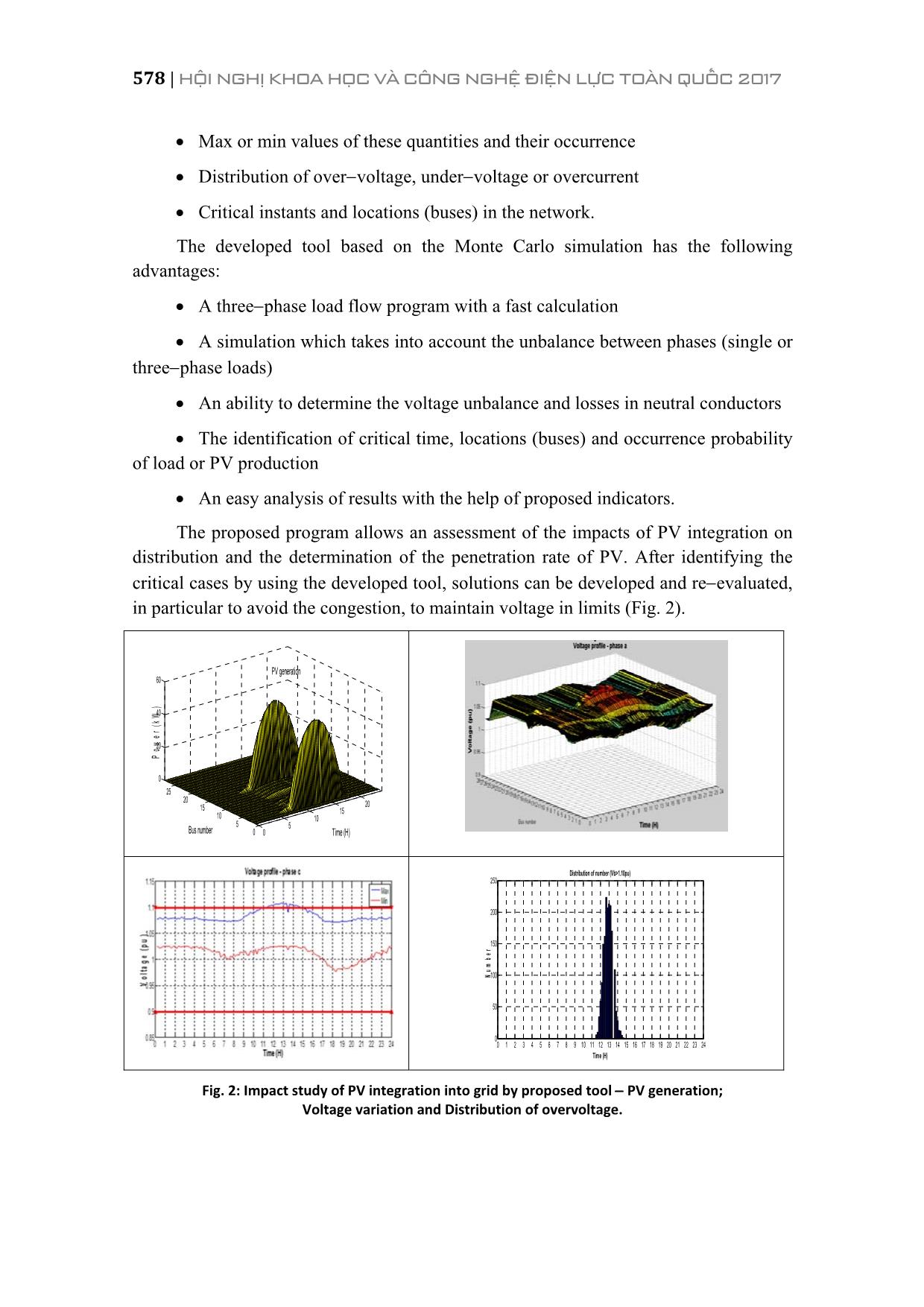
Trang 3
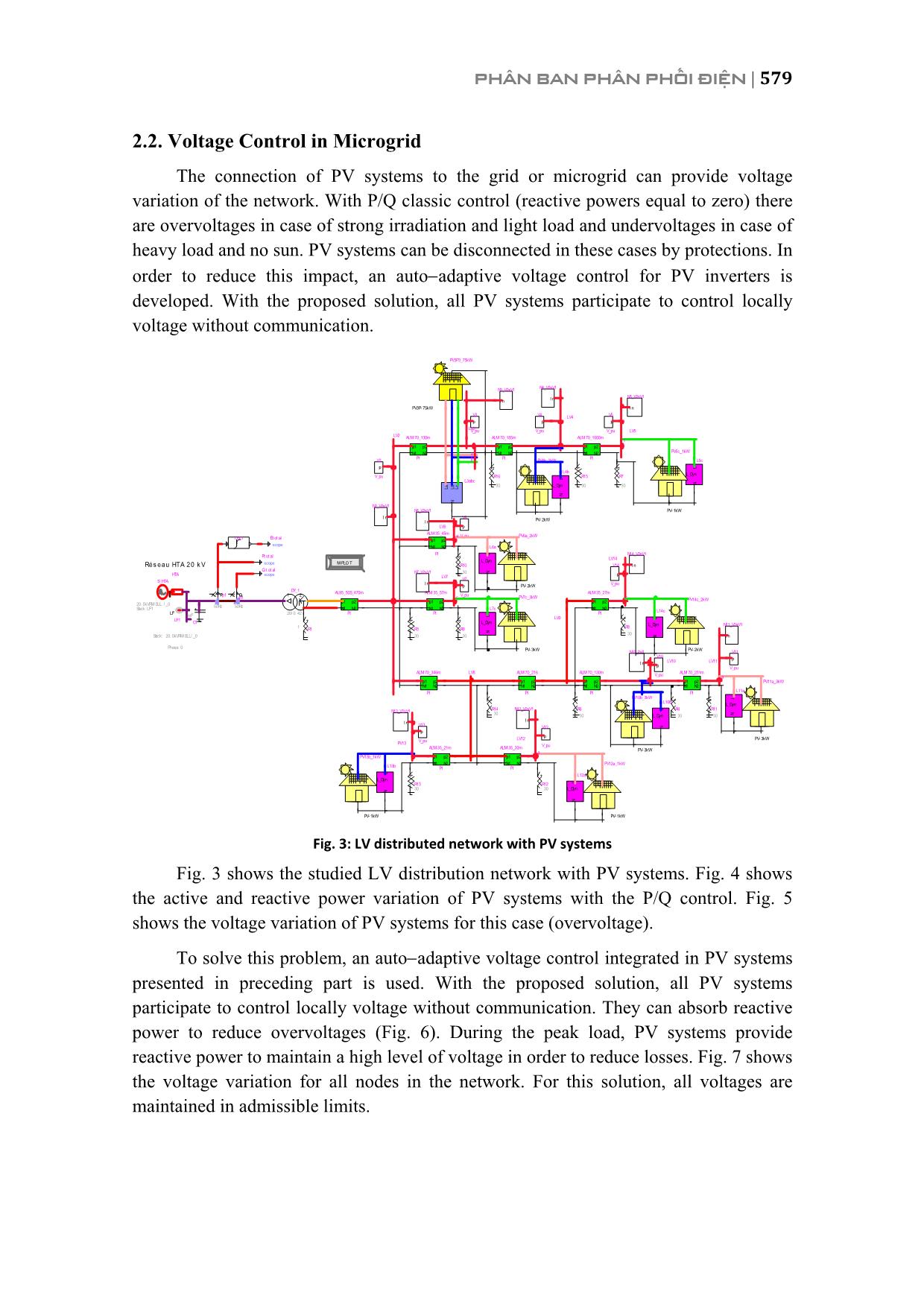
Trang 4
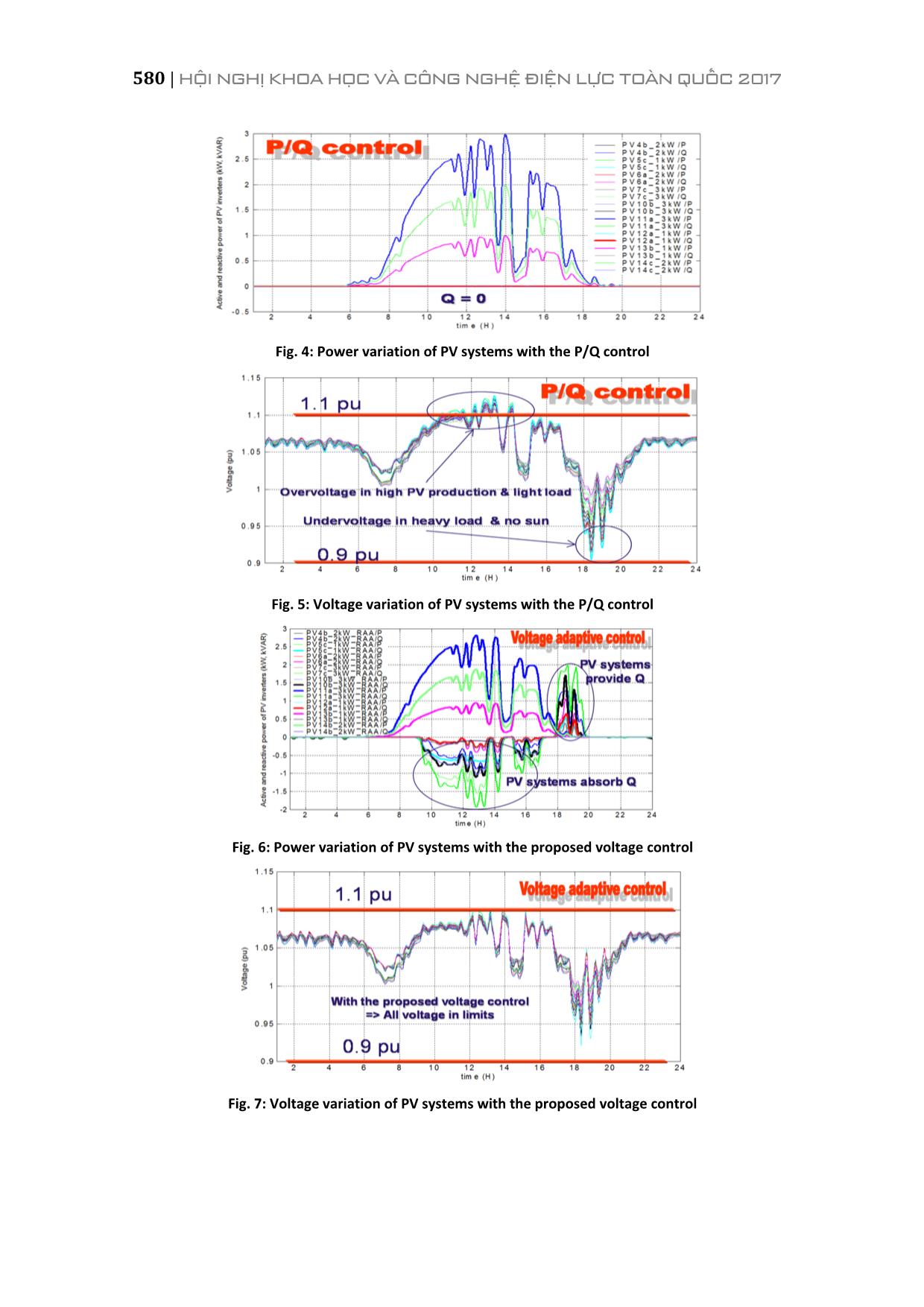
Trang 5
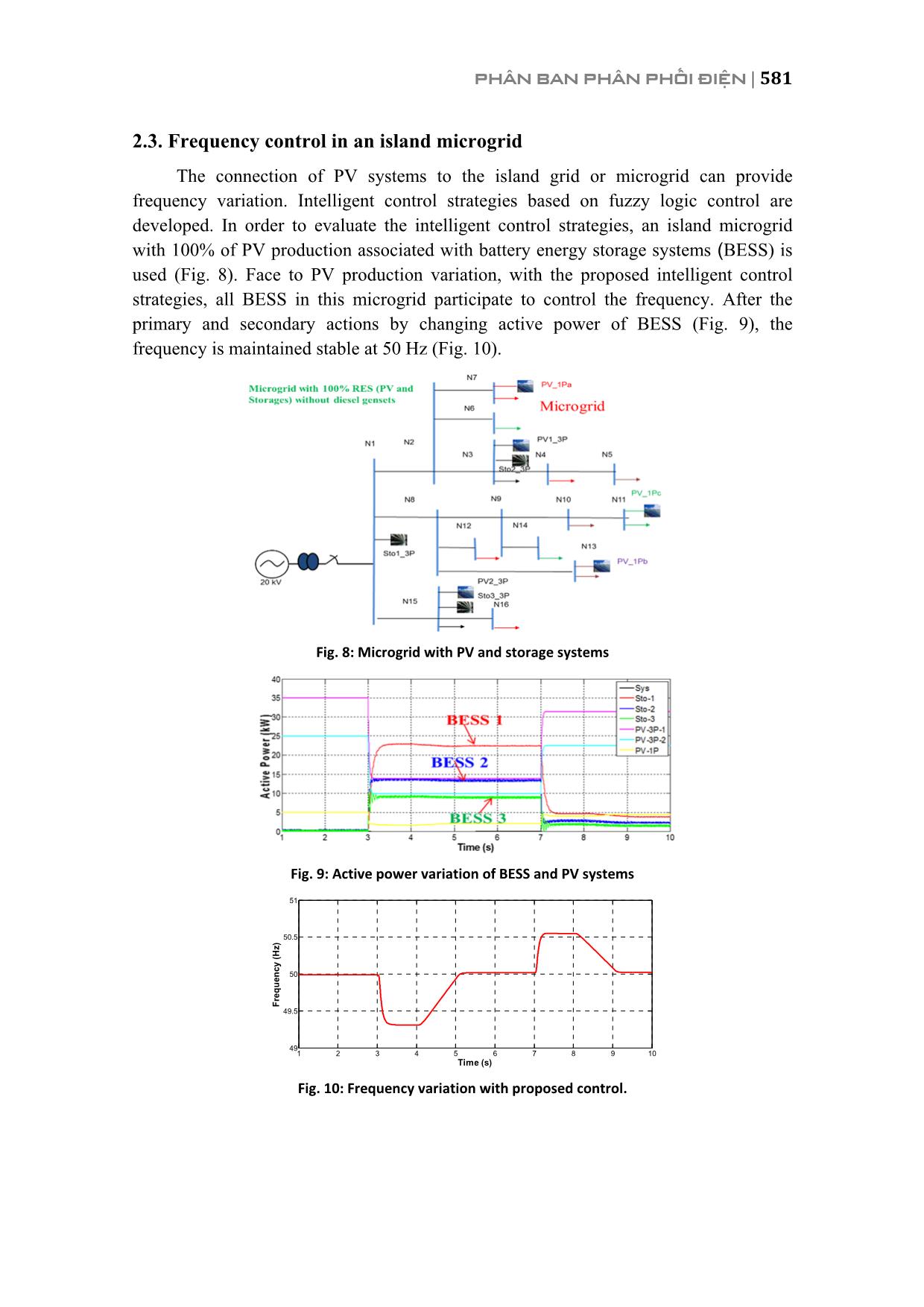
Trang 6
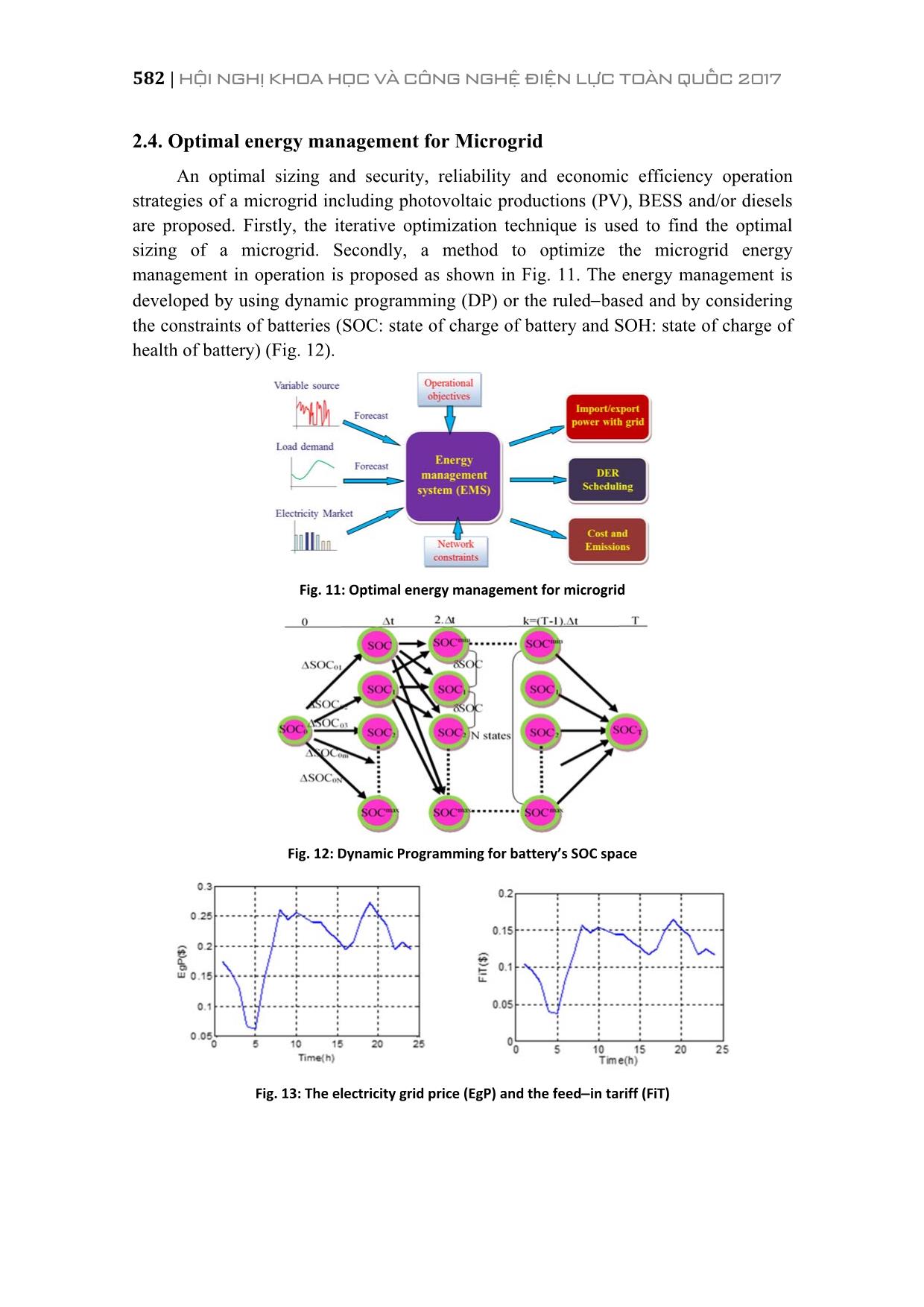
Trang 7
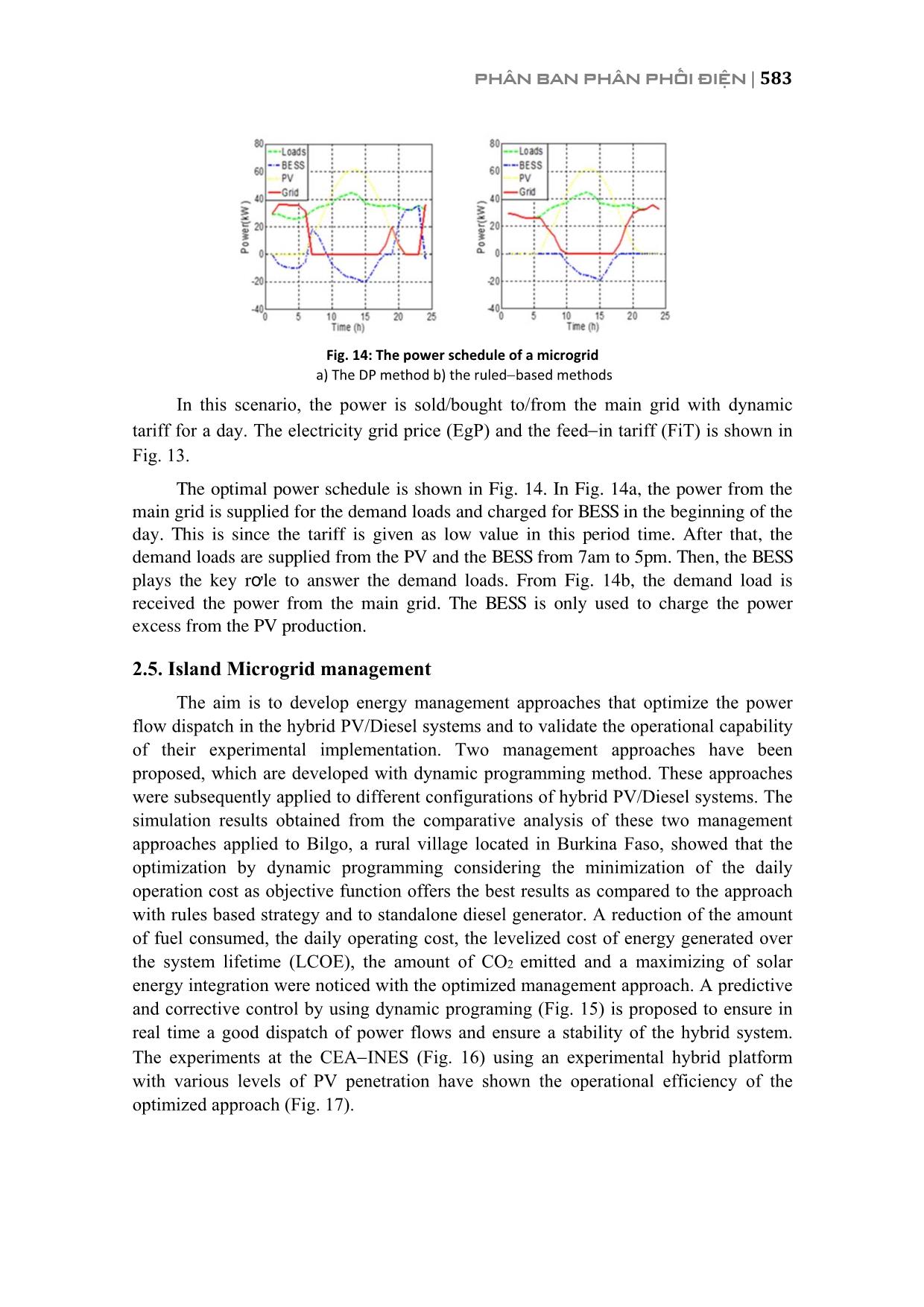
Trang 8
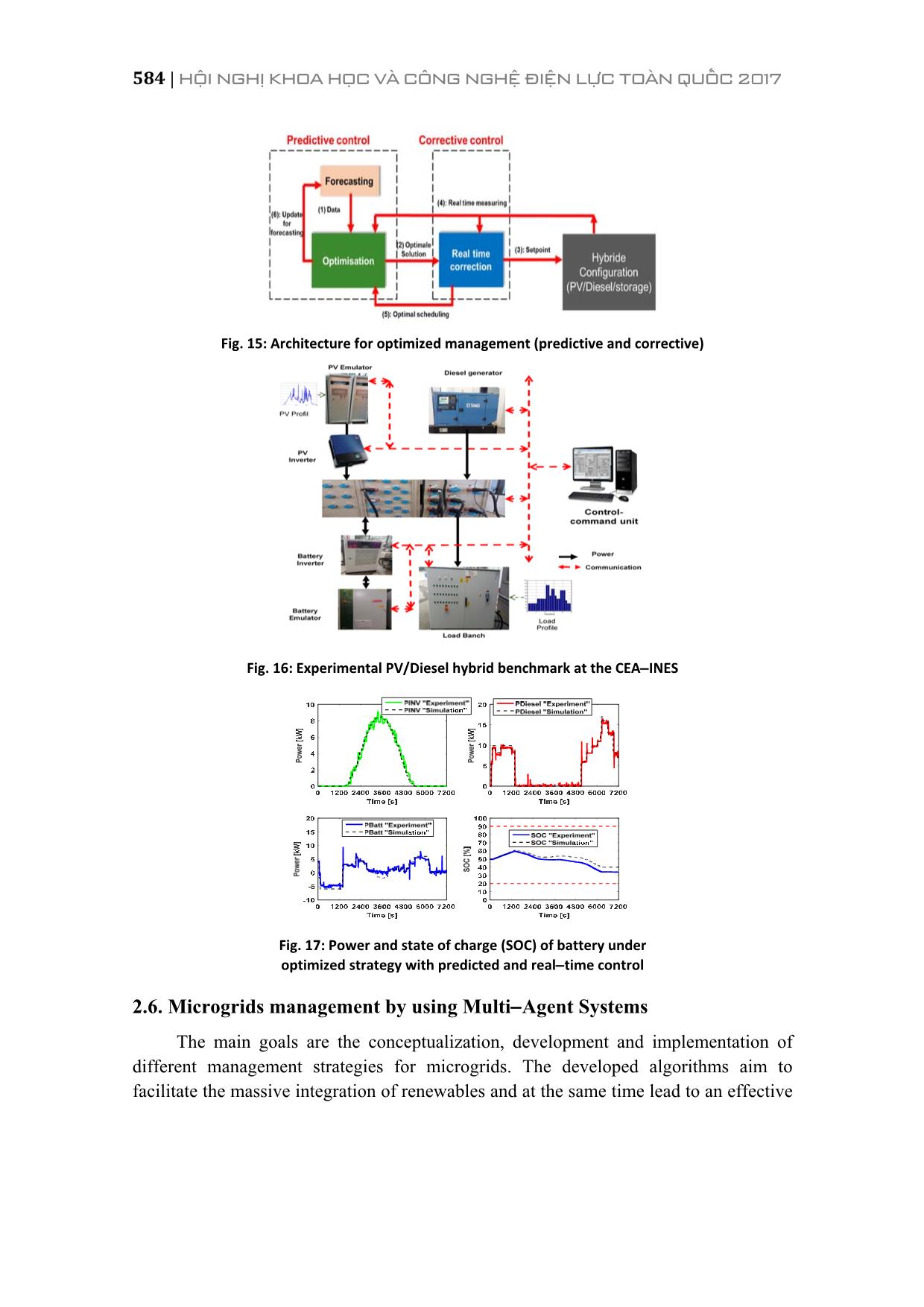
Trang 9
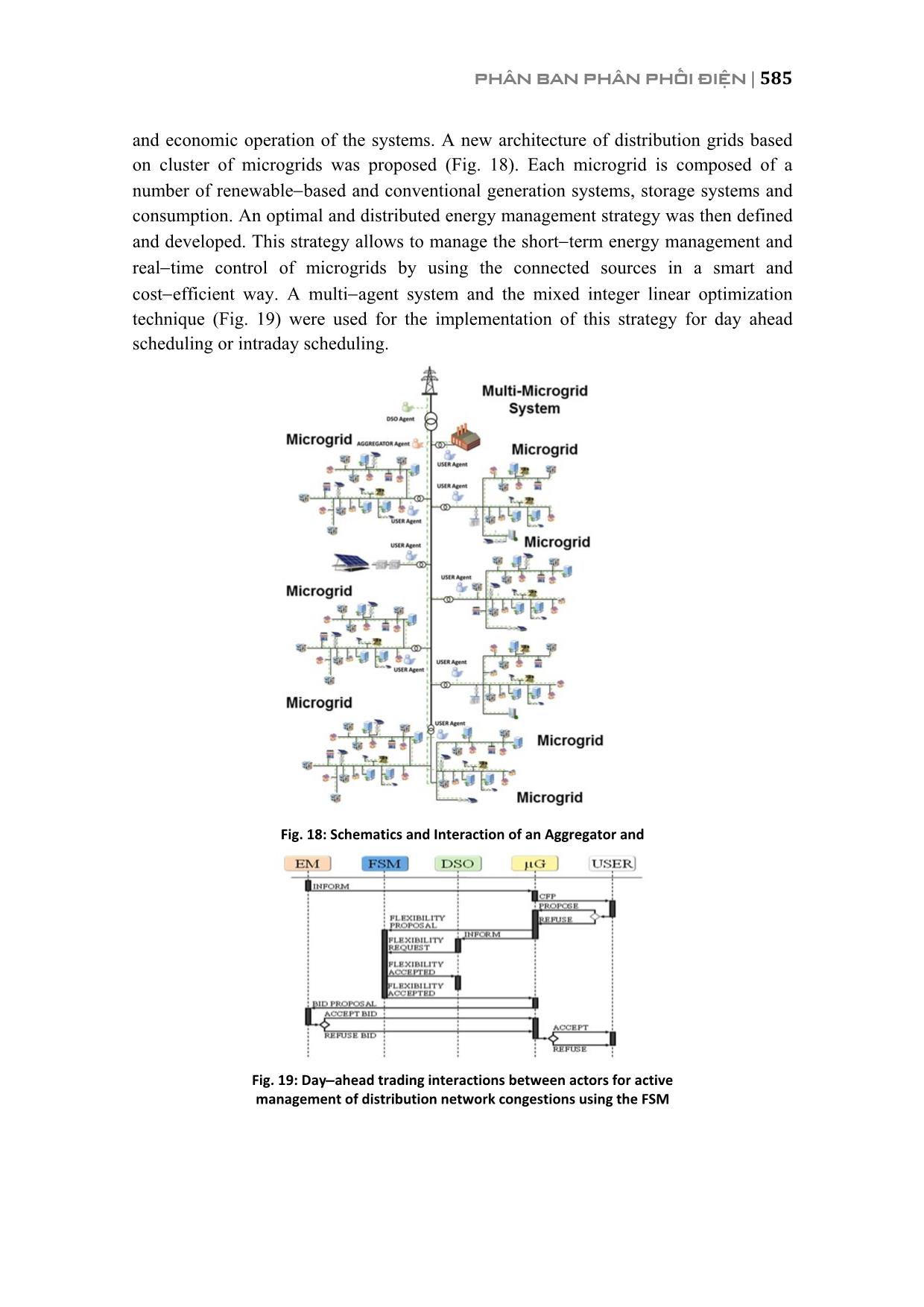
Trang 10
Tải về để xem bản đầy đủ
Tóm tắt nội dung tài liệu: From microgrids to smartgrids
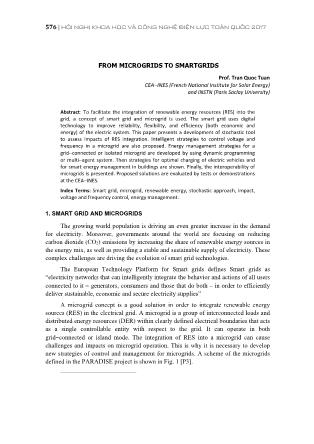
ra day and the real time management of microgrids. Fig. 20a: PV power profiles of forecasted data over 15 minutes and real time measures Fig. 20b: Aggregated consumption power profiles of forecasted data and real time measures Fig. 21: Real power profiles of photovoltaic, battery, consumption and grid exchange in tm without intra day re optimizations PHÂN BAN PHÂN PHỐI ĐIỆN | 587 Fig. 22: Real power profiles of photovoltaic, battery, consumption and grid exchange in tm with intra day re optimizations Fig. 22 illustrate the actual results of the test, which clearly show a more performant control thanks to the combination of re optimization algorithm and the ESS in comparison in case without intra day re optimization (Fig. 21). The use of re optimization allows to reduce the battery’s solicitations and to restore the pack’s SOC in order to reach a final value of 50%. 2.7. Optimal Charging Scheduling of EV in Microgrid Recently, there has been a rapid growth of electric vehicles (EV) connected to the grid. Electric vehicles (EVs) play a key rơle in the transition towards a cleaner energy future. The intersection of energy and automotive sectors and the Smart Grid potential given by electric mobility is followed with great interest. In France in 2020, this enthusiasm for electric vehicles will result in the consumption of 4 to 5 TWh for 2 million electric vehicles. Fig. 23 presents a microgrid includes Electric Vehicles (EV) and PV system. The connection of a large number of electric vehicles to the grid can raise several technical problems or can have significant impacts on power systems. G ri d P g r id P P V P E V s Fig. 23: Microgrid with Electrical Vehicle and PV system In this part, the management system determines the charging planning of EV in order to minimize the total peak consumption. 588 | HỘI NGHỊ KHOA HỌC VÀ CÔNG NGHỆ ĐIỆN LỰC TOÀN QUỐC 2017 Finally, the objective function is to minimize the total peak consumption of the charging station: min ቄmaxt∈D ሼ ܲݐ ሽቅ = min ቐmaxt∈D ቐ PEVi Xit NEV i=1 ቑቑ The simulations are carried out with charging parking in an industrial grid with 100 electric vehicles with normal charge (3 kW). The capacity of each EV is 25 kWh. EV charging can be switched on or switched off each 30 minutes (or 10 min). The charging start time is varied randomly with a normal distribution in the parking between 7am and 9am. The charging stop time is randomly varied between 5pm and 7pm with a normal distribution. The initial SOC of EVs is randomly varied between 20 and 80% with a uniform distribution. Fig. 24: EV total power and power exchange with grid without management and with management (without PV production) Fig. 25: Evolution of EVs’ SOC (without PV production) Fig. 26: EV total power and power exch ange with grid without management and with management (with DSO; without PV production) PHÂN BAN PHÂN PHỐI ĐIỆN | 589 Fig. 27: EV total power and power exchange with grid without management and with management (with PV production) In Fig. 24, the simulation results show that without management the total peak consumption can reach 300 kW between 8:30am and 9:00am. With the proposed solution, the total peak consumption can be reduced to 111 kW (black curve). Fig. 25 presents the evolution of EVs’ SOC over time. After charging stop time Tstop of each vehicle, almost all batteries of EV are filled. With the proposed solution according to the requirement of DSO (signal received from DSO) to reduce total charging power to 50 kW between 11:00am to 12:30pm. Before 11:00am and after 12:30pm, the peak consumption is minimized to 129 kW (red curve in Fig. 26). Fig. 27 shows the EV total power and power exchange with grid without management and with management (with 150 kW of PV production). Figs. 28 and 29 present real demonstration with a EV charging station at the CEA Grenoble and CEA INES. Fig. 28: Real demonstration at CEA Grenoble Fig. 29: Real demonstration with a EV charging station at the CEA INES 590 | HỘI NGHỊ KHOA HỌC VÀ CÔNG NGHỆ ĐIỆN LỰC TOÀN QUỐC 2017 2.8. Energy Management Systems in Buildings Actually, it has been observed that the building sector contributes considerably to final energy demand. Therefore, many studies have been realized in this area. Our work focuses on the smart control of energy management systems in buildings (Fig. 30). This paper proposes a structure of the control system in buildings, in particular for a heating control. This application uses a new real time control method that allows to reduce peak consumption while maintaining thermal comfort. The proposed control method is based on wireless sensor network (WSN) technology which offers continuous measurement, and an interoperable communication network. This method is validated by simulations and real tests. The results demonstrate the performance of the proposed solution and its capability to control heating load. This method can be adapted to any problems by considering dynamic pricing, signals from energy provider and distribution system operator (DSO). The proposition relies on a wireless sensor network (WSN) which allows simultaneous, real time measurement of indoor temperature and power consumption (Fig. 31). The ZigBee communication protocol is used between wireless temperature sensors and equipment. Fig.30: Energy management system Fig.31: Architecture ofthe proposed system for heatingcontrol PHÂN BAN PHÂN PHỐI ĐIỆN | 591 This system comprises an array of wireless temperature sensors, a wireless electrical power sensor, radiators equipped with adaptive controls, and a central control unit. The proposed solution is tested for a real apartment to control radiators (Fig. 32). Fig. 32: Plan of the test apartment Fig. 33: Power required with the traditional heating regulator (R0) Fig. 34: Interior temperature of the three rooms with the traditional heating regulator (R0) To illustrate the advantages of the proposed method, we applied a 2700 W limit for authorized power. Thermal comfort is always maintained, but T_max is adjusted according to the instantaneous power consumption. Fig. 35: Power required with the proposed method (R1) Power required(W) 0 500 1000 1500 2000 2500 3000 3500 4000 14:24:00 16:48:00 19:12:00 21:36:00 00:00:00 Temperature 19 19,5 20 20,5 21 21,5 22 22,5 23 14:24:00 16:48:00 19:12:00 21:36:00 00:00:00 Time °C T1 T2 T3 Authorizedpowe Power required(W) 0 500 1000 1500 2000 2500 3000 3500 4000 14:24:00 16:48:00 19:12:00 21:36:00 00:00:00 Time W 592 | HỘI NGHỊ KHOA HỌC VÀ CÔNG NGHỆ ĐIỆN LỰC TOÀN QUỐC 2017 Fig. 36: Interior temperature of the three rooms with the proposed method These results indicate that: The proposed heating control allows a reduction in total power demand from 3770 W to 2700 W. Thermal comfort is maintained: the interiortemperature of the rooms remains between 22 °C and 20 °C. With the proposed method, Fig. 13 shows that maximal temperature is variable, and minimal temperature is always maintained at 20 °C. The reduction of contract power therefore results in a lower cost of subscription. Temperature was regulated for the two cases over a 24 hour period (from 0h to 24h). The table below shows that the differences in energy consumption between the two cases are negligible. Tmin (°C) Tmax(°C) Consumption (kWh) Pmax (W) Traditional regulation 20 22 22.843 2700 Proposed method 20 22 24.84 3700 2.9. Interoperability of microgrid platforms The interoperability of micro grid platforms, particularly in information and communication layers has been developed. The implementation of Common Information Model (CIM) semantic over OPC Unified Architecture (OPC UA) protocol, particularly the mapping of CIM semantic to OPC UA address space, is also considered, to ensure that the exchanged data is mutually and correctly understood by all the partners of the network. Some insights about requirements to deliver OPC based application via WAN connection are provided. This combination brings CIM semantic to the OPC UA communication and allows the provision of OPC based applications via WAN connection. This contribution enables a seamless and meaningful communication among partners of the collaboration network and provides a strong support for Variable setpoint Temperature 19 19,5 20 20,5 21 21,5 22 22,5 23 14:24:00 16:48:00 19:12:00 21:36:00 00:00:00 Time °C T1 T2 T3 PHÂN BAN PHÂN PHỐI ĐIỆN | 593 interoperability of micro grid platforms between PRISME (CEA INES) and PREDIS (G2elab) (Figs. 37 and 38). Fig. 37: Interoperability of micro grid platforms between PRISME (CEA INES) and PREDIS (G2elab). Fig. 38: SCADA and cloud architecture proposed for interoperability between two platforms – PRISME (CEA INES) and PREDIS (G2elab) 3. DEMONSTRATIONS In order to analyze impacts of RES integration and evaluate proposed solutions for microgrid, a real time simulator and real demonstration are used at the CEA INES (Figs. 39 and 40). 594 | HỘI NGHỊ KHOA HỌC VÀ CÔNG NGHỆ ĐIỆN LỰC TOÀN QUỐC 2017 Fig. 39: Microgrid with real time simulator at the CEA INES Fig. 40: Microgrid demonstration at CEA INES 4. CONCLUSIONS Smartgrids and microgrids are one of the most important activities at the CEA/INES, and the lab of intelligent electricity systems (LSEI). The LSEI develops management and control strategies of any complex electricity system for efficient smart grid integration or stand alone operation. The developed algorithms are on one side predictive algorithms which use production and consumption forecasting for energy management, and on the other side real time system control algorithms. Proposed solutions are evaluated by the demonstration at the CEA INES. REFERENCES [1] Gabin Koucoi, Quoc Tuan Tran, H. Buttin, “Energy Management Strategies for Hybrid PV/Diesel Energy Systems: Simulation and Experimental Validation”, International Journal of Energy and Power Engineering. Vol. 5, No. 1, 2016. [2] V.H. Nguyen, T. Tran Quoc, Y. Besanger, “SCADA as a service approach for interoperability of micro grid platforms”, in Elsevier Sustainable Energy, Grids and Networks (2016). [3] Quoc Tuan TRAN, Van Linh NGUYEN, “Integration of Electric Vehicles into Industrial Network: Impact Assessment and Solutions”, IEEE/PES, General Meeting, Boston, Massachusetts, USA 17 21 July 2016. PHÂN BAN PHÂN PHỐI ĐIỆN | 595 [4] E. Krüger, E. Amicarelli and Q. T. Tran, "Impact of european market frameworks on integration of photovoltaics in virtual power plants," IEEE EEEIC, 16 IEEE International Conference on Environment and Electrical Engineering, Florence, ITALY, 7 10 June 2016. [5] E. Amicarelli, T. Tran Quoc, S. Bacha, “Multi Agent System for Day Ahead Energy Management of Microgrid”, European Conference on Power Electronics and Applications (EPE ECCE Europe), 5–9 September 2016, Karlsruhe, Germany. [6] T. Tran Quoc, “Optimal energy management and control for an isolated area”; CIGRE, International Symposium DEVELOPMENT OF ELECTRICITY INFRASTRUCTURES IN SUB SAHARAN AFRICA, Cape Town–South Africa –26 30 October 2015. [7] N.A Luu, T. Tran Quoc, “Optimal energy management for grid connected microgrid by using Dynamic programming method”, IEEE/PES, General Meeting, Denver, Colorado, USA 27 30 July 2015. Ph.D. thesis T1. Guillaume RAMI, "Adaptive voltage control for distributed generations connected to distribution networks", PhD thesis prepared at the Grenoble INP, Defended on 9 November 2006. T2. Thanh Luong LE “Detection of instability in grid with high RES penetration”, PhD thesis prepared at the Grenoble INP, Defended on 22 January 2008. T3. Thi Minh Chau LE, "Coupling Photovoltaic inverters to the network, aspects of control and support capacity for disturbances", PhD thesis prepared at the Grenoble INP, Defended on 25 January 2012. T4. Van Linh NGUYEN, "Coupling photovoltaic systems and electric vehicles to the grid: Problems and solutions"; PhD Thesis prepared at the Grenoble INP and CEA INES, Defended on 1st October 2014. T5. Cedric ABBEZZOT, "Flywheel energy storage system coupled to the photovoltaic generator and controlled by a real time simulator"; PhD Thesis prepared at the CEA INES, Defended on 15 December 2014. T6. Ngoc An LUU, "Strategies of control and management for microgrids", PhD Thesis prepared at the CEA INES and G INP, Defended on 18 December 2014 T7. Eiko KRUGER, "Development of algorithms for optimal management of energy storage systems based on adaptive models", PhD Thesis prepared at the CEA INES, Defended on 21 November 2016. T8. GABIN A. KOUCOI, "Energy Management in PV/Diesel hybrid system for isolated and rural zones: optimization and experimentation”, PhD Thesis prepared at the CEA INES and University of Burkina Faso, Defended on 28 February 2017. T9. Elvira AMICARELLI, "Management strategy for power grids with a high rate of distributed renewable production", PhD Thesis prepared at the CEA INES, Defended on 16 October 2017. 596 | HỘI NGHỊ KHOA HỌC VÀ CÔNG NGHỆ ĐIỆN LỰC TOÀN QUỐC 2017 T10. Hélène CLEMOT, "Strategies for optimal management of marine wave resources ", PhD Thesis prepared at the CEA INES and ECN. T11. Tung Lam NGUYEN, " Smart control strategies in Microgrids with Multi Agent Systems", PhD Thesis prepared at the CEA INES and G INP. T12. Karla SOUSA, "Stability in large network with high RES penetration”. T13. Laurène PARENT, "Primary and secondary reserve in large network with high RES penetration". T14. Anthony ROY, "Management of an island grid in SEA". T15. Tran The HOANG, "Smart protection strategies in Microgrid". T16. Tai LE, "New Architecture of PV power plants ". Several Projects [P1] GREENLYS: Smart grid demonstration of the management of multiple electric vehicle charging stations. [P2] REFLEXE: Smart grid demonstration at the regional size (south east of France). [P3] PARADISE (ANR PROGELEC Project ID: ANR‐13‐PRGE‐0007): high penetration of renewable energy resources into grid with DS. [P4] ERIGRID (H2020, project ID: 654113) European Research Infrastructure supporting Smart Grid Systems Technology Development, Validation and Roll Out. [P5] SEAS (Call 7 ITEA2, No. 1204) Smart Energy Aware Systems. [P6] UNITED GRID (H2020, Project ID: 314175): Autonomous Management System Developed for Building and District Levels. [P7] M2M GRID (ERA‐NET, Project ID: 82136): From micro to Mega GRID: Integration approach for the new generation of smart grids. [P8] PPInterop (Carnot project): Interoperability between two platforms: PRISME PREDIS. [P9] FENIX (European project) Virtual power plants.
File đính kèm:
from_microgrids_to_smartgrids.pdf