EEG – based study on sleep quality improvement by using music
Napping is essential for human to reduce drowsiness, contribute to improving cognitive function,
reflex, short-term memory, and state. Some studies have shown that a certain amount of time
for a nap can boost the body's immunity and reduce the danger of cardiovascular disease. Using
music for relaxation and enjoyment to fall asleep is an effective solution that earlier studies have
shown. There are many genres of music that have been used for stimulation, such as binaural
beats or melodic sounds. The aim of the study was to confirm the positive effect of music on
sleep quality by analyzing electroencephalography signal. There were four types of music is being
used in this study: instrumental music, Ballad music, K-pop music, and Jazz. The study applied
the pre-processing include filtering block, features extraction, and clustering steps to analyze raw
data. This research calculated the power spectrum of Alpha wave and Theta wave, to detect the
transition of wake - sleep stages by K-means clustering algorithm. Sleep latency is one of the factors
that determine the quality of sleep. The sleep onset is detected based on the phase shift of the
Alpha and Theta waves. The exact timing of the sleep onset was important in this study. The user
interface was developed in this study to compute sleep latency in normal and musical experiment.
As a result, music is an intervention in helping people fall asleep easier (mean of sleep latency in
normal and musical experiment was 9.0714 min and 5.6423 min, respectively) but the standard
deviation of this result was rather high due to the little number of experiments. However, the study
concludes that listening to music before naptime can improve sleep latency in some participants.
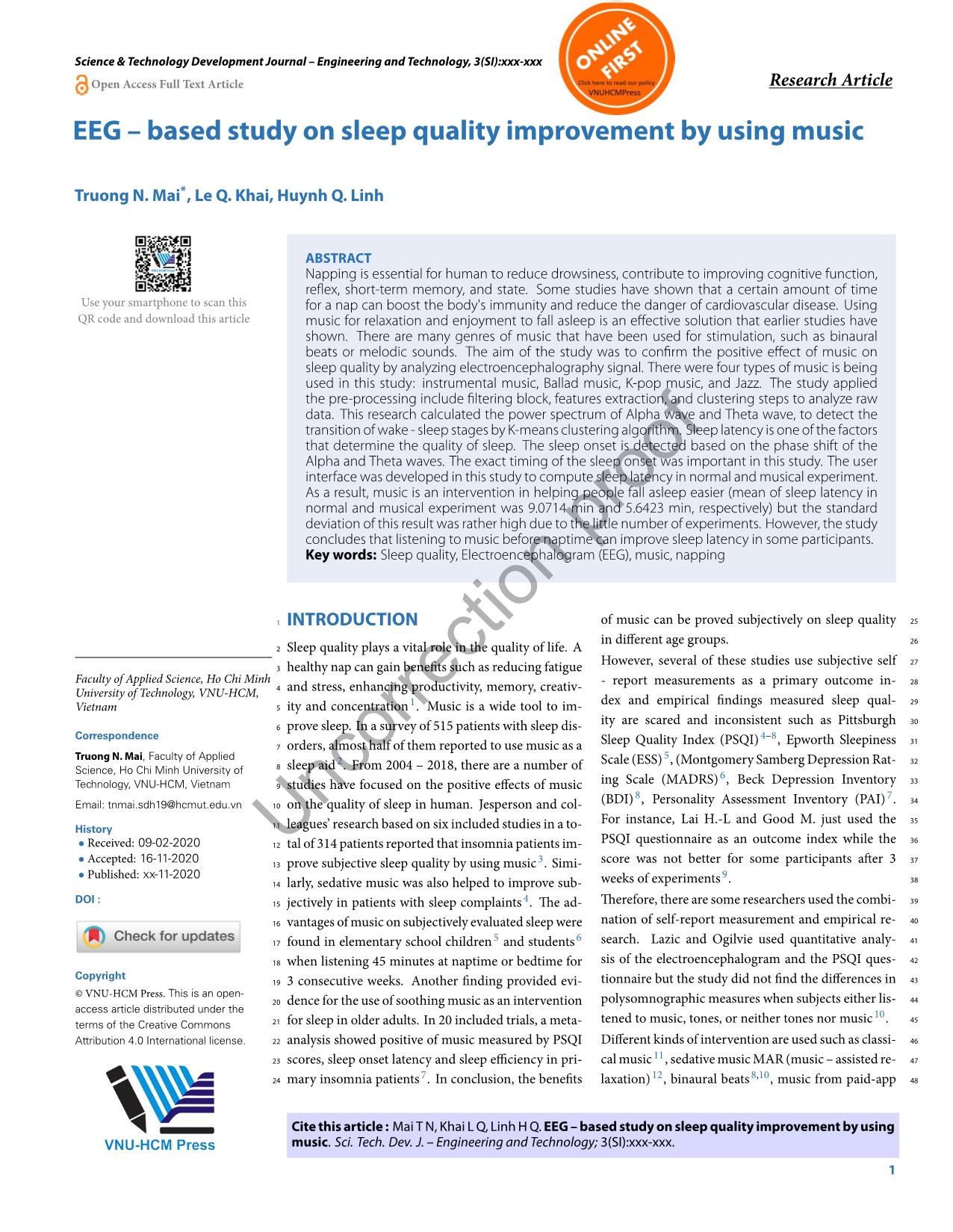
Trang 1
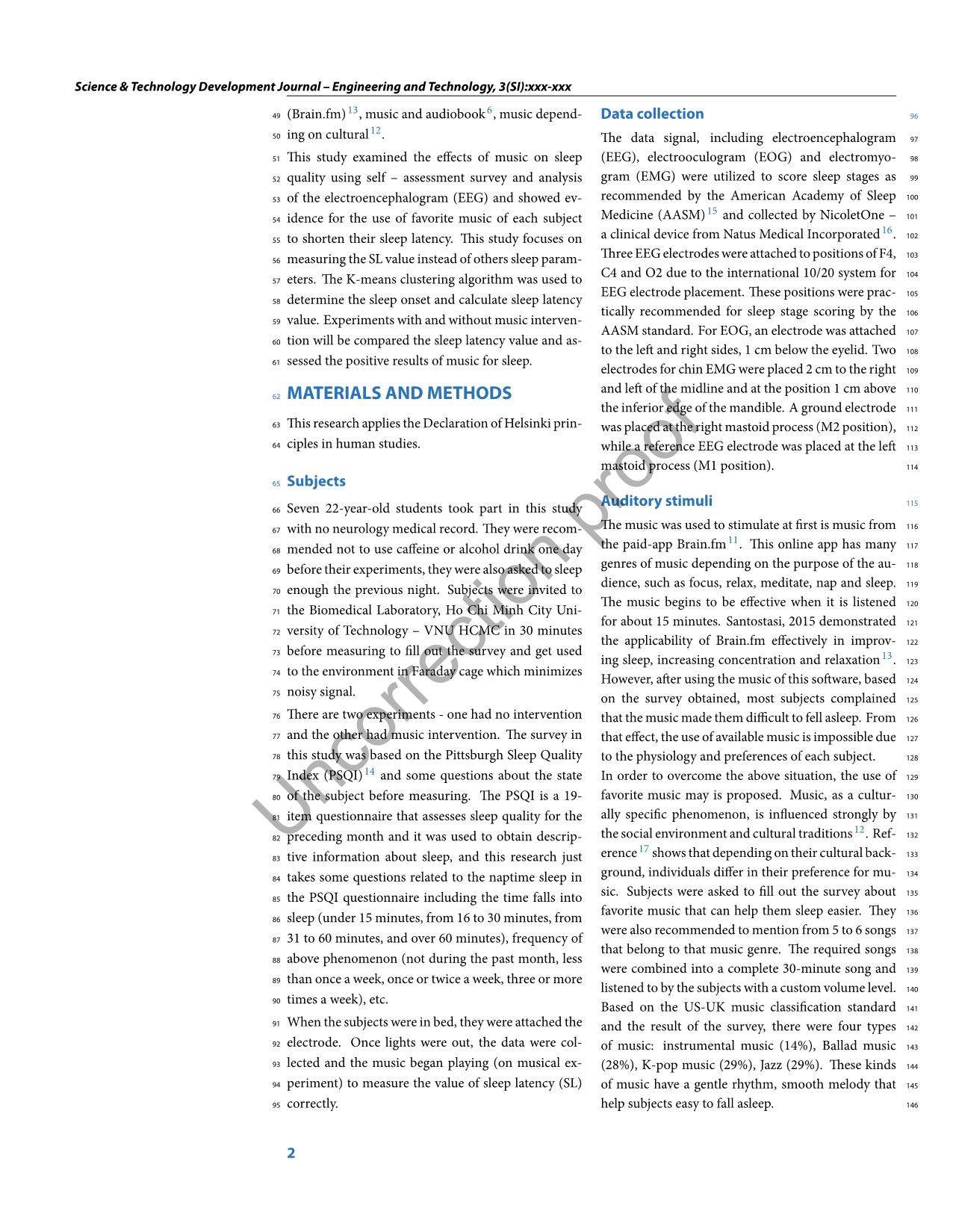
Trang 2
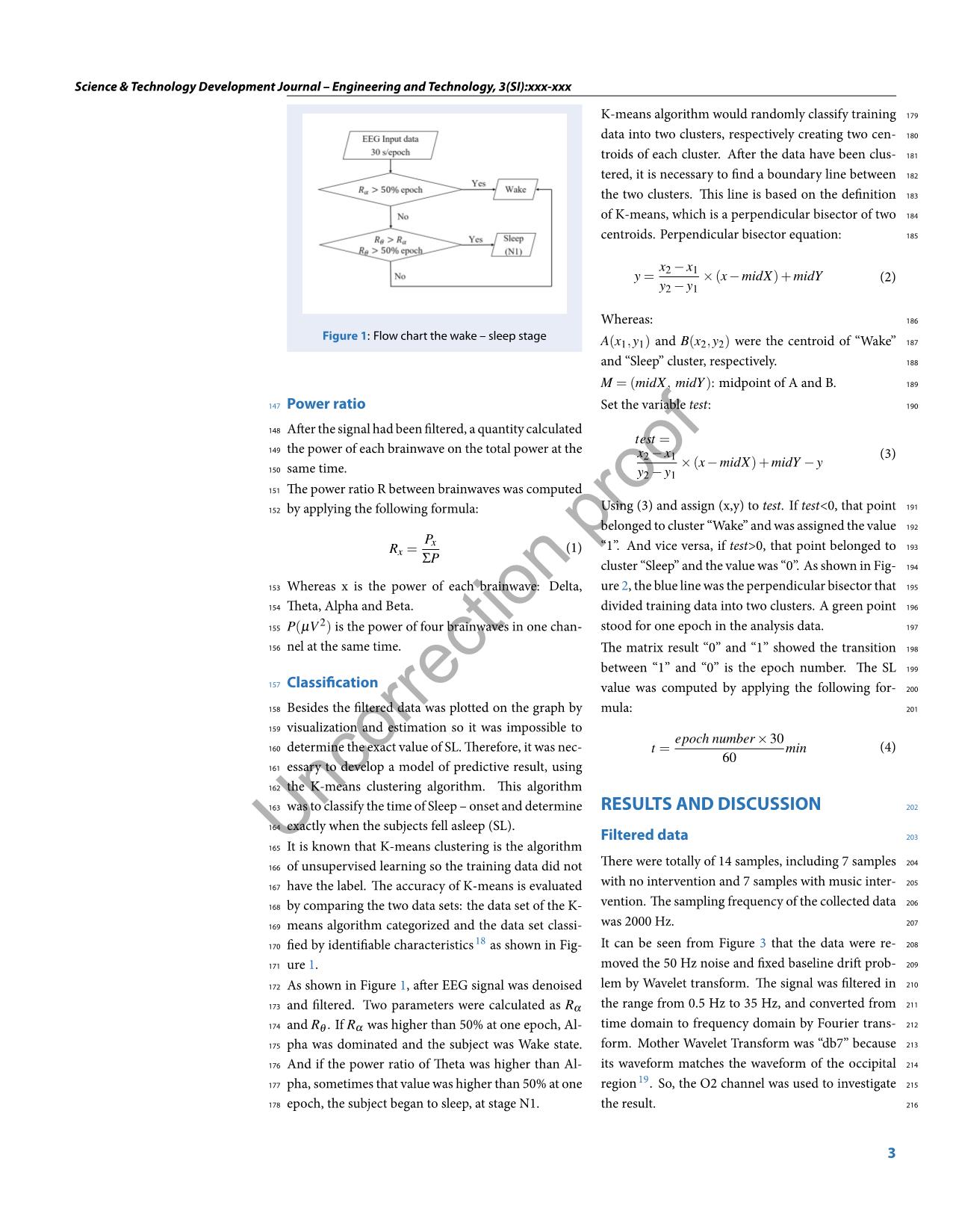
Trang 3
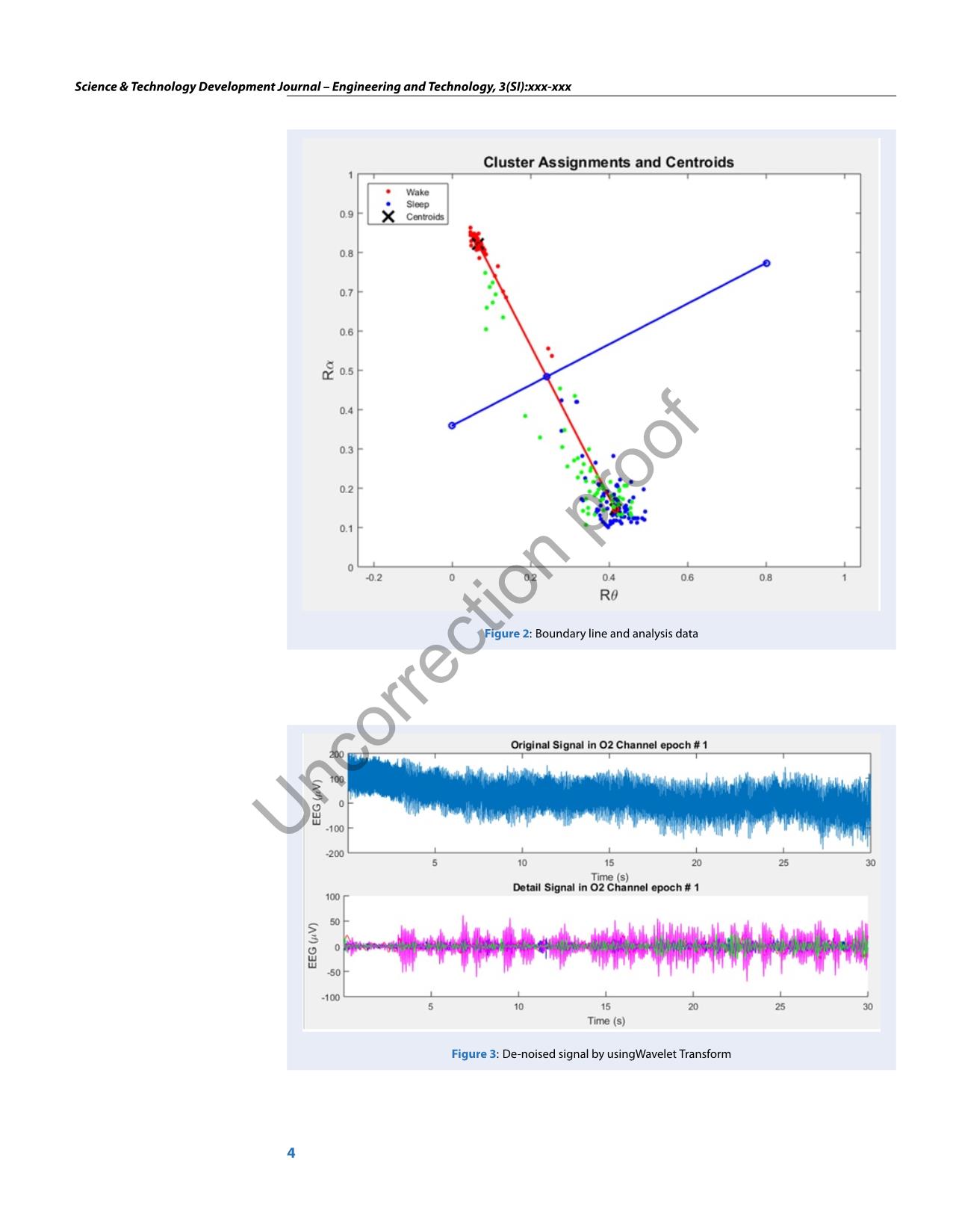
Trang 4
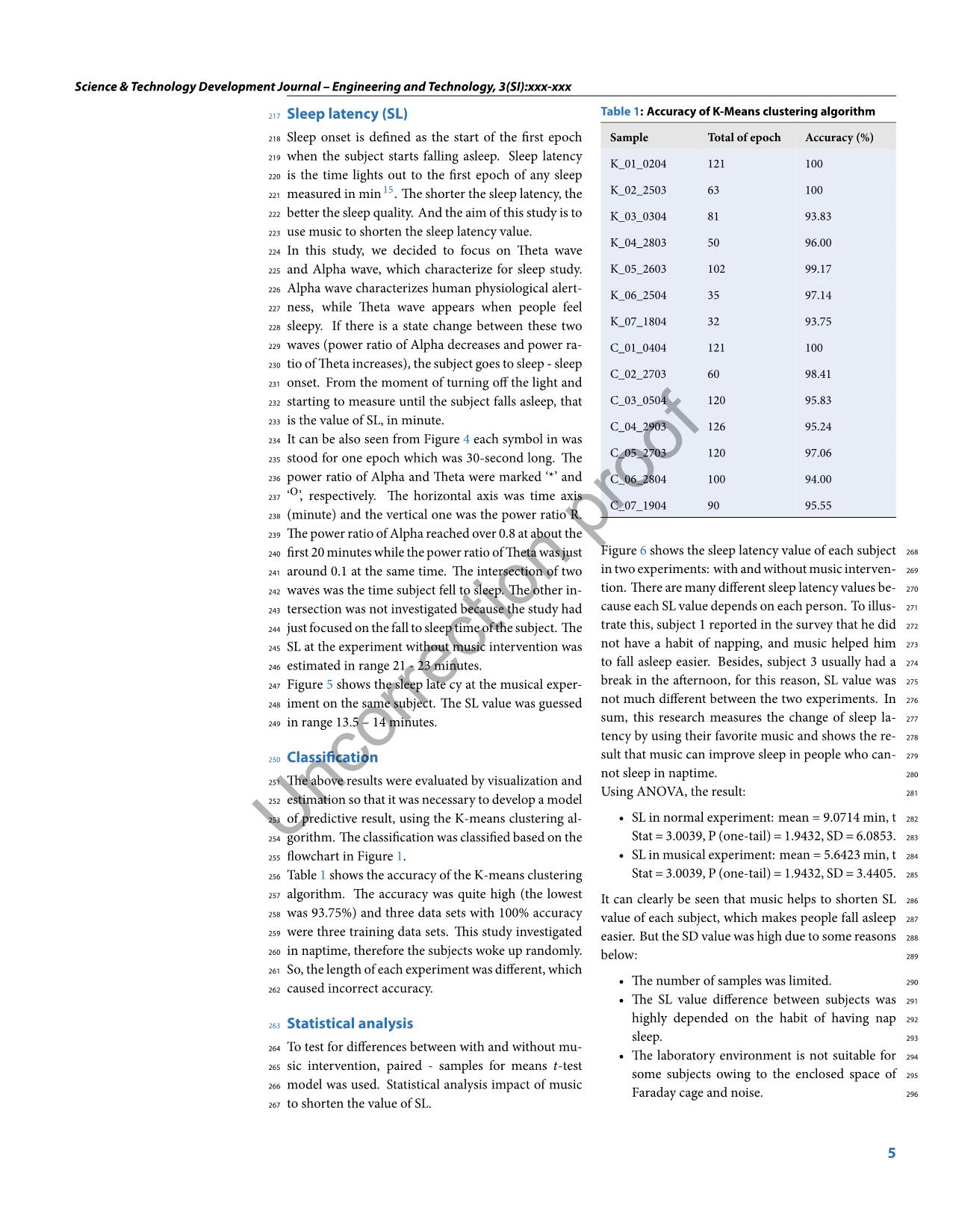
Trang 5
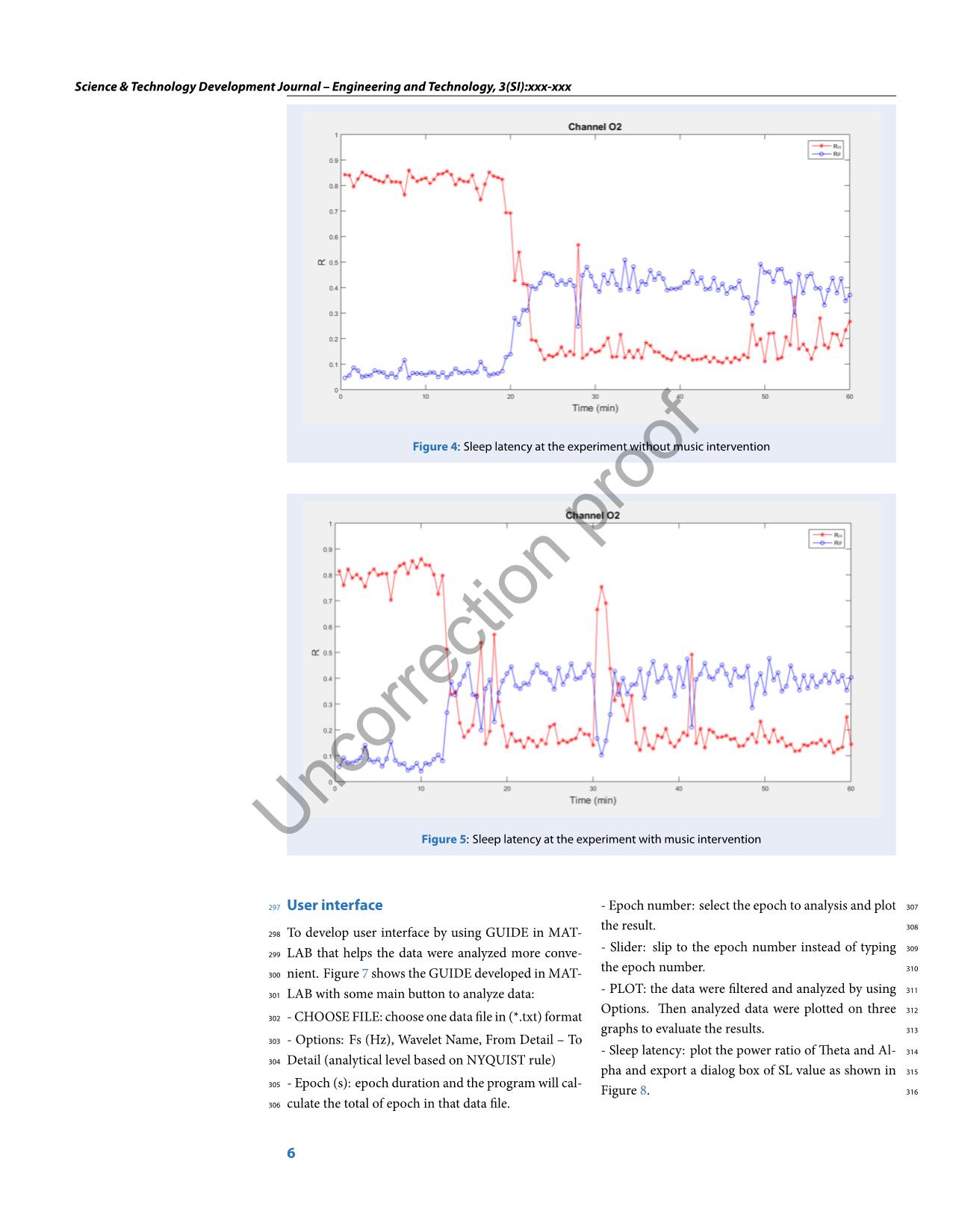
Trang 6
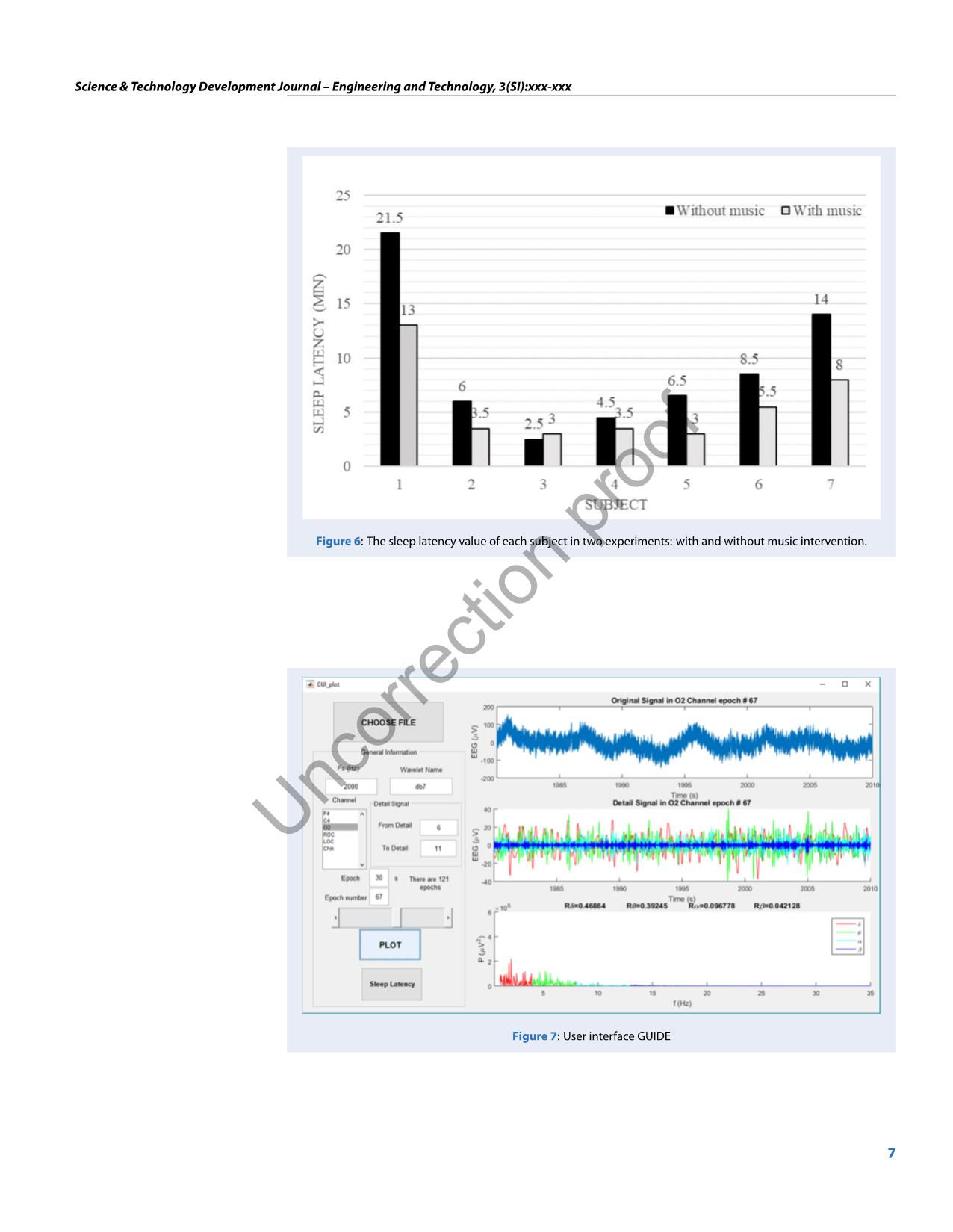
Trang 7
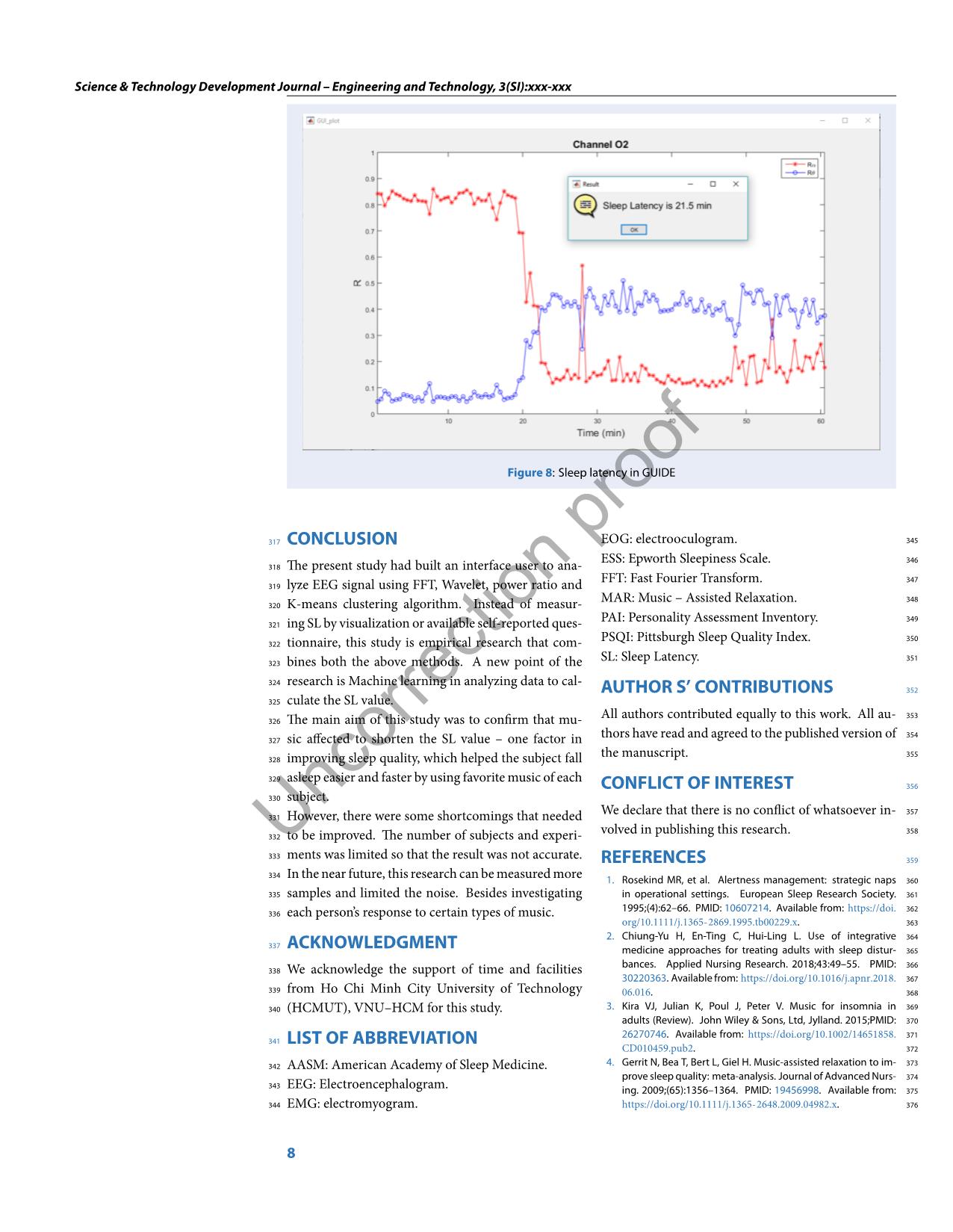
Trang 8
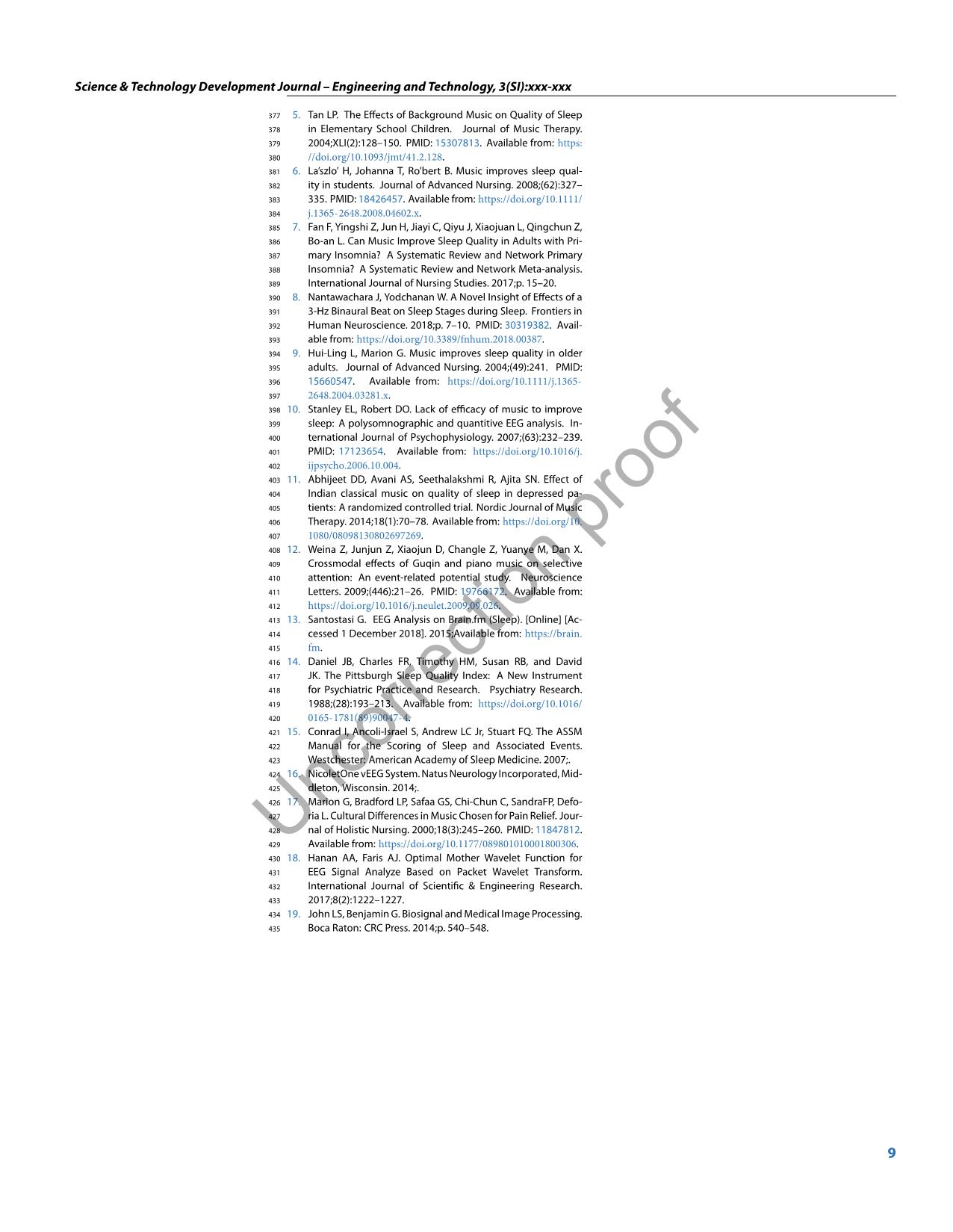
Trang 9
Tóm tắt nội dung tài liệu: EEG – based study on sleep quality improvement by using music
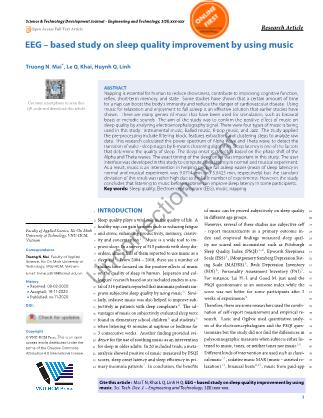
Al-176 pha, sometimes that value was higher than 50% at one177 epoch, the subject began to sleep, at stage N1.178 K-means algorithm would randomly classify training 179 data into two clusters, respectively creating two cen- 180 troids of each cluster. After the data have been clus- 181 tered, it is necessary to find a boundary line between 182 the two clusters. This line is based on the definition 183 of K-means, which is a perpendicular bisector of two 184 centroids. Perpendicular bisector equation: 185 y= x2 x1 y2 y1 (x midX)+midY (2) Whereas: 186 A(x1;y1) and B(x2;y2) were the centroid of “Wake” 187 and “Sleep” cluster, respectively. 188 M = (midX ; midY ): midpoint of A and B. 189 Set the variable test: 190 test = x2 x1 y2 y1 (x midX)+midY y (3) Using (3) and assign (x,y) to test. If test<0, that point 191 belonged to cluster “Wake” andwas assigned the value 192 “1”. And vice versa, if test>0, that point belonged to 193 cluster “Sleep” and the value was “0”. As shown in Fig- 194 ure 2, the blue line was the perpendicular bisector that 195 divided training data into two clusters. A green point 196 stood for one epoch in the analysis data. 197 The matrix result “0” and “1” showed the transition 198 between “1” and “0” is the epoch number. The SL 199 value was computed by applying the following for- 200 mula: 201 t = epoch number30 60 min (4) RESULTS ANDDISCUSSION 202 Filtered data 203 There were totally of 14 samples, including 7 samples 204 with no intervention and 7 samples with music inter- 205 vention. The sampling frequency of the collected data 206 was 2000 Hz. 207 It can be seen from Figure 3 that the data were re- 208 moved the 50 Hz noise and fixed baseline drift prob- 209 lem by Wavelet transform. The signal was filtered in 210 the range from 0.5 Hz to 35 Hz, and converted from 211 time domain to frequency domain by Fourier trans- 212 form. Mother Wavelet Transform was “db7” because 213 its waveform matches the waveform of the occipital 214 region19. So, the O2 channel was used to investigate 215 the result. 216 3 Un co rre cti on pr oo f Science & Technology Development Journal – Engineering and Technology, 3(SI):xxx-xxx Figure 2: Boundary line and analysis data Figure 3: De-noised signal by usingWavelet Transform 4 Un co rre cti on pr oo f Science & Technology Development Journal – Engineering and Technology, 3(SI):xxx-xxx Sleep latency (SL)217 Sleep onset is defined as the start of the first epoch218 when the subject starts falling asleep. Sleep latency219 is the time lights out to the first epoch of any sleep220 measured in min15. The shorter the sleep latency, the221 better the sleep quality. And the aim of this study is to222 use music to shorten the sleep latency value.223 In this study, we decided to focus on Theta wave224 and Alpha wave, which characterize for sleep study.225 Alpha wave characterizes human physiological alert-226 ness, while Theta wave appears when people feel227 sleepy. If there is a state change between these two228 waves (power ratio of Alpha decreases and power ra-229 tio ofTheta increases), the subject goes to sleep - sleep230 onset. From the moment of turning off the light and231 starting to measure until the subject falls asleep, that232 is the value of SL, in minute.233 It can be also seen from Figure 4 each symbol in was234 stood for one epoch which was 30-second long. The235 power ratio of Alpha and Theta were marked ‘*’ and236 ‘O’, respectively. The horizontal axis was time axis237 (minute) and the vertical one was the power ratio R.238 Thepower ratio of Alpha reached over 0.8 at about the239 first 20minutes while the power ratio ofThetawas just240 around 0.1 at the same time. The intersection of two241 waves was the time subject fell to sleep. The other in-242 tersection was not investigated because the study had243 just focused on the fall to sleep time of the subject. The244 SL at the experiment without music intervention was245 estimated in range 21 - 23 minutes.246 Figure 5 shows the sleep late cy at the musical exper-247 iment on the same subject. The SL value was guessed248 in range 13.5 – 14 minutes.249 Classification250 The above results were evaluated by visualization and251 estimation so that it was necessary to develop amodel252 of predictive result, using the K-means clustering al-253 gorithm. The classification was classified based on the254 flowchart in Figure 1.255 Table 1 shows the accuracy of the K-means clustering256 algorithm. The accuracy was quite high (the lowest257 was 93.75%) and three data sets with 100% accuracy258 were three training data sets. This study investigated259 in naptime, therefore the subjects woke up randomly.260 So, the length of each experiment was different, which261 caused incorrect accuracy.262 Statistical analysis263 To test for differences between with and without mu-264 sic intervention, paired - samples for means t-test265 model was used. Statistical analysis impact of music266 to shorten the value of SL.267 Table 1: Accuracy of K-Means clustering algorithm Sample Total of epoch Accuracy (%) K_01_0204 121 100 K_02_2503 63 100 K_03_0304 81 93.83 K_04_2803 50 96.00 K_05_2603 102 99.17 K_06_2504 35 97.14 K_07_1804 32 93.75 C_01_0404 121 100 C_02_2703 60 98.41 C_03_0504 120 95.83 C_04_2903 126 95.24 C_05_2703 120 97.06 C_06_2804 100 94.00 C_07_1904 90 95.55 Figure 6 shows the sleep latency value of each subject 268 in two experiments: with andwithoutmusic interven- 269 tion. There are many different sleep latency values be- 270 cause each SL value depends on each person. To illus- 271 trate this, subject 1 reported in the survey that he did 272 not have a habit of napping, and music helped him 273 to fall asleep easier. Besides, subject 3 usually had a 274 break in the afternoon, for this reason, SL value was 275 not much different between the two experiments. In 276 sum, this research measures the change of sleep la- 277 tency by using their favorite music and shows the re- 278 sult that music can improve sleep in people who can- 279 not sleep in naptime. 280 Using ANOVA, the result: 281 • SL in normal experiment: mean = 9.0714 min, t 282 Stat = 3.0039, P (one-tail) = 1.9432, SD = 6.0853. 283 • SL in musical experiment: mean = 5.6423 min, t 284 Stat = 3.0039, P (one-tail) = 1.9432, SD = 3.4405. 285 It can clearly be seen that music helps to shorten SL 286 value of each subject, which makes people fall asleep 287 easier. But the SD value was high due to some reasons 288 below: 289 • The number of samples was limited. 290 • The SL value difference between subjects was 291 highly depended on the habit of having nap 292 sleep. 293 • The laboratory environment is not suitable for 294 some subjects owing to the enclosed space of 295 Faraday cage and noise. 296 5 Un co rr cti on pr oo f Science & Technology Development Journal – Engineering and Technology, 3(SI):xxx-xxx Figure 4: Sleep latency at the experiment without music intervention Figure 5: Sleep latency at the experiment with music intervention User interface297 To develop user interface by using GUIDE in MAT-298 LAB that helps the data were analyzed more conve-299 nient. Figure 7 shows the GUIDE developed inMAT-300 LAB with some main button to analyze data:301 - CHOOSE FILE: choose one data file in (*.txt) format302 - Options: Fs (Hz), Wavelet Name, From Detail – To303 Detail (analytical level based on NYQUIST rule)304 - Epoch (s): epoch duration and the program will cal-305 culate the total of epoch in that data file.306 - Epoch number: select the epoch to analysis and plot 307 the result. 308 - Slider: slip to the epoch number instead of typing 309 the epoch number. 310 - PLOT: the data were filtered and analyzed by using 311 Options. Then analyzed data were plotted on three 312 graphs to evaluate the results. 313 - Sleep latency: plot the power ratio of Theta and Al- 314 pha and export a dialog box of SL value as shown in 315 Figure 8. 316 6 Un co rre cti on pr oo f Science & Technology Development Journal – Engineering and Technology, 3(SI):xxx-xxx Figure 6: The sleep latency value of each subject in two experiments: with and without music intervention. Figure 7: User interface GUIDE 7 Un co rre cti on pr oo f Science & Technology Development Journal – Engineering and Technology, 3(SI):xxx-xxx Figure 8: Sleep latency in GUIDE CONCLUSION317 The present study had built an interface user to ana-318 lyze EEG signal using FFT, Wavelet, power ratio and319 K-means clustering algorithm. Instead of measur-320 ing SL by visualization or available self-reported ques-321 tionnaire, this study is empirical research that com-322 bines both the above methods. A new point of the323 research is Machine learning in analyzing data to cal-324 culate the SL value.325 The main aim of this study was to confirm that mu-326 sic affected to shorten the SL value – one factor in327 improving sleep quality, which helped the subject fall328 asleep easier and faster by using favoritemusic of each329 subject.330 However, there were some shortcomings that needed331 to be improved. The number of subjects and experi-332 ments was limited so that the result was not accurate.333 In the near future, this research can bemeasuredmore334 samples and limited the noise. Besides investigating335 each person’s response to certain types of music.336 ACKNOWLEDGMENT337 We acknowledge the support of time and facilities338 from Ho Chi Minh City University of Technology339 (HCMUT), VNU–HCM for this study.340 LIST OF ABBREVIATION341 AASM: American Academy of Sleep Medicine.342 EEG: Electroencephalogram.343 EMG: electromyogram.344 EOG: electrooculogram. 345 ESS: Epworth Sleepiness Scale. 346 FFT: Fast Fourier Transform. 347 MAR: Music – Assisted Relaxation. 348 PAI: Personality Assessment Inventory. 349 PSQI: Pittsburgh Sleep Quality Index. 350 SL: Sleep Latency. 351 AUTHOR S’ CONTRIBUTIONS 352 All authors contributed equally to this work. All au- 353 thors have read and agreed to the published version of 354 the manuscript. 355 CONFLICT OF INTEREST 356 We declare that there is no conflict of whatsoever in- 357 volved in publishing this research. 358 REFERENCES 359 1. Rosekind MR, et al. Alertness management: strategic naps 360 in operational settings. European Sleep Research Society. 361 1995;(4):62–66. PMID: 10607214. Available from: https://doi. 362 org/10.1111/j.1365-2869.1995.tb00229.x. 363 2. Chiung-Yu H, En-Ting C, Hui-Ling L. Use of integrative 364 medicine approaches for treating adults with sleep distur- 365 bances. Applied Nursing Research. 2018;43:49–55. PMID: 366 30220363. Available from: https://doi.org/10.1016/j.apnr.2018. 367 06.016. 368 3. Kira VJ, Julian K, Poul J, Peter V. Music for insomnia in 369 adults (Review). John Wiley & Sons, Ltd, Jylland. 2015;PMID: 370 26270746. Available from: https://doi.org/10.1002/14651858. 371 CD010459.pub2. 372 4. Gerrit N, Bea T, Bert L, Giel H. Music-assisted relaxation to im- 373 prove sleep quality: meta-analysis. Journal of AdvancedNurs- 374 ing. 2009;(65):1356–1364. PMID: 19456998. Available from: 375 https://doi.org/10.1111/j.1365-2648.2009.04982.x. 376 8 Un co rre cti n p roo f Science & Technology Development Journal – Engineering and Technology, 3(SI):xxx-xxx 5. Tan LP. The Effects of Background Music on Quality of Sleep377 in Elementary School Children. Journal of Music Therapy.378 2004;XLI(2):128–150. PMID: 15307813. Available from: https:379 //doi.org/10.1093/jmt/41.2.128.380 6. La’szlo’ H, Johanna T, Ro’bert B. Music improves sleep qual-381 ity in students. Journal of Advanced Nursing. 2008;(62):327–382 335. PMID: 18426457. Available from: https://doi.org/10.1111/383 j.1365-2648.2008.04602.x.384 7. Fan F, Yingshi Z, Jun H, Jiayi C, Qiyu J, Xiaojuan L, Qingchun Z,385 Bo-an L. Can Music Improve Sleep Quality in Adults with Pri-386 mary Insomnia? A Systematic Review and Network Primary387 Insomnia? A Systematic Review and Network Meta-analysis.388 International Journal of Nursing Studies. 2017;p. 15–20.389 8. Nantawachara J, Yodchanan W. A Novel Insight of Effects of a390 3-Hz Binaural Beat on Sleep Stages during Sleep. Frontiers in391 Human Neuroscience. 2018;p. 7–10. PMID: 30319382. Avail-392 able from: https://doi.org/10.3389/fnhum.2018.00387.393 9. Hui-Ling L, Marion G. Music improves sleep quality in older394 adults. Journal of Advanced Nursing. 2004;(49):241. PMID:395 15660547. Available from: https://doi.org/10.1111/j.1365-396 2648.2004.03281.x.397 10. Stanley EL, Robert DO. Lack of efficacy of music to improve398 sleep: A polysomnographic and quantitive EEG analysis. In-399 ternational Journal of Psychophysiology. 2007;(63):232–239.400 PMID: 17123654. Available from: https://doi.org/10.1016/j.401 ijpsycho.2006.10.004.402 11. Abhijeet DD, Avani AS, Seethalakshmi R, Ajita SN. Effect of403 Indian classical music on quality of sleep in depressed pa-404 tients: A randomized controlled trial. Nordic Journal of Music405 Therapy. 2014;18(1):70–78. Available from: https://doi.org/10.406 1080/08098130802697269.407 12. Weina Z, Junjun Z, Xiaojun D, Changle Z, Yuanye M, Dan X.408 Crossmodal effects of Guqin and piano music on selective409 attention: An event-related potential study. Neuroscience410 Letters. 2009;(446):21–26. PMID: 19766172. Available from:411 https://doi.org/10.1016/j.neulet.2009.09.026.412 13. Santostasi G. EEG Analysis on Brain.fm (Sleep). [Online] [Ac-413 cessed 1 December 2018]. 2015;Available from: https://brain.414 fm.415 14. Daniel JB, Charles FR, Timothy HM, Susan RB, and David416 JK. The Pittsburgh Sleep Quality Index: A New Instrument417 for Psychiatric Practice and Research. Psychiatry Research.418 1988;(28):193–213. Available from: https://doi.org/10.1016/419 0165-1781(89)90047-4.420 15. Conrad I, Ancoli-Israel S, Andrew LC Jr, Stuart FQ. The ASSM421 Manual for the Scoring of Sleep and Associated Events.422 Westchester: American Academy of Sleep Medicine. 2007;.423 16. NicoletOnevEEGSystem.NatusNeurology Incorporated,Mid-424 dleton, Wisconsin. 2014;.425 17. Marion G, Bradford LP, Safaa GS, Chi-Chun C, SandraFP, Defo-426 ria L. Cultural Differences inMusic Chosen for Pain Relief. Jour-427 nal of Holistic Nursing. 2000;18(3):245–260. PMID: 11847812.428 Available from: https://doi.org/10.1177/089801010001800306.429 18. Hanan AA, Faris AJ. Optimal Mother Wavelet Function for430 EEG Signal Analyze Based on Packet Wavelet Transform.431 International Journal of Scientific & Engineering Research.432 2017;8(2):1222–1227.433 19. John LS, BenjaminG. Biosignal andMedical Image Processing.434 Boca Raton: CRC Press. 2014;p. 540–548.435 9 Un co rr cti on pr oo f
File đính kèm:
eeg_based_study_on_sleep_quality_improvement_by_using_music.pdf